Open Access
REVIEW
Technologies Behind the Smart Grid and Internet of Things: A System Survey
1 Department of Computer Science and Engineering, Lovely Professional University, Punjab, India
2 Department of Artificial Intelligence and Big Data, Woosong University, Daejeon, 34606, Korea
3 Center for ICT & Automotive Convergence, Kyungpook National University, Daegu, 41566, Korea
4 School of Electronics Engineering, Kyungpook National University, Daegu, 41566, Korea
* Corresponding Author: Dong Seog Han. Email:
Computers, Materials & Continua 2023, 75(3), 5049-5072. https://doi.org/10.32604/cmc.2023.035638
Received 28 August 2022; Accepted 17 February 2023; Issue published 29 April 2023
Abstract
Electric smart grids enable a bidirectional flow of electricity and information among power system assets. For proper monitoring and controlling of power quality, reliability, scalability and flexibility, there is a need for an environmentally friendly system that is transparent, sustainable, cost-saving, energy-efficient, agile and secure. This paper provides an overview of the emerging technologies behind smart grids and the internet of things. The dependent variables are identified by analyzing the electricity consumption patterns for optimal utilization and planning preventive maintenance of their legacy assets like power distribution transformers with real-time parameters to ensure an uninterrupted and reliable power supply. In addition, the paper sorts out challenges in the traditional or legacy electricity grid, power generation, transmission, distribution, and revenue management challenges such as reducing aggregate technical and commercial loss by reforming the existing manual or semi-automatic techniques to fully smart or automatic systems. This article represents a concise review of research works in creating components of the smart grid like smart metering infrastructure for postpaid as well as in prepaid mode, internal structure comparison of advanced metering methods in present scenarios, and communication systems.Keywords
The existing technologies in the electricity supply contain information technology (IT) and operational technology (OT) broader aspects. In the OT part, the supply operational infrastructure like supervisory control and data acquisition (SCADA), real-time data acquisition system (RTDAS), and distribution management system are covering the demand side, whereas revenue management system (RMS) mainly, meter reading or billing and collection, geographical information system (GIS), assets management, plant management, etc. are the IT aspects. The combination of the concepts of operational mechanism and economics is used for maintaining the equilibrium dynamically in the demand and supply of the smart energy grid system for improving the efficiency and reliability of the smart grid system [1]. Demand-side management is a need of the current era for deployment in power distribution utilities. While discussing the challenges in traditional energy trading, there are numerous challenges in the operational aspects of the energy smart grid system, the cyber security vulnerabilities such as manipulation of data analysis [2], theft of energy [3], process interruption in the trans-active energy system [1], and energy market [4]. All such challenges can be encountered by potential technologies like blockchain mechanisms [5–7], artificial intelligence, and machine learning techniques [8,9]. Nowadays, the electric meter reading is performed with different techniques in the different power distribution utilities across the globe. A few are running over the manual meter reading with the base billing system and distribution of the bills via post or human representative of the utilities, whereas a few are based on the real-time consumption meter reading in the smart metering infrastructure in both the prepaid as well as the postpaid mode.
In most of the developing countries across the globe like India, the smart postpaid metering system is in use in a few distribution companies whereas power distribution utilities are lacking in the smart prepaid meter functionality due to drawbacks of the reconnection and disconnection automatically with the scheduled triggers from the utility engines. Such triggers are purely dependent on the communication between the metering device and the utility billing engine or metering data acquisition system as per the situation. In the journey of the meters, the metal disk rotating mechanical electrical meter to the smart electronics prepaid as well as postpaid meter, the verities of the metering devices, proposed a few meters reading and bills generation systems. All the systems are unique and are following the main meter reading and bill generation based on the consumption of energy and then revenue collection through various modes. Not only the power supply equipment strengthening can resolve the issue, but the advancement of the revenue management system is also required so that the energy sold by the power trading companies can be realized in terms of money to minimize the commercial loss incurred due to poor billing and collection efficiency across the distribution companies. This paper is intended to study, analyze and represent an overview of the different techniques used in these processes. Section 2 covers the related research; Section 3 poses the research question followed by Section 4 with the research methodology. Section 5 shows the result and discussion, and finally, Section 6 concludes the article.
The prior art of the technologies in the literature on electricity consumption covering energy management, distribution management and revenue management system, the energy smart grid poses challenges in the integration with advanced emerging technologies with traditional legacy electricity infrastructure. These conventional developed intelligent systems like reduction of energy wastage, real-time load on infrastructure, energy prepaid system, energy monitoring and controlling system, energy demand side management, etc. Rapid developments are observed in [10]. The internet presently across the globe is affecting human life in various aspects [11]. Today, this has become ubiquitous, low power wide area (LPWA) technologies are affecting a range of various industries and their aim is investigated in the literature. The LPWA supports an internet of things (IoT) deployment in an electricity theft-detecting framework [12] and defines a category of wireless communication technologies for designing smart channel coding schemes [13]. While reviewing the list of most common technologies of LPWA networks (LPWAN), it is revealed within unlicensed and licensed bands like long-range (LoRa) and narrow-band IoT (NB-IoT) in [14,15]. NB-IoT can be directly deployed in long-term evolution (LTE) or global systems for mobile (GSM) communications networks [16]. A modulation scheme selection was proposed for NB-IoT systems in [17–19]. As far as commercial success is concerned in [20–22].
Another effort of NB-IoT was investigated in [23]. Use of radio frequency (RF) switches integrated circuit (IC) for NB-IoT node which presented in [24]. A prediction of connected devices by 2021 is about 28 billion, of which more than 15 billion will be M2M (Machine to Machine) and consumer-electronic devices only [25]. The network infrastructure is also simplified because of the significant lowering of memory storage requirements in the various parts of the network architecture like mobility management entity (MME), serving gateway (SGW) and the cellular IoT radio access network (CIoT RAN) [26–28]. NB-IoT is used in remote smart energy metering such as the rapid development of smart metering infrastructure [29] and the smart cities in street lighting systems [30]. Power distribution companies are capable of taking meter readings remotely [31]. Lamp posts are available with various modules in [32]. In the case of smart parking, these modules can direct motorists to the nearby free parking space available with the shortest path [33,34]. Automatically optimized systems can be achieved by the NB-IoT fifth generation (5G) [35]. A smart meter is using NB-IoT and is mainly used in gas, water and electricity meter readings infrastructure [36–38]. The limited energy availability raises a challenge in design to ensure a long network lifetime [39]. NB-IoT modules over the battery as the power source can deliver deep indoor penetration [40]. A complex use case of NB-IoT for smart cities is analyzed in [41]. NB-IoT modules with long service life are maintenance-free with a range of 20 dB wider than conventional mobile network connections [42]. Researchers in the literature have focused on energy harvesting as a solution to the deficiency problem with energy in NB-IoT [43–46]. Various research studies including [31,44–46] have proposed energy harvesting as a solution to the energy deficiency problem in NB-IoT. After applying enhanced duty cycles on the circuit of the transceiver, an effective reduction is observed [47].
