Open Access
ARTICLE
Multi-Layer Deep Sparse Representation for Biological Slice Image Inpainting
1 College of Information and Electrical Engineering, China Agricultural University, Beijing, 100000, China
2 Yantai Research Institute, China Agricultural University, Yantai, 264670, China
* Corresponding Author: Shuli Mei. Email:
Computers, Materials & Continua 2023, 76(3), 3813-3832. https://doi.org/10.32604/cmc.2023.041416
Received 21 April 2023; Accepted 29 July 2023; Issue published 08 October 2023
Abstract
Biological slices are an effective tool for studying the physiological structure and evolution mechanism of biological systems. However, due to the complexity of preparation technology and the presence of many uncontrollable factors during the preparation processing, leads to problems such as difficulty in preparing slice images and breakage of slice images. Therefore, we proposed a biological slice image small-scale corruption inpainting algorithm with interpretability based on multi-layer deep sparse representation, achieving the high-fidelity reconstruction of slice images. We further discussed the relationship between deep convolutional neural networks and sparse representation, ensuring the high-fidelity characteristic of the algorithm first. A novel deep wavelet dictionary is proposed that can better obtain image prior and possess learnable feature. And multi-layer deep sparse representation is used to implement dictionary learning, acquiring better signal expression. Compared with methods such as NLABH, Shearlet, Partial Differential Equation (PDE), K-Singular Value Decomposition (K-SVD), Convolutional Sparse Coding, and Deep Image Prior, the proposed algorithm has better subjective reconstruction and objective evaluation with small-scale image data, which realized high-fidelity inpainting, under the condition of small-scale image data. And the -level time complexity makes the proposed algorithm practical. The proposed algorithm can be effectively extended to other cross-sectional image inpainting problems, such as magnetic resonance images, and computed tomography images.Keywords
Cite This Article
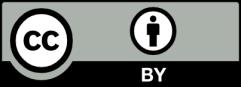