Open Access
ARTICLE
Computational Investigation of Hand Foot Mouth Disease Dynamics with Fuzziness
1 Department of Mathematics, Cankaya University, Balgat, Ankara, 06530, Turkey
2 Department of Medical Research, China Medical University, Taichung, 406040, Taiwan
3 Department of Computer Science and Mathematics, Lebanese American University, Beirut, Lebanon
4 Department of Mathematics, School of Science, University of Management and Technology, Lahore, 54000, Pakistan
5 Department of Mathematics and Statistics, University of Lahore, Lahore, 54590, Pakistan
6 Department of Mathematics, Faculty of Science and Technology, University of Central Punjab, Lahore, 54000, Pakistan
7 Near East University, Mathematics Research Center, Department of Mathematics, Near East Boulevard, 99138, Nicosia/Mersin 10, Turkey
8 Department of Mathematics, Govt. Maulana Zafar Ali Khan Graduate College Wazirabad, Punjab Higher Education Department (PHED), Lahore, 54000, Pakistan
* Corresponding Author: Nauman Ahmed. Email:
Computers, Materials & Continua 2023, 75(2), 4175-4189. https://doi.org/10.32604/cmc.2023.034868
Received 30 June 2022; Accepted 26 January 2023; Issue published 31 March 2023
Abstract
The first major outbreak of the severely complicated hand, foot and mouth disease (HFMD), primarily caused by enterovirus 71, was reported in Taiwan in 1998. HFMD surveillance is needed to assess the spread of HFMD. The parameters we use in mathematical models are usually classical mathematical parameters, called crisp parameters, which are taken for granted. But any biological or physical phenomenon is best explained by uncertainty. To represent a realistic situation in any mathematical model, fuzzy parameters can be very useful. Many articles have been published on how to control and prevent HFMD from the perspective of public health and statistical modeling. However, few works use fuzzy theory in building models to simulate HFMD dynamics. In this context, we examined an HFMD model with fuzzy parameters. A Non Standard Finite Difference (NSFD) scheme is developed to solve the model. The developed technique retains essential properties such as positivity and dynamic consistency. Numerical simulations are presented to support the analytical results. The convergence and consistency of the proposed method are also discussed. The proposed method converges unconditionally while the many classical methods in the literature do not possess this property. In this regard, our proposed method can be considered as a reliable tool for studying the dynamics of HFMD.Keywords
Although it can affect adults as well, HFMD is a viral illness that is only mildly contagious and often affects young children. The two viruses most frequently responsible for the spread of HFMD are coxsackievirusesA16 and enteroviruses71 [1,2]. The main symptoms of HFMD are fever, vomiting, headache, malaise, lethargy, poor appetite, and a sore throat, but many infected persons don’t have any symptoms. Another sign of HFMD is sores in the mouth or on the lips, as well as on the hands, feet, and occasionally the buttocks and legs.
After being discovered in New Zealand in 1957, HFMD has rapidly gained popularity throughout the world. It does not cause a particularly serious illness and is only moderately contagious. Recent HFMD outbreaks in China, Taiwan, Singapore, Sarawak, Malaysia, etc. have spread awareness of the disease through fatalities across the globe [3]. The HFMD virus can spread through coughing, sneezing, and infected feces. For HFMD, there is no specific treatment. Specialists recommend different medications for different symptoms, such as pain and fever that can be treated with medication. Since there is no effective therapeutic treatment against HFMD, it should be controlled by appropriate preventative measures such as isolation, personal protection against contact with infected people, etc. In general, HFMD is not a serious condition in the infected population. Only a very small minority of those infected require hospitalization, mainly due to neurological complications (encephalitis, meningitis or acute flaccid paralysis) or pulmonary edema/pulmonary hemorrhage. There is no lifelong immunity to HFMD because the disease is caused by a group of viruses very similar to influenza.
In addition to medical issues, HFMD has tremendous social and financial repercussions on countries. Understanding the spread of HFMD among susceptible populations is therefore very important that will help policymakers to maximize the effectiveness of treatment resources. The subject hasn’t been explored all that much, and there isn’t much literature on it that deals with mathematical and numerical modeling. Roy et al. developed an SEIR model in order to better understand the dynamics of HFMD and concluded that the transmission of HFMD depends more on the number of actively infected individuals in the population at the start and also on the rate of disease transmission at any given time [4].