In the development of effective IoT devices, wireless technology plays a vital role. The authors investigated the IoT evolution propagating towards the new generation technologies such as NB-IoT with 3rd generation partnership project (3GPP) standard and IoT over fifth generation (5G) technology [17]. The expert industry players like Nokia, Huawei and Ericsson are developing their devices with a keen interest in NB-IoT [48]. In the next generation, the main place of technology in wireless communication will be NB-IoT. Such devices for this technology are expected to embed NB-IoT [49] and repeated times utilized in [50–52], whereas the existing others working on this technology for new feature of repetition in [53] and high throughput of LPWAN technology [54]. The LoRa-LPWAN technology is functional under 1 GHz (Giga Hertz), a non-licensed band, for a high range of communication. These are using the modulation in the physical layer (PHY) also called chirp spread spectrum (CSS) modulation. The data is transferred with a low data rate [55]. The battery lifetime used with the IoT devices [56,57]. In the power sectors, IoT devices for the collection of electricity consumption readings are also in the field area. As per [58], the estimated number of connected NB-IoT devices was calculated even more than 50 billion. Every smart handset, smart meter and other communication device is propagating toward the NB-IoT technology-based devices. The hacking or compromising of such devices is very difficult and preventive measurements are implemented over the memory, network utilization, and computations. These devices may attract the hacker’s intention for compromising the system [59]. The challenges in context with the memory management, network operations, energy consumption and compute infrastructure to be considered for designing the architecture of the NB-IoT devices.
A blockchain approach is proposed in [60–63]. The spectrum related to cryptocurrency is used in blockchain technology. The blockchain is focusing on various AI-based applications like expert decision systems [64–66]. In blockchain technology, the block is mainly linked with cryptography having growing records in blocks. All blocks are linked with each other with the help of a hash key encrypted, of the previous block along with the timestamp and data of the records of transactions. Such formations are represented in a Merkle Tree [67]. While considering the previous network topologies of the mobile generations such as 5G technology that is expected a fundamentally transformational role in the telecommunication society [68]. There are a few technologies related to the improvement of energy efficiency, like the cognitive ambient backscatter approach as elaborated by [69]. It is an NB-IoT node with the primary 5G embedded device. The use of NB-IoT is elaborated in [70]. Enhanced network performance in context to lower the latency of the network along with a reduction in power consumption over the shifting of the transmission bulky load to the high-speed network infrastructure.
The baseband units (BBU) were investigated in [71]. Such BSs enable the upper-level cooperation between them to enable the scalability of the network [72], which was researched in [73]. The author in [74] explained the approach with a direct Link interface co-channel (DLI) [74]. An architecture of C-RAN with a 5G Network was proposed by the author of [75]. The use of C-RAN in the NB-IoT system can lower the huge cost of deployment of the network by deploying the network function virtualization (NFV) and implementation with the robust software-defined networking (SDN) algorithm [72]. An error correction approach for the NB-IoT is proposed in [76–79]. The LPWAN technology uses the Star-Topology of the networking, whereas a few are making use of mesh or tree topology. Later, they switched to star topology [80]. The purpose of the NB-IoT proposed for high-coverage network and power consumption [81].
To achieve the above objectives, a systematic review was carried out of the present literature. The papers from the reputed databases were searched with the intent to identify the state of the art in the electricity smart grid scale-up and up-gradation with the emerging techniques in the field of electricity consumption. The motivation of the survey is to find the key performing indicators in the context of reforming of their legacy infrastructure and technologies. The following are the key aspects identified from the prior arts available in the literature:
1. How to scale up and upgrade legacy traditional infrastructure with emerging technologies in the electricity smart grid.
2. How to optimize the present techniques used in the revenue management system of the electricity consumption sector?
3. How to optimize the current techniques of the electricity meter reading to get an accurate measurement of the electricity consumption in real-time to reduce Aggregate Technical and Commercial Losses?
4. How to control the various losses like technical and commercial occurred in power trading?
To achieve the above objectives, a systematic review was carried out of the present literature. The papers from the reputed databases were searched with the intent to identify the state of the art in the electricity smart grid scale-up and upgradation with the emerging techniques in the field of electricity consumption. The various electricity trading utilities serve their valued customers with their optimum satisfaction. The motivation of the survey is to find the key performing indicators in the context reforming of their legacy infrastructure and technologies. The following are the key aspects identified from the prior arts available in the literature.
➢ Categorization Aspects
➢ Deployment Aspects
➢ Operational/Functional Aspects
➢ The core of Revenue Management Aspects
Nowadays, the common type of electricity meter used by the power distribution companies such as the electromechanical meter. This device is measuring electricity consumption with a count of revolutions of a metallic disk which is investigated in [82]. The main types of electricity meters with advantages and disadvantages are shown in Table 1. As there is no comprehensive function associated with the electromechanical meter, these meters are more popular and adopted by power utilities for a long tie due to their reliability in most cases.
At the time, due to complex enhanced requirements related to control of the supply and demand, electronic meters started to replace these meters. These electronic meters are built on digital micro technology (DMT) instead of a moving part. An electronic meter embedded function of remote meter reading (AMR) can measure the electricity consumption by reading the electronic meter from the premises of their valued consumer, without a physical visit by a human being over the consumer’s premises. In addition, smart electricity meters, make it feasible to level loads as per Real-Time Pricing (RTP) [10]. In the future, the prepaid smart metering infrastructure can help power distribution companies to achieve the set targets in an efficient manner. The traditional electromechanical meters were the first robust meters used in the previous period, further, the RS232/RS485 (Recommended Standard 232/Recommended Standard 485) port-embedded electric meters were another type of electric meter. The automated AMR or remote meter reading (RMR) was able to communicate in the unidirectional for the purpose of the meter reading only. Without physically visiting the premises of the consumers, such electric meters were capable of allowing the utility to read over long distances one-way, to stimulate the demand side management (DSM), advanced meter infrastructure (AMI) came into the existence with both directional communications. AMI development was one of the important steps in intelligent energy networks (IENs) [84]. The smart meter can work in both modes, i.e., prepaid as well as in postpaid mode in Table 2.
Recent development in such devices, as well as in the postpaid mode. Recent development in such devices, along with the infrastructure of IENs in [84], enables the following functions:
▪ Bi-directional communication in smart devices and utility.
▪ Controlling and managing devices remotely.
▪ Automatic and bi-directional metering and billing customers accordingly.
▪ Outage management system by detecting and diagnosing system faults.
▪ Real-time data acquisition system formulations.
▪ Storing and managing the metering information at the central place.
▪ Discovery of other complicated applications, e.g., detection of electricity theft, enhancement of system security, DSM and load balancing.
Deployment of smart meters across the globe is exponentially increased and major devices are installed in India, China and other PAC (Pacific) countries. Fig. 1 is depicted the expected growth in the number of connected devices in billions. In a journey towards the enhancement the strengthening of the infrastructure, the higher authorities keep on providing the best schemes like Revamped Distribution Sector Scheme (RDSS), The prime aim of the scheme is to improve the quality, reliability and affordability of power supply through digitalization and network improvement. The target is to bring the Aggregate Technical & Commercial Loss (AT& C) levels to 12%–15% by FY and ACS—ARR (Average cost of supply per unit of power—average revenue realized) gap to zero by 2024-25 [85,86]. Smart metering solutions have been deployed in India for more than two years, but it is at a very early stage. Initially, there was an issue around acceptance with consumer resistance, but gradually, the development started increasing in different states. The management campaigning to install smart meters for all residential customers by 2025. The installation has increased significantly. In the year 2021–2025, India will install approx. 250 million smart meters [87].
