Open Access
ARTICLE
Fruit Leaf Diseases Classification: A Hierarchical Deep Learning Framework
1 Department of Computer Science, HITEC University, Taxila, 47080, Pakistan
2 Computer Sciences Department, College of Computer and Information Sciences, Princess Nourah bint Abdulrahman University, Riyadh, 11671, Saudi Arabia
3 Department of Electrical Engineering, College of Engineering, Jouf University, Sakakah, 72311, Saudi Arabia
4 College of Computer Engineering and Sciences, Prince Sattam bin Abdulaziz University, Al-Kharj, 16242, Saudi Arabia
5 Department of ICT Convergence, Soonchunhyang University, Asan, 31538, Korea
* Corresponding Author: Yunyoung Nam. Email:
Computers, Materials & Continua 2023, 75(1), 1179-1194. https://doi.org/10.32604/cmc.2023.035324
Received 16 August 2022; Accepted 14 December 2022; Issue published 06 February 2023
Abstract
Manual inspection of fruit diseases is a time-consuming and costly because it is based on naked-eye observation. The authors present computer vision techniques for detecting and classifying fruit leaf diseases. Examples of computer vision techniques are preprocessing original images for visualization of infected regions, feature extraction from raw or segmented images, feature fusion, feature selection, and classification. The following are the major challenges identified by researchers in the literature: (i) low-contrast infected regions extract irrelevant and redundant information, which misleads classification accuracy; (ii) irrelevant and redundant information may increase computational time and reduce the designed model’s accuracy. This paper proposed a framework for fruit leaf disease classification based on deep hierarchical learning and best feature selection. In the proposed framework, contrast is first improved using a hybrid approach, and then data augmentation is used to solve the problem of an imbalanced dataset. The next step is to use a pre-trained deep model named Darknet53 and fine-tune it. Next, deep transfer learning-based training is carried out, and features are extracted using an activation function on the average pooling layer. Finally, an improved butterfly optimization algorithm is proposed, which selects the best features for classification using machine learning classifiers. The experiment was carried out on augmented and original fruit datasets, yielding a maximum accuracy of 99.6% for apple diseases, 99.6% for grapes, 99.9% for peach diseases, and 100% for cherry diseases. The overall average achieved accuracy is 99.7%, higher than previous techniques.Keywords
Cite This Article
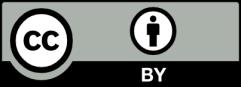