Every community experiences changes as the climate changes. Similar to this, the parameters used in mathematical modeling are dynamic and cannot be fixed [5]. Global warming is one of the key causes of the rise in the earth’s average temperature. The varying temperatures also have an impact on how quickly the virus spreads throughout society. In an effort to forecast the relationship of HFMD with weather patterns, Urashima et al. explored the consequences of global warming [6]. By taking into account quarantine in the population of children, Liu provided a periodic model for the simulation of the dynamics of HFMD transmission [7]. Samanta investigated the discrete delay, non-uniform population size, and saturation incidence rate in the delayed HFMD model. The model of a pulsed vaccination has also been studied [8,9]. Hii et al. investigated Singapore’s HFMD incidence risk in relation to weather variables [10].
Parameters used in existing HFMD epidemic models use crisp numbers, while parameter uncertainty and population heterogeneity are very likely to occur. In order to make the model more realistic, the use of fuzzy parameters in these models is very important. Many researchers have applied fuzzy theory to study disease transmission. Barros et al. developed an epidemic model with fuzzy transmission coefficients [11]. A comparison of the average change in viral load and the average number of people infected was done to analyze the reproduction number. Ortega et al. studied a rabies model in fuzzy senses [12]. Verma et al. developed a Susceptible, Exposed, Infected and Recovered (SEIR) model with fuzzy criteria [13]. A Susceptible, Infected and Recovered (SIR) model was presented by Das et al. with imprecise parameters [14]. A model of food chain in the fuzzy environment with optimal harvesting was studied by Sadhukhan et al. [15]. Mishra et al. presented a fuzzy Susceptible, Exposed, Infected, Quarantine Recovered and Susceptible (SEIQRS) computer virus model [16]. Mangongo et al. introduced fuzzy global stability [17]. A fuzzy Susceptible, Exposed, Infected, Quarantine and Recovered (SEIQR) model was described by Allehiany et al. [18]. The model was solved using the NSFD technique in fuzzy senses, a development of Micken’s theory [19]. Dayan et al. proposed a SIR model in a fuzzy environment [20]. Euler, Runge Kutta of order 4 (RK-4) and the NSFD methods were developed with fuzzy extensions for the solution of the model. Dayan et al. also presented rumor based fuzzy model and developed an NSFD scheme for its solution [21]. Fractal fractional operators are used for the numerical solution of a tumour-immune model by Ahmad et al. [22]. Many researchers studied the NSFD schemes in stochastic senses. Arif et al. studied a stochastic SIR epidemic model [23]. A stochastic model for the numerical investigation of the computer virus was presented by Shatanawi et al. [24]. A stochastic Dengue model using NSFD schemes was proposed by Noor et al. [25]. Shoaib Arif et al. presented a stochastic model of COVID-19 and studied it using NSFD scheme [26]. Researchers studied fractional stochastic models, for example [27], for example. Naveed et al. studied a COVID-19 model with delay effect using NSFD theory [28]. Shatanawi et al. proposed a corona virus model in stochastic senses and developed numerical schemes for its solutions [29]. Baleanu et al. presented a fractional chaotic system and NSFD scheme is used to study the chaotic behavior of the model [30]. Nawaz et al. proposed a fractional order diffusive epidemic model to study the COVID-19 [31].
The existing HFMD models employing crisp numbers are insufficient in order to construct the fuzzy numerical and mathematical techniques. In this context, we examined a SEIR model with fuzzy parameters. The terms susceptible, exposed, infected and recovered are uncertain due to differences in susceptibility, exposure, infectivity and recovery among individuals in the population. Due to the different characteristics of these parameters in the population, uncertainties may arise. Different ages of the population considered may have different customs, habits, resistances, etc. because of their different origins. In many real situations, collecting numerical data as a fixed value is quite difficult, while the range of the data can be easily decided. Models are needed to deal with the above uncertainties for these different levels of individuals. The fuzzy theory facilitates us in resolving the troubles of quantifying uncertainty in mathematical modeling. In this context, mathematical models with fuzziness are more meaningful and perform better. With this in mind, we have extended a classic SEIR model by introducing fuzziness into the model. HFMD transmission, recovery and human mortality rates due to disease are considered fuzzy numbers because these parameters are direct functions of HFMD. In the case of a classical system, these parameters are not direct functions of the disease. Therefore, the fuzzy model can be considered more balanced and flexible. Thus, the use of fuzzy parameters helps us to explain HFMD transmission in more detail.