Figure 1: Expected growth in the number of connected devices in billions with a prediction by using a linear regression algorithm
As per a study carried out by IoT research firm Berg Insight, approximately 572.3 million Smart Metering Devices are expected to be deployed during the year 2021–2025 in the APAC (Asia-Pacific) covering countries like China, India, Japan and South Korea. At the end of 2019, the overall penetration of smart meters stood at 69% in these countries which is corresponding to an installed base of approx. 653.3 million units [88]. The electricity grid is a critical entity and is the backbone of the power system [89], in the context of infrastructure. For efficient microgrid operations and real-time decisions, deep learning techniques in the bidirectional gated recurrent unit for stability prediction [90], Annealing algorithm [91] and Significant methods [92] are key to impacting direct parameters. Renewable energy resources like PV/Solar Panels in the smart grid have led the modern era of the electricity system investigated in [93]. Researchers highlighted that the Malaysian Government has set an ambitious target to achieve greater penetration of renewable energy [94]. Distinguished data aggregation framework based on the blockchain and homomorphic encryption [95].
4.3 Operational/Functional Aspect
As smart meters are based on bi-directional communication. In most of the parts of the world, smart meters move to prepaid mode, whereas it is shown in both modes in prepaid as well as postpaid mode in a few countries. Net energy metering (NEM) is also achieved by smart meters. There are some systems as follows:
Energy Management System: This system is for controlling, monitoring, measuring, and optimizing the usage of electricity in smart homes and industry of the factory premises with computerized software with various actuators, sensors, detectors, etc.
Demand Response Techniques: These techniques are responding to the instantaneous demand and help in the reduction of costs and fluctuations with the elegance of the services like frequency regulation, spinning reserves and operating reserves with implementation for smart gird reliability improvement.
Big Data Analytics: As the data of the smart devices are the instantaneous data with an interval of approx. 15 min. And a huge data is accumulated in the metering data acquisition system. A big data analytics tool analyses these data in real-time with high speed by using map-reduce frameworks on the HDFS (Hadoop Distributed File System) platform.
Artificial Intelligence & Machine Learning Algorithms: These emerging techniques work on the past consumption history of electricity with statistical formulas like ARIMA (Autoregressive integrated moving average) for the prediction of the demand on real-time analysis along with other variables like weather parameters etc.
Smart Power Meters: A few functions applied and deployed in the smart meters are enumerated as below: Regular and Precise Metering: Precise metering at a predefined interval is the main basic function of any metering system of any utility like electricity, gas, or any other type. The important purpose of any IEN is to reduce technical aggregation and commercial loss. There is a requirement in the present smart metering infrastructure to get the information from the smart machines [96] more frequently on an hourly or at fewer periodical intervals. We can take the example of an American utility PG&E where their metering system accumulates the consumption data by default on an hourly basis whereas the metering systems at center-point energy & Oncor reads it at an integral of only 15 min. There also exists the NEM, a metering technology, in the smart meters where the consumers must pay only the cost of the excel amount of the electricity as per the final net metering data, such meters are provided by center-point energy for use in multi-channel metering technology.
Data Recording and Alarming: Data acquisition and alarming is one of the common functions of smart meters for monitoring the status of energy networks. It is for valued consumers for awareness of the real-time consumption of their electricity usage. This relates to precise and interval-based meter readings and generating alarms accordingly. This function enables the meter to serve in the prepaid mode also. For example, Chinese vendors provide meters with such prepaid mode functionality.
Two-way Communication: Smart meters can communicate both ways, i.e., with a bi-directional function with interactive information communications between the utilities and the consumers. To improve the reliability of the smart meters, the functions of the communication module are independent of the basic functions like meter recording, alarming, etc. Such machines are working in both offline mode and online mode. Table 3 elaborates on the methods of communication with their specific variables of the comparison. Based on the information, the range, costing, data rates, frequency, technology maturity and bandwidth can be enumerated as the specific communication methods for use in the different techniques for the meter reading, invoice generation and revenue collection activities in the electricity consumption paradigm.
Appliance Control: These appliances are, washing machines, geysers, air conditioners, etc. These devices are to maintain the maximum load at a specific time to control the energy invoices to skip from the overload drawn instantly. This can be achieved by switching off the specific appliance as per the status of the electric power parameters.
Demand-side management: Apart from the functions enumerated above, the demand-side management (DSM) functions of the smart electricity functions are enabling many more comprehensive applications. Dynamic-Pricing-Mechanisms are important for this management that can significantly affect the behavior of the consumers. Commonly used methods are as follows:
Time of use (TOU)–multiple tariffs are used for peak and valley time.
Incremental-Pricing (IP)–increment in the tariff is directly proportional to consumption growth.
Critical-Peak-Pricing (CPP)–a flat rate is applied till the critical situation where a very high price is applied in the case of the critical situation.
Critical-Peak-Rebate (CPR)–consumers are motivated to reduce their consumption at the time of peak load time.
RTP-market price is a floating price and is decided by the market Demand & Supply of the electricity.
Detection of electricity theft and enhancing system security: With the help of advanced technology, electric power is transmitted across geographical areas and time zones with a challenge, power theft prohibits the power distribution utilities from fully benefiting from the development. As this power theft is an issue worldwide among utilities and several attempts are made to resolve the issue with the help of smart metering infrastructure. Smart meters embedded with numerous sensors can intelligently detect the tempering, fault and unauthorized access to the smart meters/devices. As smart meters are networked with the infrastructure, so the chances of hacking, malware, or any other cyber risks are increasing rapidly. For mitigating such issues, the operations technology must be kept with up-to-date patches. Best practices must be adopted to keep the network isolated from open internet access and should keep updating its attacking techniques and tools.
Smart distribution: These are the self-optimizing, Self-balancing and self-healing including the super-conducting cables for automated monitoring over the long-distance transmission of electricity. Smart sub-stations: These manage the critical and non-critical direct variables like power factor performance, transformers, breakers and battery status by monitoring and controlling such operational data.
4.4 Core Revenue Management Aspects
Across the globe, there are emerging technologies in the core process of revenue management systems i.e., electricity meter reading, energy invoice Generating and Revenue Collection against energy consumption. A few are the findings in context with the existing techniques in the electricity meter reading, energy invoice generations and the revenue collections techniques as below:
Electricity meter readings techniques: In approximately, all the electricity trading companies, meter reading techniques are very common. The electricity meter reading techniques are investigated in Fig. 2. The traditional metering techniques are the bottleneck in the achievement of the targets set annually by the respective regulatory commissions. In these techniques, Kilo Watt Hour (KWH), Voltage (V), Power Factor (pf), Frequency (f), Kilo Volt Ampere Hour (KVAH) and max Load are the direct variables to compare and analyze.
Figure 2: Electricity meter reading process
Metering functions: Metering functions are mostly used in electricity consumption. It has importance in the core functioning of the revenue management system in electricity consumption such as data recording, total consumption, Time of-use metering, peak demand metering, load survey, recording of a power outage, etc.
Monitoring and control: The monitoring and control variables are to achieve the operational functionality in the electricity consumption, for example, reporting of a real-time power outage, detection of leak and tampering, control and Scheduling of load, etc.
Customer interface: To service the citizens in a better way, the customer interface variables are security, billing functions and energy management systems for the customer management in the electricity consumption:
Communications metering techniques: In the communication of electricity metering, the traditional systems are the mechanical meters where the metal disk is rotating and the per rotations cycle consumption was calculated as shown in Fig. 3. The automatic meter reading, remote meter reading and AMI are the modern metering system. Communication metering techniques are available for electricity consumption such as electromechanical meters, automated/remote meter readings, advanced metering infrastructure and smart metering.
