Open Access
ARTICLE
A Deep Learning Approach for Crowd Counting in Highly Congested Scene
1 Electrical Section, Universiti Kuala Lumpur British Malaysian Institute, Selangor, 53100, Malaysia
2 Department of Electrical Engineering, International Islamic University, Islamabad, 44000, Pakistan
3 Department of Information Technology, College of Computer, Qassim University, Buraydah, 51452, Saudi Arabia
4 Department of Electrical Engineering, National University of Computer and Emerging Sciences, Islamabad, 44000, Pakistan
5 Computer Engineering Technology Section, Universiti Kuala Lumpur Malaysian Institute of Information Technology, Kuala Lumpur, 50250, Malaysia
* Corresponding Author: Kushsairy Abdul Kadir. Email:
Computers, Materials & Continua 2022, 73(3), 5825-5844. https://doi.org/10.32604/cmc.2022.027077
Received 10 January 2022; Accepted 04 March 2022; Issue published 28 July 2022
Abstract
With the rapid progress of deep convolutional neural networks, several applications of crowd counting have been proposed and explored in the literature. In congested scene monitoring, a variety of crowd density estimating approaches has been developed. The understanding of highly congested scenes for crowd counting during Muslim gatherings of Hajj and Umrah is a challenging task, as a large number of individuals stand nearby and, it is hard for detection techniques to recognize them, as the crowd can vary from low density to high density. To deal with such highly congested scenes, we have proposed the Congested Scene Crowd Counting Network (CSCC-Net) using VGG-16 as a core network with its first ten layers due to its strong and robust transfer learning rate. A hole dilated convolutional neural network is used at the back end to widen the relevant field to extract a large range of information from the image without losing its original resolution. The dilated convolution neural network is mainly chosen to expand the kernel size without changing other parameters. Moreover, several loss functions have been applied to strengthen the evaluation accuracy of the model. Finally, the entire experiments have been evaluated using prominent data sets namely, ShanghaiTech parts A, B, UCF_CC_50, and UCF_QNRF. Our model has achieved remarkable results i.e., 68.0 and 9.0 MAE on ShanghaiTech parts A, B, 199.1 MAE on UCF_CC_50, and 99.8 on UCF_QNRF data sets respectively.Keywords
Cite This Article
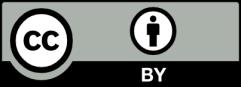