2 HFMD Model with Fuzzy Parameters
Consider the following system of 4 first order ordinary differential equations representing the SEIR model of HFMD dynamics proposed by Putri et al. [32].
The corresponding fuzzy model can be written as
The HFMD transmission, recovery from HFMD and the mortality rates of the infected individuals are considered fuzzy numbers due to their uncertain natures. These parameters are denoted by
and
The death rate
2.1 The Fuzzy Basic Reproductive Number (BRN)
The BRN
Since
Case 1: If
Case 2: If
Case 3: If
The fuzzy reproduction number can be found as follows [17]:
2.2 Fuzzy Equilibrium Analysis
Case 1:
Case 2: If
Case 3: If
3.1 Non-Standard Finite Difference (NSFD) Scheme
NSFD scheme for the system (5–8) is
In this segment, the convergence of the developed NSFD scheme at DFE point
The system (13–16) can be written as:
The Jacobian matrix corresponding to the system (17–20) is
The above Jacobian matrix becomes
The above Jacobian matrix at the Disease Free Equilibrium (DFE)
The proposed NSFD scheme will be unconditionally convergent iff
Here,
To check the consistency of the proposed scheme, we apply the Taylor’s series. From Eq. (13),
For the consistency, following procedure is adopted, considering the Taylor’s series expansion
Substituting the value of
Taking
Or
From Eq. (14),
The Taylor’s series expansion of the compartment E is
Substituting the value of
Taking
Similarly, we can get
and
by applying Taylor’s series on Eqs. (13–16). It is therefore concluded that our proposed scheme is consistent of order 1.
Dynamics of the subpopulations are shown in Figs. 1 and 2 for DFE at
Figure 1: Dynamics of subpopulations at DFE at
Figure 2: Dynamics of subpopulations at DFE at
Fig. 3 shows the solutions of the exposed and infected compartments respectively at the first EE point, i.e., case 2 at a small step size
Figure 3: Dynamics of exposed and infected populations at first EE at
Figure 4: Dynamics of exposed and infected populations at first EE at
Dynamics of the exposed and infected populations for case 3 are shown in Figs. 5 and 6 respectively. An increase in both compartments can be seen with the increase in the value of the transmission rate of the disease. The consistency, convergence and positive solutions at both smaller and large step sizes can also be observed which are the most important features of such kinds of models. Many standard schemes fail to preserve these characteristics. From the above graphs, it can be concluded that the method developed in this work can be considered a more reliable strategy for investigating the disease of FHMD in the human population at EE.
Figure 5: Dynamics of subpopulations at second EE at
Figure 6: Dynamics of subpopulations at second EE at
A comparison of the exposed and infected compartments of the studied model at DFE and EEs is shown in Figs. 7 and 8 respectively. The proposed method gives convergent solutions at both small and a large step values of
Figure 7: Comparison of subpopulations at DFE and EE at
Figure 8: Comparison of subpopulations at DFE and EE at
A model of HFMD with fuzzy parameters is studied in this work. In general, due to the natural immune power of mankind, with a small amount of viruses, a disease may not be effective. The system will be endemic if the virus quantity is higher. Therefore, for a small amount of virus, heavy treatment is not necessary. This phenomenon can only be observed in the fuzzy model, and the crisp model does not have the ability to sustain it. Therefore, fuzzy models are more flexible than corresponding classical models. Further, while considering the problems associated with human health in the world, more reliable models are needed and the fuzzy models are quite capable of this. The parameters
Acknowledgement: Thanks to our families and colleagues who supported us morally.
Funding Statement: The authors received no specific funding for this study.
Conflicts of Interest: The authors declare that they have no conflicts of interest to report regarding the present study.