Figure 3: Communication methods used in the metering system of the electricity consumption
Due to the large-scale deployment of the emerging techniques in the electricity smart grids in context with the time span, the heterogeneous techniques in the same utility are operational. Based on the survey of these techniques, the followings are a detailed analysis of the findings:
5.1 Benefits and Costs of Smart Meters
Smart meters drastically, reformed electricity trading. Some of the benefits of the applications of smart meters are enumerated from a stakeholder’s perspective in Table 4. On one side, the consumers are benefited from the real-time consumption in units as well as in monetary terms, on the other hand, the power distribution utility end. The quality of the supply is maintained for supply 24/7 in a better way. Table 4 enumerates the various applications of the smart meters in context with different stakeholder perspectives like market forecasting, load forecasting, transmission and distribution perspective, billing services along with security, etc. and finally the customers and utilities. In the present era, all the electricity distribution companies are restructuring and reforming their traditional infrastructure to strengthen the smart grids. The following are the recommendation based on the findings above, in context to the metering, billing and collection activities in the electricity consumption:
In summary of the smart grid techniques, metering device techniques, especially the smart meters are depicted in Tables 1 and 4. Its functions and applications along with the implications, cost and benefits.
Challenges and future directions: The EV (Electric Vehicle) charging infrastructure is the main challenge in the recent era, in the future on the roads of developing countries the traffics will cover most of the vehicles with electric energy. The smart pre-paid metering infrastructure in developing countries like India will move completely to the smart metering infrastructure.
The heavy aggregate and commercial losses reported by most of the power distribution companies will be minimized with the help of pre-paid smart metering. The huge amount converted to bad debts in the energy sector will be able to get the money in advance in the form of recharges. The online recharge facilities through mobile applications, web portals, etc. can enhance the revenue collection part with positive change. The key role is played by communication technologies. An efficient communication technology is required to manage the real-time data of the electricity consumption and respond with optimum load shedding and demand response, ensuring the lost cost, safe and reliable services in the electricity distribution system as the various actuators, sensors and smart device like smart meters are connected to the central systems through this communication media.
The more pressing challenges of outlier data treatment toward smart energy management are outlier data treatment procedures of the Smart Grid environment from the perspective of big data analytics as information security technologies with scenarios of outlier denial and outlier mining as power system operation [102].
In order to reduce overall technical and financial losses and increase the efficiency of the energy supply, the constant advancement of SGs algorithms relies on the adoption of cutting-edge methods for addressing network capacity issues in the energy industry [103]. Users of the services must add renewable energy to the grid in order for green energy algorithms to integrate local power generation sources into the main grid. This synergy benefits both SGs and consumers through various compensation plans [104].
When considering the potential, smart grids are supporting efficient and optimal energy use, green communication via 5G and infrastructure for recharging electric vehicles. The integration of social and economic networks with cyber-physical systems in Table 5. With the aid of smart grid technology, the outliner enables aberrant patterns with real-time power generation analytics, starting from generation companies to transmission and ultimately to the end-users through distributors. With the help of efficient communication tools, the creation of microgrids outfitted with sensor systems and cloud computing infrastructures, the administration of power transmission is appropriately controlled, resulting in intelligent electrical grids.
Presently, modern distribution companies are moving towards the smart metering functionality of electricity meters in the prepaid mode of effective control over electricity usage. This approach is helping the utilities in the reduction of the AT & C loss and enhancement of the quality of the power supply. Fig. 1 depicts the expected growth in the number of connected devices in billion across the world for mobile phones PC/Tablets vs. the M2M devices and consumer electronics across the world. Fig. 2 depicts the electricity meter reading techniques along with the algorithms and communication used in the electricity consumption.
Fig. 3 depicts the communication methods available and used in the electricity meter reading techniques for the measurement of the power usage by the consumers of the electricity consumption. Fig. 4 depicts the complexity of the smart grid with the bi-directional flow of electricity as well as information between the electric assets. Fig. 5 depicts the IoT techniques comparison in data rates and coverage of the area with costing.
Figure 4: An overview of electric smart grid complexity
Figure 5: IoT techniques comparison in data rates and range coverage with cost
Table 1 elaborates on the types of electricity meters with their pros and cons with the functional control variables like communication mode, payment modes in prepaid or postpaid as well as in the NEM to monitor the two-way flow of the electricity and measure the same for invoice purposes. The following are the traditional/legacy electric meter reading techniques. Table 2 shows the comparative study of the different metering systems available these days, along with the traditional metering systems with the mode, measurement and methods of energy controls.
In recent times, there is a rapid revolutionary growth of technology in the energy sector, especially electric power consumption as described in Table 6. Government formulates the different policies for the optimized generation of electricity, transmitting the same to the end users domestic, non-domestic, agricultural, or industrial consumers, etc. If we go back two decades back, we find the human meter reader visiting every house with a register and pen and noting down the meter readings, these readings are sent to the utility offices for the generation of the energy invoices and then dispatched by the same human being or by post to the end user customer. After receipt of the energy bills, the customer visited the collection counters of the electricity department and paid their bills in cash after a long queue. This process is simply composed of the broad three processes for the power distribution companies such as electricity meter reading, energy invoice generation and revenue collection. Technology played a vital role in these processes and minimized human intervention and reached the accuracy and timely generation of the bills in return for the new collection, the different digital payment collection options like e-wallets, online methods like payment gateways and various Customized Mobile Applications facilitated the electricity consumers for the timely payment for the electricity consumption. The summary of the benefits of smart grids is as follows.
▪ Capacity scalability by metering the increasing energy demands. This increasing demand is a vital issue in the electricity supply and it can easily be handled with smart grids.
▪ Facilitates e-vehicles recharge at a broad scale. The next generation of vehicles is electricity based, the smart grid with e-vehicle recharging, drastically helping the vehicle world with ease.
▪ Elegance of renewable power feasibility via micro-grids with solar cells/panels, windmills, etc. a full-fledged smart grid equipped with intelligent electronic devices can cater to the load shedding automatically with the priority options. Load curtailment at the consumer’s side with smart devices can manage the black-out caused due to sudden frequency drops from the generation side.
▪ Reduces the cost of electricity production as well as consumption. Electricity can be generated as per real-time demand analysis on the metering data acquisition dashboard.
▪ Decreases the likelihood of blackouts, surges and burnouts. Smart devices embedded in the smart grids like smart meters, IoT-based devices for controlling and managing the grid operations on the real-time bases with the enhanced decision support system of MDAS (Metering Data Acquisition System).
▪ Near to real-time control of consumers over the energy bills by real-time consumption patterns and prepaid, postpaid and presumes facilities.
The limitations include the functional aspects of not carrying out accurate and complete meter readings against actual electricity consumption. There are challenges in the effective monitoring and controlling of the smart grid intelligent devices in real time to maintain the quality of the electricity supply. The scalability in the smart grids with respect to the emerging technologies in the IoT/Smart intelligent devices/Smart grids is a big challenge. There is a big gap in the industry practice with a stabilized solution to the new-born technologies. The communication needs to be upgraded to the latest i.e., 5G/6G, LPWAN, NB-IoT, etc. with strengthened infrastructure. The consumer interface needs to be embedded with a dashboard to show the real-time consumption of electricity. The recommendations suggest the functional aspects for accounting for the trading of complete electricity consumption near to real-time with accuracy.
