Open Access
ARTICLE
Prediction of Uncertainty Estimation and Confidence Calibration Using Fully Convolutional Neural Network
1 Department of Computer Science, College of Arts and Sciences at Tabarjal, Jouf University, Jouf, Saudi Arabia
2 College of Sciences and Humanities, Prince Sattam Bin Abdulaziz University, Al-Kharj, Saudi Arabia
3 University of Sfax, Sfax, Tunisia
4 Department of Information Systems, College of Computer and Information Sciences, Jouf University, Jouf, Saudi Arabia
* Corresponding Author: Karim Gasmi. Email:
Computers, Materials & Continua 2023, 75(2), 2557-2573. https://doi.org/10.32604/cmc.2023.033270
Received 12 June 2022; Accepted 16 September 2022; Issue published 31 March 2023
Abstract
Convolution neural networks (CNNs) have proven to be effective clinical imaging methods. This study highlighted some of the key issues within these systems. It is difficult to train these systems in a limited clinical image databases, and many publications present strategies including such learning algorithm. Furthermore, these patterns are known for making a highly reliable prognosis. In addition, normalization of volume and losses of dice have been used effectively to accelerate and stabilize the training. Furthermore, these systems are improperly regulated, resulting in more confident ratings for correct and incorrect classification, which are inaccurate and difficult to understand. This study examines the risk assessment of Fully Convolutional Neural Networks (FCNNs) for clinical image segmentation. Essential contributions have been made to this planned work: 1) dice loss and cross-entropy loss are compared on the basis of segment quality and uncertain assessment of FCNNs; 2) proposal for a group model for assurance measurement of full convolutional neural networks trained with dice loss and group normalization; And 3) the ability of the measured FCNs to evaluate the segment quality of the structures and to identify test examples outside the distribution. To evaluate the study’s contributions, it conducted a series of tests in three clinical image division applications such as heart, brain and prostate. The findings of the study provide significant insights into the predictive ambiguity assessment and a practical strategies for outside-distribution identification and reliable measurement in the clinical image segmentation. The approaches presented in this research significantly enhance the reliability and accuracy rating of CNN-based clinical imaging methods.Keywords
Cite This Article
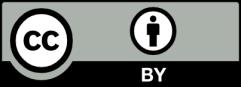