Open Access
ARTICLE
Deep Learning Prediction Model for NiCrAlY Diffusion Barrier Thickness for Tungsten Wires
Department of Computer Sciences, College of Computer and Information Sciences, Princess Nourah bint Abdulrahman University, P.O. Box 84428, Riyadh, 11671, Saudi Arabia
* Corresponding Author: Hanan A. Hosni Mahmoud. Email:
Computers, Materials & Continua 2022, 73(3), 5755-5769. https://doi.org/10.32604/cmc.2022.032212
Received 10 May 2022; Accepted 12 June 2022; Issue published 28 July 2022
Abstract
In the last decades, technology has used Copper for IC interconnect and it has been the best material used in the wire downsizing. However, Copper is now showing inefficiency as downscaling is getting deeper. Recent research starts to show Tungsten (W) as a possible replacement, for its better downsizing characteristic. The scaling-down of interconnects dimension has to be augmented with thin diffusion layers. It is crucial to subdue tungsten diffusion in the nickel-based thermal spray Flexicord (NiCrAlY) coating layers. Inappropriately, diffusion barriers with thicknesses less than 4.3 nm do not to execute well. With the introduction of two dimensional layers, hexagonal boron has been recommended as a substitute for Tungsten diffusion barrier layers with thicknesses less than 1.5 Nano meters (nm). Nevertheless, vacancies flaws may develop into a Tungsten dissemination path, which is a problematic issue in the manufacturing of diffusion barriers. The energy layer density, of Tungsten atom diffusion via a di-vacancy in NiCrAlY, is computed by density functions 3D. NiCrAlY has complex energy barrier which is thicker than other materials such as Graphene. This is due to the sturdier contact and charge variance of NI and Cr in NiCrAlY. Also, we utilize the energy barriers of several vacancy constructions and produce a dataset to be employed in the proposed 3-imensional deep learning model (3D-DNN). Our trained deep learning neural model can predict the energy barrier of Tungsten diffusion through arbitrarily configured NiCrAlY with accuracy greater than 98.4% in 5 × 5 cell. Prediction results generate directors on selecting barriers through energy computation.Keywords
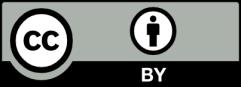