In the current scenarios, the transactions are drastically increasing to monitor the consumption of electricity in real time. The data from the smart meters are accumulated at the interval of fifteen minutes, which calculates the energy charges with the various parameters of the energy tariffs to deduct the balance in the smart pre-paid mode. Real-time analytics is the demand of such smart grid infrastructure. Future research trends need to be focused on the speed of the processing and storage of the data smart catering to the variety of data. The velocity, volume, variety, veracity and value give a picture of big-data analytics. The Hadoop distributed file system, a map-reduce framework for fast processing and big data analytics can be a boom for the world of the smart grid to provide the qualitative supply of electricity with optimum cost benefits.
This work reviewed the literature on smart grids, narrowband Internet of Things, Wireless Networks and Intelligent electronic devices like smart meters. The papers are from SCI and Scopus-indexed journals published from 2011 onwards. We carried out a systematic survey of the literature on smart grid technologies. A list of 590 research papers with different aspects of smart grid techniques is filtered. Finally, 79% of papers from recent last six years are on emerging technologies of smart grids related to IoT are 20%, NB-IoT at 24%, smart intelligent systems at 16% and 21% from the networking area. We studied and analyzed the various techniques used in the electricity meter reading, bill generation, and revenue collection of the electric power consumption in the smart grid. There exist some distribution companies where the legacy systems and equipment are to be exchanged to a great extent. The revamp of such infrastructure is mandatory to move to the advanced smart grid infrastructure. A discussion on related works in the emerging trends of technologies in the advancement of smart energy grids is carried out. Then we analyzed the process for the meter-reading, invoice generation and revenue collections in the power sector in the context of its functions, monitoring and control, along with the consumer interface and communication techniques. The smart prepaid mode in the utilities can resolve the issue of heavy debts, especially in developing countries where the huge loss is a major burden to the public due to unawareness of the electricity values. The consumers will use the electricity as per allotted funds for the electricity as done in case of mobile usage. The prepaid concept can minimize aggregate technical and commercial loss up to the maximum extent. Especially we mainly targeted the various technologies in revamping the IoT-based smart grid. Further, we plotted the comparison of different techniques of meter billing collection in the context of electricity consumption. Finally, some incoming research-related suggestions have been considered based on our study.
We can develop a framework that can mitigate the issues of the non-receipt near to real-time electricity meter readings due to numerous reasons like no physical visit of the meter reader and non-availability of the network services by developing an IoT device to facilitate the existing RS232 embedded Electricity Meter to convert with the functionality of the smart meter in postpaid as well as prepaid mode. The non-receipt of the bills may also be mitigated with the missed call framework. The same type of framework may be sued for the no-power supply complaint lodging with ease.
Funding Statement: This work was supported by Basic Science Research Program through the National Research Foundation of Korea (NRF) funded by the Ministry of Education (2021R1A6A1A03043144), and Woosong University Academic Research in 2022.
Author Contributions: Kuldeep Sharma and A. S. M. Sanwar Hosen contributed to the conception and design, acquisition of data, analysis and interpretation of data. Arun Malik supervised the work and conceived the analysis part. Isha Batra contributed to data collection. Dong Seog Han helped in the implementation part. Md. Abdul Latif Sarker worked on the accuracy and integrity of work. All authors have read and agreed to the published version of the manuscript.
Conflicts of Interest: The authors declare that they have no conflicts of interest to report regarding the present study.
References
1. Y. Zhang, V. V. G. Krishnan, J. Pi, K. Kaur, A. Srivastava et al., “Cyber-physical security analytics for transactive energy systems,” IEEE Transactions on Smart Grid, vol. 11, no. 2, pp. 931–941, 2020. [Google Scholar]
2. W. L. Chin, W. Li and H. Chen, “Energy big data security threats in IoT-based smart grid communications,” IEEE Communications Magazine, vol. 55, no. 10, pp. 70–75, 2017. [Google Scholar]
3. W. Li, T. Logenthiran, V. Phan and W. L. Woo, “A novel smart energy theft system (sets) for IoT-based smart home,” IEEE Internet of Things Journal, vol. 6, no. 3, pp. 5531–5539, 2019. [Google Scholar]
4. C. Barreto, H. Neema and X. Koutsoukos, “Attacking electricity markets through IoT devices,” Computer, vol. 53, no. 5, pp. 55–62, 2020. [Google Scholar]
5. M. U. Hassan, M. H. Rehmani and J. Chen, “Privacy preservation in blockchain-based IoT systems: Integration issues, prospects, challenges, and future research directions,” Future Generation. Computer Systems, vol. 97, pp. 512–529, 2019. [Google Scholar]
6. G. Rathee, A. Sharma, R. Kumar and R. Iqbal, “A secure communicating things network framework for industrial iot using blockchain technology,” Ad hoc Networks, vol. 94, no. 101933, pp. 1–15, 2019. [Google Scholar]
7. D. Minoli, “Positioning of blockchain mechanisms in IoT-powered smart home systems: A gateway-based approach,” Internet of Things, vol. 10, no. 100147, pp. 1–17, 2020. [Google Scholar]
8. S. U. Jan, S. Ahmed, V. Shakhov and I. Koo, “Toward a lightweight intrusion detection system for the internet of things,” IEEE Access, vol. 7, pp. 42450–42471, 2019. [Google Scholar]
9. Z. Ullah, F. Al-Turjman, I. Mostarda and R. Gagliardi, “Applications of artificial intelligence and machine learning in smart cities,” Computer Communications, vol. 154, pp. 313–323, 2020. [Google Scholar]
10. K. Sharma and A. Malik, “An efficient IoT based framework for missed call messaging services for electricity consumers,” Communications in Computer and Information Science, vol. 1394, pp. 83–100, 2021. [Google Scholar]
