Open Access
ARTICLE
Hybrid Malware Variant Detection Model with Extreme Gradient Boosting and Artificial Neural Network Classifiers
1 Department of Computer Science, Northern Border University, Arar, 9280, Saudi Arabia
2 Department of Computer Sciences, Faculty of Computing and Information Technology, Northern Border University, Rafha, 91911, Saudi Arabia
3 Department of Information Systems, College of Computer Science and Information, Jouf University, Sakaka, Aljouf, Saudi Arabia
4 School of Computing, University Teknologi Malaysia, 81310 UTM Johor Bahru, Johor, 81310, Malaysia
5 Department of Computer and Electronic Engineering, Sana’a Community College, Sana’a, 5695, Yemen
* Corresponding Author: Abdulbasit A. Darem. Email:
Computers, Materials & Continua 2023, 76(3), 3483-3498. https://doi.org/10.32604/cmc.2023.041038
Received 08 April 2023; Accepted 01 July 2023; Issue published 08 October 2023
Abstract
In an era marked by escalating cybersecurity threats, our study addresses the challenge of malware variant detection, a significant concern for a multitude of sectors including petroleum and mining organizations. This paper presents an innovative Application Programmable Interface (API)-based hybrid model designed to enhance the detection performance of malware variants. This model integrates eXtreme Gradient Boosting (XGBoost) and an Artificial Neural Network (ANN) classifier, offering a potent response to the sophisticated evasion and obfuscation techniques frequently deployed by malware authors. The model’s design capitalizes on the benefits of both static and dynamic analysis to extract API-based features, providing a holistic and comprehensive view of malware behavior. From these features, we construct two XGBoost predictors, each of which contributes a valuable perspective on the malicious activities under scrutiny. The outputs of these predictors, interpreted as malicious scores, are then fed into an ANN-based classifier, which processes this data to derive a final decision. The strength of the proposed model lies in its capacity to leverage behavioral and signature-based features, and most importantly, in its ability to extract and analyze the hidden relations between these two types of features. The efficacy of our proposed API-based hybrid model is evident in its performance metrics. It outperformed other models in our tests, achieving an impressive accuracy of 95% and an F-measure of 93%. This significantly improved the detection performance of malware variants, underscoring the value and potential of our approach in the challenging field of cybersecurity.Keywords
Cite This Article
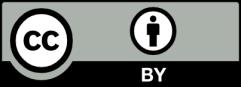