Open Access
ARTICLE
Explainable Anomaly Detection Using Vision Transformer Based SVDD
1 Department of Computer Science, Kyonggi University, Suwon-si 16227, Korea
2 Division of AI Computer Science and Engineering, Kyonggi University, Suwon-si 16227, Korea
* Corresponding Author: Kyungyong Chung. Email:
Computers, Materials & Continua 2023, 74(3), 6573-6586. https://doi.org/10.32604/cmc.2023.035246
Received 13 August 2022; Accepted 01 November 2022; Issue published 28 December 2022
Abstract
Explainable AI extracts a variety of patterns of data in the learning process and draws hidden information through the discovery of semantic relationships. It is possible to offer the explainable basis of decision-making for inference results. Through the causality of risk factors that have an ambiguous association in big medical data, it is possible to increase transparency and reliability of explainable decision-making that helps to diagnose disease status. In addition, the technique makes it possible to accurately predict disease risk for anomaly detection. Vision transformer for anomaly detection from image data makes classification through MLP. Unfortunately, in MLP, a vector value depends on patch sequence information, and thus a weight changes. This should solve the problem that there is a difference in the result value according to the change in the weight. In addition, since the deep learning model is a black box model, there is a problem that it is difficult to interpret the results determined by the model. Therefore, there is a need for an explainable method for the part where the disease exists. To solve the problem, this study proposes explainable anomaly detection using vision transformer-based Deep Support Vector Data Description (SVDD). The proposed method applies the SVDD to solve the problem of MLP in which a result value is different depending on a weight change that is influenced by patch sequence information used in the vision transformer. In order to draw the explain-ability of model results, it visualizes normal parts through Grad-CAM. In health data, both medical staff and patients are able to identify abnormal parts easily. In addition, it is possible to improve the reliability of models and medical staff. For performance evaluation normal/abnormal classification accuracy and f-measure are evaluated, according to whether to apply SVDD. Evaluation Results The results of classification by applying the proposed SVDD are evaluated excellently. Therefore, through the proposed method, it is possible to improve the reliability of decision-making by identifying the location of the disease and deriving consistent results.Keywords
Cite This Article
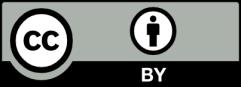
This work is licensed under a Creative Commons Attribution 4.0 International License , which permits unrestricted use, distribution, and reproduction in any medium, provided the original work is properly cited.