11. P. Sethi and S. R. Sarangi, “Internet of things: Architectures, protocols and applications,” Journal of Electronic and Computer Engineering, vol. 2017, pp. 1–25, 2017. [Google Scholar]
12. K. Sharma, A. Malik and I. Isha, “An efficient IoT based electricity theft detecting framework for electricity consumption,” in Proc. ICCS, Phagwara, India, pp. 244–248, 2021. [Google Scholar]
13. M. E. Migabo, K. Djouani, A. Kurien and T. Olwal, “A comparative survey study on lpwa networks: Lora and nb-iot,” in Proc. FTC, Vancouver, BC, Canada, pp. 1045–1051, 2017. [Google Scholar]
14. K. E. Nolan, W. Guibene and M. Y. Kelly, “An evaluation of low power wide area network technologies for the internet of things,” in Proc. IWCMC, Paphos, Cyprus, pp. 439–444, 2016. [Google Scholar]
15. K. F. Muteba, K. Djouani and T. O. Olwal, “Opportunistic resource allocation for narrowband internet of things: A literature review,” in Proc. ICECCE, Istanbul, Turkey, pp. 1–6, 2020. [Google Scholar]
16. A. R. Alvarino, M. Vajapeyam, H. Xu, X. Wang, Y. Blankenship et al., “An overview of 3gpp enhancements on a machine-to-machine communications,” IEEE Communication Magazine, vol. 54, no. 6, pp. 14–21, 2016. [Google Scholar]
17. C. Yu, I. Yu, Y. Wu, Y. He and Q. Lu, “Uplink scheduling and link adaptation for narrowband internet of things systems,” IEEE Access, vol. 5, pp. 1724–1734, 2017. [Google Scholar]
18. J. Lee, “Prediction-based energy-saving mechanism in 3gpp nb-iot,” Sensors, vol. 17, no. 2, pp. 1–22, 2017. [Google Scholar]
19. P. Massam, P. Bowden and T. Howe, “Narrow band transceiver,” Patent, vol. WO 2008: 065414, no. EP 2092682 B1, pp. 1–22, 2013. [Google Scholar]
20. GSMA industry, “Low power wide area connectivity,” 2016. [Online]. Available: https://www.gsma.com/iot/wp-content/uploads/2016/03/Mobile-IoT-Low-Power-Wide-Area-Connecti-vity-GSMA-Industry-Paper.pdf [Google Scholar]
21. Huawei Technologies, “Nb-iot—Enabling new business opportunities,” 2015. [Online]. Available: https://www.huawei.com/minisite/4-5g/img/NB-IOT.pdf [Google Scholar]
22. C. B. Mwakwata, H. Malik, M. Mahtab Alam, Y. L. Moullec, S. Par et al., “Narrowband internet of things (nb-iotFrom physical (phy) and media access control (mac) layers perspectives,” Sensors, vol. 19, no. 11, pp. 2613, 2019. [Google Scholar] [PubMed]
23. RF Design, “Narrowband iot (nb-iot)—RF design,” 2017. [Online]. Available: https://rf-design.co.za/2017/12/05/narrowband-iot-nbiot-2/ [Google Scholar]
24. F. Z. Y. Dawji, “Integrated antenna switch for NB-IoT,” M.S. Dissertations, Lund University, Sweden, 2018. [Google Scholar]
25. S. Landstrom, J. Bergstrom, E. Westerberg and D. Hammarwall, “nbiot: A sustainable technology for connecting billions of devices,” Ericsson, Stockholm, Sweden, Tech. Rep., vol. 101, no. 1, pp. 1–12, 2016. [Google Scholar]
26. K. Spees and L. Lave, “Impacts of responsive load in pjm: Load shifting and real time pricing,” The Energy Journal, vol. 29, no. 2, pp. 101–22, 2008. [Google Scholar]
27. P. A. Maldonado, P. Ameigeiras, J. Prados-garzon, J. Ramos-munoz and J. Lopez-soler, “Virtualized mme design for iot support in 5g systems,” Sensors, vol. 16, no. 8, pp. 1338, 2016. [Google Scholar]
28. P. Salva-garcia, J. M. Alcaraz-calero, Q. Wang, J. B. Bernabe and A. Skarmeta, “5g nb-iot: Efficient network traffic filtering for multitenant iot cellular networks,” Secure Communication Network, vol. 2018, pp. 1–21, 2018. [Google Scholar]
29. S. Bell, “Virtualization: A critical capability for service provider success in IoT, 5G & beyond,” Technology Report, vol. 317, CA, USA, 2017. [Google Scholar]
30. P. Kaipainen, “Nb-iot extends the opportunities of smart metering,” 2018. [Online]. Available: https://eu.landisgyr.com/blog/nb-iot-extends-the-opportunities-of-smart-metering [Google Scholar]
31. S. Chen, G. Xiong, J. Xu, S. Han, F. Y. Wang et al., “The smart street lighting system based on nb-iot,” in Proc. CAC, Xi’an, China, pp. 1196–1200, 2018. [Google Scholar]
32. M. Pennacchioni, M. G. Benedette, T. Pecorella, C. Carlini and P. Obino, “NB-IoT system deployment for smart metering: Evaluation of coverage and capacity performances,” in Proc. AIAC, Cagliari, Italy, pp. 1–6, Sep. 2017. [Google Scholar]
33. B. Abinaya and S. Guru Priya, “IoT based smart and adaptive lighting in street lighting,” in Workshop, Dept. Inf. Technol., Sri Sairam Engineering College, Tamilnadu, India, pp. 195–198, 2017. [Google Scholar]
34. J. Shi, I. Jin, J. Li and Z. Fang, “A smart parking system based on NB-IoT and third-party payment platform,” in Proc. ISCIT, Cairns, Queensland, Australia, pp. 1–5, 2017. [Google Scholar]
35. T. Lin, H. Rivano and F. L. Moule, “A survey of smart parking solutions,” IEEE Transaction Intelligent Transportation Systems, vol. 18, no. 12, pp. 3229–3253, 2017. [Google Scholar]
36. R. S. Sinha, Y. Wei and S. H. Hwang, “A survey on lpwa technology: Lora and nb-iot,” ICT Express, vol. 3, no. 1, pp. 14–21, 2017. [Google Scholar]
37. R. Zhang, S. Cui and C. Zhao, “Design of a data acquisition and transmission system for smart factory based on nb-iot,” in Proc. Springer ICCSPS, Dalian, China, vol. 517, pp. 875–880, 2018. [Google Scholar]
38. A. Adhikary, X. Lin and Y. P. Wang, “Performance evaluation of nb-iot coverage,” in Proc. IEEE VTC, QC, Canada, pp. 1–5, 2016. [Google Scholar]
39. O. Elijah, T. A. Rahman, I. Orikumhi, C. Y. Leow and M. N. Hindia, “An overview of internet of things (IoT) and data analytics in agriculture: Benefits and challenges,” IEEE Internet of Things, vol. 5, no. 5, pp. 3758–3773, 2018. [Google Scholar]
40. C. Y. Yeoh, A. B. Man, Q. M. Ashraf and A. K. Samingan, “Experimental assessment of battery lifetime for commercial off-the shelf nb-iot module,” in Proc. ICACT, Gangwon-do, South Korea, pp. 223–228, 2018. [Google Scholar]
41. M. Lauridsen, R. Krigslund, M. Rohr and G. Madueno, “An empirical nb-iot power consumption model for battery lifetime estimation,” in Proc. IEEE VTC, Porto, Portugal, pp. 1–5, 2018. [Google Scholar]
42. M. E. Soussi, P. Zand, F. Pasveer and G. Dolmans, “Evaluating the performance of emtc and nb-iot for smart city applications,” in Proc. ICC, Kansas City, USA, pp. 1–7, 2018. [Google Scholar]
43. E. M. Migabo, K. D. Djouani and A. M. Kurien, “The narrowband internet of things (NB-IoT) resources management performance state of art, challenges, and opportunities,” IEEE Access, vol. 8, pp. 97658–97675, 2020. [Google Scholar]
44. V. Spajic, “Narrowband internet of things,” Infoteh Jahorina, vol. 16, pp. 201–206, 2017. [Google Scholar]
