Open Access
ARTICLE
Multi-Classification of Polyps in Colonoscopy Images Based on an Improved Deep Convolutional Neural Network
1 College of Quality and Technical Supervision, Hebei University, Baoding, 071002, China
2 Hebei Technology Innovation Center for Lightweight of New Energy Vehicle Power System, Baoding, 071002, China
3 National & Local Joint Engineering Research Center of Metrology Instrument and System, Hebei University, Baoding, 071002, China
4 College of Electronic Information Engineering, Hebei University, Baoding, 071002, China
5 Department of Orthopedics, Affiliated Hospital of Hebei University, Baoding, 071002, China
6 State Key Laboratory of Molecular Vaccinology and Molecular Diagnostics, Center for Molecular Imaging and Translational Medicine, Department of Laboratory Medicine, School of Public Health, Xiamen University, Xiamen, 361102, China
* Corresponding Authors: Kun Yang. Email: ; Linyan Xue. Email:
Computers, Materials & Continua 2023, 75(3), 5837-5852. https://doi.org/10.32604/cmc.2023.034720
Received 25 July 2022; Accepted 20 October 2022; Issue published 29 April 2023
Abstract
Achieving accurate classification of colorectal polyps during colonoscopy can avoid unnecessary endoscopic biopsy or resection. This study aimed to develop a deep learning model that can automatically classify colorectal polyps histologically on white-light and narrow-band imaging (NBI) colonoscopy images based on World Health Organization (WHO) and Workgroup serrAted polypS and Polyposis (WASP) classification criteria for colorectal polyps. White-light and NBI colonoscopy images of colorectal polyps exhibiting pathological results were firstly collected and classified into four categories: conventional adenoma, hyperplastic polyp, sessile serrated adenoma/polyp (SSAP) and normal, among which conventional adenoma could be further divided into three sub-categories of tubular adenoma, villous adenoma and villioustublar adenoma, subsequently the images were re-classified into six categories. In this paper, we proposed a novel convolutional neural network termed Polyp-DedNet for the four- and six-category classification tasks of colorectal polyps. Based on the existing classification network ResNet50, Polyp-DedNet adopted dilated convolution to retain more high-dimensional spatial information and an Efficient Channel Attention (ECA) module to improve the classification performance further. To eliminate gridding artifacts caused by dilated convolutions, traditional convolutional layers were used instead of the max pooling layer, and two convolutional layers with progressively decreasing dilation were added at the end of the network. Due to the inevitable imbalance of medical image data, a regularization method DropBlock and a Class-Balanced (CB) Loss were performed to prevent network overfitting. Furthermore, the 5-fold cross-validation was adopted to estimate the performance of Polyp-DedNet for the multi-classification task of colorectal polyps. Mean accuracies of the proposed Polyp-DedNet for the four- and six-category classifications of colorectal polyps were 89.91% ± 0.92% and 85.13% ± 1.10%, respectively. The metrics of precision, recall and F1-score were also improved by 1%~2% compared to the baseline ResNet50. The proposed Polyp-DedNet presented state-of-the-art performance for colorectal polyp classifying on white-light and NBI colonoscopy images, highlighting its considerable potential as an AI-assistant system for accurate colorectal polyp diagnosis in colonoscopy.Keywords
Cite This Article
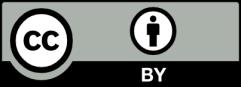