References
1. J. Y. Yang, Y. Chen and F. Q. Zhang, “Stability analysis and optimal control of a hand–foot–mouth disease (HFMD) model,” Journal of Applied Mathematics and Computing, vol. 41, no. 1, pp. 99–117, 2013. [Google Scholar]
2. Q. Zhu, Y. T. Hao, J. Q. Ma, S. C. Yu and Y. Wang, “Surveillance of hand, foot and mouth disease in mainland China (2008–2009),” Biomedical and Environmental Sciences, vol. 24, no. 4, pp. 349–356, 2011. [Google Scholar] [PubMed]
3. S. Sharma and G. Samanta, “Analysis of a hand foot mouth disease model,” International Journal of Biomathematics, vol. 10, no. 2, pp. 1–47, 2017. [Google Scholar]
4. N. Roy and N. Halder, “Compartmental modeling of hand, foot and mouth disease (HFMD),” Research Journal of Applied Sciences, vol. 5, no. 3, pp. 177–182, 2010. [Google Scholar]
5. P. Panja, S. K. Mondal and J. Chattopadhyay, “Dynamical study in fuzzy threshold dynamics of a cholera epidemic model,” Fuzzy Information and Engineering, vol. 9, no. 3, pp. 381–401, 2017. [Google Scholar]
6. M. Urashima, N. Shindo and N. Okabe, “Seasonal models of herpangina and hand–foot–mouth disease to simulate annual fluctuations in urban warming in Tokyo,” Japanese Journal of Infectious Diseases, vol. 56, no. 2, pp. 48–53, 2003. [Google Scholar] [PubMed]
7. J. Liu, “Threshold dynamics for a HFMD epidemic model with periodic transmission rate,” Nonlinear Dynamics, vol. 64, no. 1, pp. 89–95, 2011. [Google Scholar]
8. G. P. Samanta, “Analysis of a delayed hand–foot–mouth disease epidemic model with pulse vaccination,” Systems Science & Control Engineering: An Open Access Journal, vol. 2, no. 1, pp. 61–73, 2014. [Google Scholar]
9. G. P. Samanta, “A delayed hand–foot–mouth disease model with pulse vaccination strategy,” Computational and Applied Mathematics, vol. 34, no. 3, pp. 1131–1152, 2015. [Google Scholar]
10. Y. L. Hii, J. Rocklov and N. Ng, “Short-term effects of weather on hand, foot and mouth disease,” PLoS One, vol. 6, no. 2, pp. 99–105, 2011. [Google Scholar]
11. L. C. Barros, M. B. Ferreira Leite and R. C. Bassanezi, “The SI epidemiological models with a fuzzy transmission parameter,” Computers & Mathematics with Applications, vol. 45, no. 10, pp. 1619–1628, 2003. [Google Scholar]
12. N. R. S. Ortega, P. C. Sallum and E. Massad, “Fuzzy dynamical systems in epidemic modeling,” Kybernetes, vol. 29, no. 2, pp. 201–218, 2000. [Google Scholar]
13. R. Verma, S. P. Tiwari and R. K. Upadhyay, “Transmission dynamics of epidemic spread and outbreak of Ebola in West Africa: Fuzzy modeling and simulation,” Journal of Applied Mathematics and Computing, vol. 60, no. 1, pp. 637–671, 2019. [Google Scholar]
14. A. Das and M. Pal, “A mathematical study of an imprecise SIR epidemic model with treatment control,” Journal of Applied Mathematics and Computing, vol. 56, no. 1, pp. 477–500, 2018. [Google Scholar]
15. D. Sadhukhan, L. N. Sahoo, B. Mondal and M. Maitri, “Food chain model with optimal harvesting in fuzzy environment,” Journal of Applied Mathematics and Computing, vol. 34, no. 1, pp. 1–18, 2010. [Google Scholar]
16. B. Mishra and A. Prajapati, “Spread of malicious objects in computer network: A fuzzy approach,” Applications and Applied Mathematics: An International Journal, vol. 8, no. 2, pp. 684–700, 2013. [Google Scholar]
17. Y. Mangongo, J. Bukweli and J. Kampempe, “Fuzzy global stability analysis of the dynamics of malaria with fuzzy transmission and recovery rates,” American Journal of Operations Research, vol. 11, no. 6, pp. 257–282, 2021. [Google Scholar]
18. F. M. Allehiany, F. Dayan, F. F. Al-Harbi, N. Althobaiti, N. Ahmed et al., “Bio-inspired numerical analysis of COVID-19 with fuzzy parameters,” Computer, Materials & Continua, vol. 72, no. 2, pp. 3213–3229, 2022. [Google Scholar]
19. R. E. Mickens, “A fundamental principle for constructing non-standard finite difference schemes for differential equations,” Journal of Difference Equations and Applications, vol. 11, no. 2, pp. 645–653, 2005. [Google Scholar]