45. A. Haridas, V. S. Rao, R. V. Prasad and C. Sarkar, “Opportunities and challenges in using energy-harvesting for nb-iot,” The ACM SIGBED Review, vol. 15, no. 5, pp. 7–13, 2018. [Google Scholar]
46. O. Khan, A. Niknejad and K. Pister, “Ultra-low-power transceiver soc designs for iot, nb-iot applications,” in Proc. CICC, San Diego, USA, pp. 1–77, 2018. [Google Scholar]
47. M. Basharat, W. Ejaz, M. Naeem, A. M. Khattak, A. Anpalagan et al., “Energy efficient resource allocation for noma in cellulariot with energy harvesting,” in Proc. ICET, Islamabad, Pakistan, pp. 1–6, 2017. [Google Scholar]
48. R. Fedele, M. Merenda and F. Giammaria, “Energy harvesting for iot road monitoring systems,” Instrumentation Mesure Métrologie, vol. 18, no. 4, pp. 605–623, 2018. [Google Scholar]
49. G. Anastasi, M. Conti, M. Di Francesco and A. Passarella, “Energy conservation in wireless sensor networks: A survey,” Ad hoc Network, vol. 7, no. 3, pp. 537–568, 2009. [Google Scholar]
50. S. Agnes, “Intel, Nokia and Ericsson collaborate on NB-LTE wireless for IoT,” Capacity Magazine, Helsinki, Finland, Tech. Rep., vol. 915, pp. 1–5, 2015. [Google Scholar]
51. Y. P. E. Wang, X. Lin, A. Adhikary, A. Grovlen, Y. Sui et al., “A primer on 3gpp narrowband internet of things,” IEEE Communication Magazine, vol. 55, no. 3, pp. 117–123, 2017. [Google Scholar]
52. W. Yang, M. Hua, J. Zhang, T. Xia, J. Zou et al., “Enhanced system acquisition for NB-IoT,” IEEE Access, vol. 5, pp. 13179–13191, 2017. [Google Scholar]
53. J. Axmon, J. Bergman, D. Hao and M. Kazmi, “Application of timing advance command in wireless communication device in enhanced coverage mode,” U.S. Patent, 0 288 845, 2017. [Google Scholar]
54. F. Luo and C. Zhang, Signal Processing for 5G: Algorithms and Implementations. Hoboken, NJ, USA: Wiley-IEEE-press, 2016. [Online]. Available: https://ieeexplore.ieee.org/servlet/opac?bknumber=7572254 [Google Scholar]
55. J. Huusko, “Communication performance prediction and link adaptation based on a statistical radio channel model,” Technical Report, University of Oulu, Finland, C563, 2016. [Google Scholar]
56. A. Sharif, V. M. Potdar and R. F. Ahmad, “Adaptive channel coding and modulation scheme selection for achieving high throughput in wireless networks,” in Proc. IEEE ICAINA, Perth, WA, Australia, pp. 200–2007, 2010. [Google Scholar]
57. Y. Ji and H. Yang, “Comparison of lora and NB-IoT,” Network Communication Technology, vol. 4, no. 1, pp. 13, 2019. [Google Scholar]
58. J. Nordlof, “A study of low-power wide-area networks and an in-depth study of the lorawan standard,” M.S. Dissertation, KTH Royal Institute of Technology, Sweden, 2018. [Google Scholar]
59. M. Bor and U. Roedig, “LORA transmission parameter selection,” in Proc. DCOSS, Ottawa, Canada, pp. 5–7, 2017. [Google Scholar]
60. L. Atzori, A. Iera and G. Morabito, “The internet of things: A survey,” Computer Network, vol. 54, no. 15, pp. 2787–2805, 2010. [Google Scholar]
61. M. A. Khan and K. Salah, “IoT security: Review, blockchain solutions and open challenges,” Future Generation Computer Systems, vol. 82, pp. 395–411, 2018. [Google Scholar]
62. H. R. Hasan and K. Salah, “Combating deepfake videos using blockchain and smart contracts,” IEEE Access, vol. 7, pp. 41596–41606, 2019. [Google Scholar]
63. A. Suliman, Z. Husain, M. Abououf, M. Alblooshi and K. Salah, “Monetization of IoT data using smart contracts,” IET Network, vol. 8, no. 1, pp. 32–37, 2019. [Google Scholar]
64. A. Chaer, K. Salah, C. Lima, P. P. Ray and T. Sheltami, “Blockchain for 5G: Opportunities and challenges,” in Proc. IEEE GLOBECOM, Waikoloa, USA, pp. 1–6, 2019. [Google Scholar]
65. K. Salah, M. H. U. Rehman, N. Nizamuddin and A. Al-fuqaha, “Blockchain for AI: Review and open research challenges,” IEEE Access, vol. 7, pp. 10127–10149, 2019. [Google Scholar]
66. W. Samek, T. Wiegand and K. R. Muller, “Explainable artificial intelligence: Understanding, visualizing and interpreting deep learning models,” 2017. [Online]. Available: https://arxiv.org/abs/1708.08296 [Google Scholar]
67. T. N. Dinh and M. T. Thai, “AI and blockchain: A disruptive integration,” Computer, vol. 51, no. 9, pp. 48–53, 2018. [Google Scholar]
68. M. Schluse, M. Priggemeyer, L. Atorf and J. Rossmann, “Experimentable digital twins—Streamlining simulation-based systems engineering for industry 4.0,” IEEE Transactions on Industrial Informatics, vol. 14, no. 4, pp. 1722–1731, 2018. [Google Scholar]
69. A. Narayanan, Bitcoin and Cryptocurrency Technologies. Princeton, NJ, USA: Princeton Univ. Press, 2016. [Google Scholar]
70. Gsma, “Road to 5g: Introduction and Migration,” 2018. [Online]. Available: https://www.gsma.com/futurenetworks/wp-content/uploads/2018/04/Road-to-5G-Introduction-and-Migration_FINAL.pdf [Google Scholar]
71. D. Darsena, G. Gelli and F. Verde, “Modeling and performance analysis of wireless networks with ambient backscatter devices,” IEEE Transactions on Communication, vol. 65, no. 4, pp. 1797–1814, 2017. [Google Scholar]
72. Y. D. Beyene, R. Jantti, O. Tirkkonen, K. Ruttik, S. Iraji et al., “NB-IoT technology overview and experience from cloud-ran implementation,” IEEE Wireless Communication, vol. 24, no. 3, pp. 26–32, 2017. [Google Scholar]
73. A. Checko, H. L. Christiansen, Y. Yan, L. Scolari, G. Kardaras et al., “Cloud RAN for mobile networks—A technology overview,” IEEE Communications Surveys and Tutorials, vol. 17, no. 1, pp. 405–426, 2015. [Google Scholar]
74. T. Quek, M. Peng, O. Simeone and W. Yu, Cloud Radio Access Networks. Cambridge UK: Cambridge University Press, 2017. [Google Scholar]
75. Y. D. Beyene, R. Jantti and K. Ruttik, “Cloud-ran architecture for indoor das,” IEEE Access, vol. 2, pp. 12–1205, 2014. [Google Scholar]
76. V. Liu, A. Parks, V. Talla, S. Gollakota, D. Wetherall et al., “Ambient backscatter: Wireless communication out of thin air,” in Proc. ACM SIGCOM, Hong Kong, pp. 39–50, 2013. [Google Scholar]
77. D. Darsena, G. Gelli and F. Verde, “Cloud-aided cognitive ambient backscatter wireless sensor networks,” IEEE Access, vol. 7, pp. 57399–57414, 2019. [Google Scholar]
78. M. P. Singh and P. Kumar, “An efficient forward error correction scheme for wireless sensor network,” Procedia Technology, vol. 4, pp. 737–742, 2012. [Google Scholar]
79. F. Bouabdallah, N. Bouabdallah and R. Boutaba, “On balancing energy consumption in wireless sensor networks,” IEEE Transaction on Vehicular Technology, vol. 58, no. 6, pp. 2909–2924, 2009. [Google Scholar]
