Open Access
ARTICLE
GaitDONet: Gait Recognition Using Deep Features Optimization and Neural Network
1 Department of Computer Science, HITEC University, Taxila, Pakistan
2 Computer Sciences Department, College of Computer and Information Sciences, Princess Nourah bint Abdulrahman University, Riyadh, 11671, Saudi Arabia
3 College of Computer Engineering and Sciences, Prince Sattam bin Abdulaziz University, Al-Kharj, Saudi Arabia
4 Department of Electrical Engineering, College of Engineering, Jouf University, Sakaka 72388, Saudi Arabia
5 Department of Computer Science, College of Computer and Information Sciences, Majmaah University, Al-Majmaah, 11952, Saudi Arabia
6 Department of ICT Convergence, Soonchunhyang University, Korea
* Corresponding Author: Yunyoung Nam. Email:
Computers, Materials & Continua 2023, 75(3), 5087-5103. https://doi.org/10.32604/cmc.2023.033856
Received 29 June 2022; Accepted 04 August 2022; Issue published 29 April 2023
Abstract
Human gait recognition (HGR) is the process of identifying a subject (human) based on their walking pattern. Each subject is a unique walking pattern and cannot be simulated by other subjects. But, gait recognition is not easy and makes the system difficult if any object is carried by a subject, such as a bag or coat. This article proposes an automated architecture based on deep features optimization for HGR. To our knowledge, it is the first architecture in which features are fused using multiset canonical correlation analysis (MCCA). In the proposed method, original video frames are processed for all 11 selected angles of the CASIA B dataset and utilized to train two fine-tuned deep learning models such as Squeezenet and Efficientnet. Deep transfer learning was used to train both fine-tuned models on selected angles, yielding two new targeted models that were later used for feature engineering. Features are extracted from the deep layer of both fine-tuned models and fused into one vector using MCCA. An improved manta ray foraging optimization algorithm is also proposed to select the best features from the fused feature matrix and classified using a narrow neural network classifier. The experimental process was conducted on all 11 angles of the large multi-view gait dataset (CASIA B) dataset and obtained improved accuracy than the state-of-the-art techniques. Moreover, a detailed confidence interval based analysis also shows the effectiveness of the proposed architecture for HGR.Keywords
Cite This Article
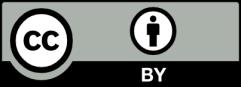