Open Access
ARTICLE
Crime Prediction Methods Based on Machine Learning: A Survey
School of Social Science, University of Manchester, M13 9PL Oxford Road, Manchester, UK
* Corresponding Author: Junxiang Yin. Email:
Computers, Materials & Continua 2023, 74(2), 4601-4629. https://doi.org/10.32604/cmc.2023.034190
Received 08 July 2022; Accepted 03 October 2022; Issue published 31 October 2022
Abstract
The objective of crime prediction, one of the most important technologies in social computing, is to extract useful information from many existing criminal records to predict the next process-related crime. It can aid the police in obtaining criminal information and warn the public to be vigilant in certain areas. With the rapid growth of big data, the Internet of Things, and other technologies, as well as the increasing use of artificial intelligence in forecasting models, crime prediction models based on deep learning techniques are accelerating. Therefore, it is necessary to classify the existing crime prediction algorithms and compare in depth the attributes and conditions that play an essential role in the analysis of crime prediction algorithms. Existing crime prediction methods can be roughly divided into two categories: those based on conventional machine learning and those based on contemporary deep learning. This survey analyses the fundamental theories and procedures. The most frequently used data sets are then enumerated, and the fundamental procedures of various algorithms are also analyzed in this paper. In light of the insufficient scale of existing data in this field, the ambiguity of data types used to predict crimes, and the absence of public data sets that have a significant impact on the research of algorithm models, this survey proposes the construction of a machine learning-based big data research model to address these issues. Future researchers who will enter this field are provided with a guide to the direction of future research development.Keywords
Cite This Article
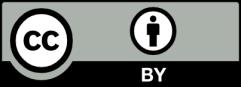