80. F. Walther, “Energy modeling: A low power wireless sensor node,” Technical Report, University of Kaiserslautern, 2006. [Online]. Available: http://www.eit.unkl.de/wehn/files/reports/micazpowermodel.pdf [Google Scholar]
81. M. E. Migabo, K. Djouani, A. M. Kurien and T. O. Olwal, “A stochastic energy consumption model for wireless sensor networks using gbr techniques,” in Proc. AFRICON, Addis Ababa, Ethiopia, pp. 1–5, 2015. [Google Scholar]
82. J. Finnegan and S. Brown, “A comparative survey of LPWA networking,” 2018. [Online]. Available: https://www.researchgate.net/publication/323141782 [Google Scholar]
83. S. Hassan, S. Ashraf, A. Sameera, B. Yasmeen and B. Nadeem, “Review on smart electric metering system based on gsm/iot,” Asian Journal of Electrical Sciences, vol. 8, no. 1, pp. 1–6, 2019. [Google Scholar]
84. A. R. Hambley, “Electrical engineering: Principles and applications,” in Upper Saddle River, 7th ed., NJ, USA: Pearson, 2008. [Google Scholar]
85. P. Umang and M. Mitul, “A review on smart meter system,” International Journal of Innovative Research in Electrical, Electronics, Instrumentation and Control Engineering, vol. 3, no. 12, pp. 2321–5526, 2015. [Google Scholar]
86. TOI, “Revamped Distribution Sector Scheme (RDSS)—The steppingstone to the energy transition,” 2021, IST in voices, India. [Online]. Available: https://timesofindia.indiatimes.com/blogs/voices/revamped-distribution-sector-scheme-rdss-the-stepping-stone-to-the-energy-transition/ [Google Scholar]
87. Q. Sun, H. Li, Z. Ma, C. Wang, J. Campillo et al., “A comprehensive review of smart energy meters in intelligent energy networks,” IEEE Internet of Things, vol. 3, no. 4, pp. 464–479, 2015. [Google Scholar]
88. Smart Energy International, “India’s smart meter rollout—250 million meters by 2025,” 2021. [Online]. Available: https://www.smart-energy.com/magazinearticle/indias-smart-meter-rollout-250-million-meters-by-2025/ [Google Scholar]
89. Enterprise IoT Insight, “Over 572m smart electricity meters to be installed in apac in 2021-25 (enterpriseiotinsights.com),” 2021. [Online]. Available: https://enterpriseiotinsights.com/20210308/energy/over-572-smartelectricity-meters-installed-apac-2021-25 [Google Scholar]
90. S. Mondal and A. K. Bohre, “Planning and control techniques of smart grids,” in Proc. ICEPES, Bhopal, India, pp. 1–6, 2021. [Google Scholar]
91. M. Massaoudi, H. Abu-Rub, S. S. Refaat, I. Chihi and F. S. Oueslati, “Accurate smart-grid stability forecasting based on deep learning: Point and interval estimation method,” in Proc. KPEC, Manhattan, KS, USA, pp. 1–6, 2021. [Google Scholar]
92. T. A. Alghamdi and N. Javaid, “A survey of preprocessing methods used for analysis of big data originated from smart grids,” IEEE Access, vol. 10, pp. 29149–29171, 2022. [Google Scholar]
93. S. Das, P. Kankanala and A. Pahwa, “Outage estimation in electric power distribution systems using a neural network ensemble,” Energies, vol. 14, no. 16, pp. 4797, 2021. [Google Scholar]
94. Y. Zhang, X. Shi, H. Zhang, Y. Cao and V. Terzija, “Review on deep learning applications in frequency analysis and control of modern power system,” International Journal of Electrical Power & Energy Systems, vol. 136, no. 107744, pp. 1–18, 2022. [Google Scholar]
95. W. Abdullah, M. Osman, A. B. Kadir and R. Verayiah, “The potential and status of renewable energy development in Malaysia,” Energies, vol. 12, no. 12, pp. 2437, 2019. [Google Scholar]
96. K. Sharma, A. Malik and I. Batra, “An AI based framework for energy efficiency in smart homes,” Neuroquantology, vol. 20, no. 7, pp. 2733–2743, 2022. [Google Scholar]
97. Y. Wang, F. Luo, Z. Dong, Z. Tong and Y. Giao, “Distributed meter data aggregation framework based on blockchain and homomorphic encryption,” IET Cyber-Physical Systems: Theory & Applications, vol. 4, no. 1, pp. 30–37, 2019. [Google Scholar]
98. E. Queen, “Smart meters and smart meter systems: A metering industry perspective,” White Paper, 2011, Washington, USA. [Online]. Available: https://www.cpsenergy.com/content/dam/corporate/en/Documents/SmartGrid/A%20Metering%20Industry%20Perspective.pdf [Google Scholar]
99. C. Logic, “Single-phase bi-directional power/energy,” 2021. [Online]. Available: https://www.cirrus.com/products/cs5460a/ [Google Scholar]
100. South African Micro-Electronic Systems, datasheet number: sa9602hpa, 1998. [Online]. Available: https://www.digchip.com/datasheets/. [Google Scholar]
101. India smart-grid forum, “AMI roll-out plan for India: India smart grid week 2017,” 2017. [Online]. Available: https://www.indiasmartgrid.org/ [Google Scholar]
102. L. Sun, K. Zhou, X. Zhang and S. Yang, “Outlier data treatment methods toward smart grid applications,” IEEE Access, vol. 6, pp. 39849–39859, 2018. [Google Scholar]
103. P. Sakthivel and S. Ganeshkumaran, “Design of automatic power consumption control system using smart grid—A review,” in Proc. WCFTRISW, Coimbatore, India, pp. 1–4, 2016. [Google Scholar]
104. S. Bera, S. Misra and M. S. Obaidat, “Energy-efficient smart metering for green smart grid communication,” in Proc. of the 2014 IEEE Global Communications Conf., Austin, TX, USA, pp. 2466–2471, 2014. [Google Scholar]
105. M. Alazab, S. Khan, S. S. R. Krishnan, Q. V. Pham, M. P. K. Reddy et al., “A multidirectional LSTM model for predicting the stability of a smart grid,” IEEE Access, vol. 8, pp. 85454–85463, 2020. [Google Scholar]
106. Q. V. Pham, M. Liyanage, N. Deepa, M. Reddy, S. Maddikunta et al., “Deep learning for intelligent demand response and smart grids: A comprehensive survey,” arXiv preprint, vol. 2101-08013, pp. 1–25, 2021. [Google Scholar]
107. J. J. M. Escobar, O. M. Matamoros, R. T. Padilla, I. L. Reyes and H. Q. Espinosa, “A comprehensive review on smart grids: Challenges and opportunities,” Sensors, vol. 21, no. 21, pp. 6978, 2021. [Google Scholar]
108. L. Lv, Z. Wu, J. Zhang, L. Zhang, Z. Tan et al., “A VMD and LSTM based hybrid model of load forecasting for power grid security,” IEEE Transactions on Industrial Informatics, vol. 18, no. 9, pp. 6474–6482, 2022. [Google Scholar]
109. S. Li, Y. Zhong and J. Lin, “AWS-DAIE: Incremental ensemble short-term electricity load forecasting based on sample domain adaptation,” Sustainability, vol. 14, no. 21, pp. 14205, 2022. [Google Scholar]
110. J. Bi, H. Ma, H. Yuan, K. Xu and M. Zhou, “Adaptive prediction of resources and workloads for cloud computing systems with attention-based and hybrid LSTM,” in Proc. IEEE ICSMC, Prague, Czech Republic, pp. 550–555, 2022. [Google Scholar]
Cite This Article
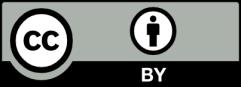