20. F. Dayan, N. Ahmed, M. Rafiq, A. Akgül, A. Raza et al., “Construction and numerical analysis of a fuzzy non-standard computational method for the solution of an SEIQR model of COVID-19 dynamics,” AIMS Mathematics, vol. 7, no. 5, pp. 8449–8470, 2022. [Google Scholar]
21. F. Dayan, M. Rafiq, N. Ahmed, D. Baleanu, A. Raza et al., “Design and numerical analysis of fuzzy nonstandard computational methods for the solution of rumor based fuzzy epidemic model,” Physica A: Statistical Mechanics and Its Applications, vol. 2022. https://doi.org/10.1016/j.physa.2022.127542 [Google Scholar] [CrossRef]
22. S. Ahmad, A. Ullah, A. Akgül and D. Baleanu, “Theoretical and numerical analysis of fractal fractional model of tumor-immune interaction with two different kernels,” Alexandria Engineering Journal, vol. 61, no. 7, pp. 5735–5752, 2022. [Google Scholar]
23. M. S. Arif, A. Raza, W. Shatanawi, M. Rafiq and M. Bibi, “A stochastic numerical analysis for computer virus model with vertical transmission over the internet,” Computers, Materials & Continua, vol. 61, no. 3, pp. 1025–1043, 2019. [Google Scholar]
24. W. Shatanawi, A. Raza, M. S. Arif, M. Rafiq, M. Bibi et al., “Essential features preserving dynamics of stochastic Dengue model,” Computer Modeling in Engineering & Sciences, vol. 126, no. 1, pp. 201–215, 2021. [Google Scholar]
25. M. A. Noor, A. Raza, M. S. Arif, M. Rafiq, K. S. Nisar et al., “Non-standard computational analysis of the stochastic COVID-19 pandemic model: An application of computational biology,” Alexandria Engineering Journal, vol. 61, no. 1, pp. 619–630, 2022. [Google Scholar]
26. M. Shoaib Arif, A. Raza, K. Abodayeh, M. Rafiq and A. Nazeer, “A numerical efficient technique for the solution of susceptible infected recovered epidemic model,” Computer Modeling in Engineering & Sciences, vol. 124, no. 2, pp. 477–491, 2020. [Google Scholar]
27. M. Shoaib Arif, A. Raza, M. Rafiq, M. Bibi, R. Fayyaz et al., “A reliable stochastic numerical analysis for typhoid fever incorporating with protection against infection,” Computers, Materials & Continua, vol. 59, no. 3, pp. 787–804, 2019. [Google Scholar]
28. M. Naveed, M. Rafiq, A. Raza, N. Ahmed, I. Khan et al., “Mathematical analysis of novel coronavirus (2019-nCov) delay pandemic model,” Computers, Materials & Continua, vol. 64, no. 3, pp. 1401–1414, 2020. [Google Scholar]
29. W. Shatanawi, A. Raza, M. S. Arif, K. Abodayeh, M. Rafiq et al., “An effective numerical method for the solution of a stochastic coronavirus (2019-ncovid) pandemic model,” Computers, Materials & Continua, vol. 66, no. 2, pp. 1121–1137, 2021. [Google Scholar]
30. D. Baleanu, S. Zibaei, M. Namjoo and A. Jajarmi, “A nonstandard finite difference scheme for the modeling and nonidentical synchronization of a novel fractional chaotic system,” Advances in Difference Equations, vol. 2021, no. 1, pp. 1–19, 2021. [Google Scholar]
31. Y. Nawaz, M. S. Arif, W. Shatanawi and M. U. Ashraf, “A new unconditionally stable implicit numerical scheme for fractional diffusive epidemic model,” AIMS Mathematics, vol. 7, no. 8, pp. 14299–14322, 2022. [Google Scholar]
32. A. Y. Putri, D. Handayani and R. Saragih, “The dynamic of hand-foot-mouth disease model using Takagi-Sugeno fuzzy system,” in AIP Conference Proceedings, vol. 2264, no. 1, pp. 1–10, 2020. [Google Scholar]
33. A. Raza, M. S. Arif, M. Rafiq, M. Bibi, M. Naveed et al., “Numerical treatment for stochastic computer virus,” Computer Modeling in Engineering & Sciences, vol. 120, no. 2, pp. 445–465, 2019. [Google Scholar]
34. M. Rafiq, A. Ahmadian, A. Raza, D. Baleanu, M. S. Ahsan et al., “Numerical control measures of stochastic malaria epidemic model,” Computers, Materials & Continua, vol. 65, no. 1, pp. 33–51, 2020. [Google Scholar]
Cite This Article
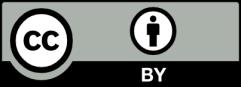