Open Access
ARTICLE
Decentralized Heterogeneous Federal Distillation Learning Based on Blockchain
Nanjing Power Supply Branch, State Grid Jiangsu Electric Power Co., Ltd., Nanjing, 210000, China
* Corresponding Author: Hong Zhu. Email:
Computers, Materials & Continua 2023, 76(3), 3363-3377. https://doi.org/10.32604/cmc.2023.040731
Received 29 March 2023; Accepted 13 June 2023; Issue published 08 October 2023
Abstract
Load forecasting is a crucial aspect of intelligent Virtual Power Plant (VPP) management and a means of balancing the relationship between distributed power grids and traditional power grids. However, due to the continuous emergence of power consumption peaks, the power supply quality of the power grid cannot be guaranteed. Therefore, an intelligent calculation method is required to effectively predict the load, enabling better power grid dispatching and ensuring the stable operation of the power grid. This paper proposes a decentralized heterogeneous federated distillation learning algorithm (DHFDL) to promote trusted federated learning (FL) between different federates in the blockchain. The algorithm comprises two stages: common knowledge accumulation and personalized training. In the first stage, each federate on the blockchain is treated as a meta-distribution. After aggregating the knowledge of each federate circularly, the model is uploaded to the blockchain. In the second stage, other federates on the blockchain download the trained model for personalized training, both of which are based on knowledge distillation. Experimental results demonstrate that the DHFDL algorithm proposed in this paper can resist a higher proportion of malicious code compared to FedAvg and a Blockchain-based Federated Learning framework with Committee consensus (BFLC). Additionally, by combining asynchronous consensus with the FL model training process, the DHFDL training time is the shortest, and the training efficiency of decentralized FL is improved.Keywords
Cite This Article
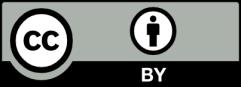