Open Access
ARTICLE
A Universal Activation Function for Deep Learning
1 Department of Computer Engineering, Anyang University, Anyang-si, 14028, Korea
2 Department of ICT Convergence Engineering, Anyang University, Anyang-si, 14028, Korea
* Corresponding Author: Jeong-Joon Kim. Email:
Computers, Materials & Continua 2023, 75(2), 3553-3569. https://doi.org/10.32604/cmc.2023.037028
Received 20 October 2022; Accepted 15 January 2023; Issue published 31 March 2023
Abstract
Recently, deep learning has achieved remarkable results in fields that require human cognitive ability, learning ability, and reasoning ability. Activation functions are very important because they provide the ability of artificial neural networks to learn complex patterns through nonlinearity. Various activation functions are being studied to solve problems such as vanishing gradients and dying nodes that may occur in the deep learning process. However, it takes a lot of time and effort for researchers to use the existing activation function in their research. Therefore, in this paper, we propose a universal activation function (UA) so that researchers can easily create and apply various activation functions and improve the performance of neural networks. UA can generate new types of activation functions as well as functions like traditional activation functions by properly adjusting three hyperparameters. The famous Convolutional Neural Network (CNN) and benchmark dataset were used to evaluate the experimental performance of the UA proposed in this study. We compared the performance of the artificial neural network to which the traditional activation function is applied and the artificial neural network to which the UA is applied. In addition, we evaluated the performance of the new activation function generated by adjusting the hyperparameters of the UA. The experimental performance evaluation results showed that the classification performance of CNNs improved by up to 5% through the UA, although most of them showed similar performance to the traditional activation function.Keywords
Cite This Article
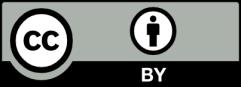