Open Access
ARTICLE
Chaotic Flower Pollination with Deep Learning Based COVID-19 Classification Model
1 Department of Information Technology, Manipal Institute of Technology Bengaluru, Manipal Academy of Higher Education, Manipal, India
2 Department of Medical Equipment Technology, College of Applied Medical Sciences, Majmaah University, Al Majmaah, 11952, Saudi Arabia
3 Public Health Department, College of Applied Medical Sciences, Majmaah University, Al Majmaah, 11952, Saudi Arabia
4 Department of Computing Technologies, College of Engineering and Technology, Faculty of Engineering and Technology, SRM Institute of Science and Technology, Kattankulathur, 603203, Tamilnadu, India
5 Medical Instrumentation Techniques Engineering Department, Al-Mustaqbal University College, Babylon, Iraq
6 College of Technical Engineering, The Islamic University, Najaf, Iraq
7 College of Information Technology, Imam Ja’afar Al-Sadiq University, Al-Muthanna, 66002, Iraq
* Corresponding Author: Ahmed Alkhayyat. Email:
Computers, Materials & Continua 2023, 74(3), 6195-6212. https://doi.org/10.32604/cmc.2023.033252
Received 12 June 2022; Accepted 12 October 2022; Issue published 28 December 2022
Abstract
The Coronavirus Disease (COVID-19) pandemic has exposed the vulnerabilities of medical services across the globe, especially in underdeveloped nations. In the aftermath of the COVID-19 outbreak, a strong demand exists for developing novel computer-assisted diagnostic tools to execute rapid and cost-effective screenings in locations where many screenings cannot be executed using conventional methods. Medical imaging has become a crucial component in the disease diagnosis process, whereas X-rays and Computed Tomography (CT) scan imaging are employed in a deep network to diagnose the diseases. In general, four steps are followed in image-based diagnostics and disease classification processes by making use of the neural networks, such as network training, feature extraction, model performance testing and optimal feature selection. The current research article devises a Chaotic Flower Pollination Algorithm with a Deep Learning-Driven Fusion (CFPA-DLDF) approach for detecting and classifying COVID-19. The presented CFPA-DLDF model is developed by integrating two DL models to recognize COVID-19 in medical images. Initially, the proposed CFPA-DLDF technique employs the Gabor Filtering (GF) approach to pre-process the input images. In addition, a weighted voting-based ensemble model is employed for feature extraction, in which both VGG-19 and the MixNet models are included. Finally, the CFPA with Recurrent Neural Network (RNN) model is utilized for classification, showing the work’s novelty. A comparative analysis was conducted to demonstrate the enhanced performance of the proposed CFPA-DLDF model, and the results established the supremacy of the proposed CFPA-DLDF model over recent approaches.Keywords
Cite This Article
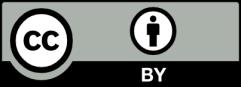