Open Access
ARTICLE
License Plate Recognition via Attention Mechanism
1 School of Computer Science and Cyberspace Security Hainan University, Haikou, 570228, China
2 Key Laboratory of Internet Information Retrieval of Hainan Province, Haikou, 570228, China
3 Hilbert College, Hamburg, NY, 14075, USA
* Corresponding Author: Jun Ye. Email:
Computers, Materials & Continua 2023, 75(1), 1801-1814. https://doi.org/10.32604/cmc.2023.032785
Received 29 May 2022; Accepted 26 August 2022; Issue published 06 February 2023
Abstract
License plate recognition technology use widely in intelligent traffic management and control. Researchers have been committed to improving the speed and accuracy of license plate recognition for nearly 30 years. This paper is the first to propose combining the attention mechanism with YOLO-v5 and LPRnet to construct a new license plate recognition model (LPR-CBAM-Net). Through the attention mechanism CBAM (Convolutional Block Attention Module), the importance of different feature channels in license plate recognition can be re-calibrated to obtain proper attention to features. Force information to achieve the purpose of improving recognition speed and accuracy. Experimental results show that the model construction method is superior in speed and accuracy to traditional license plate recognition algorithms. The accuracy of the recognition model of the CBAM model is increased by two percentage points to 97.2%, and the size of the constructed model is only 1.8 M, which can meet the requirements of real-time execution of embedded low-power devices. The codes for training and evaluating LPR-CBAM-Net are available under the open-source MIT License at: .Keywords
Cite This Article
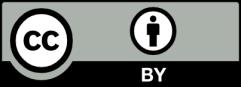