Open Access
ARTICLE
A Lightweight Deep Learning-Based Model for Tomato Leaf Disease Classification
1 Department of Software Engineering, University of Engineering and Technology, Taxila, 4400, Pakistan
2 Department of Computer Science, Faculty of Physics, Engineering, and Computer Science, University of Hertfordshire, Hatfield, AL10 9AB, UK
3 Department of Computer Science, College of Computer Science and Information System, Najran University, Najran, 55461, Saudi Arabia
4 Departments of Information Systems, College of Computer Science and Information Systems, Najran University, Najran, 61441, Saudi Arabia
5 Department of Computer Sciences, College of Computer and Information Sciences, Princess Nourah bint Abdulrahman University, Riyadh, 11671, Saudi Arabia
* Corresponding Authors: Javed Ali Khan. Email: ; Eman Abdullah Aldakheel. Email:
(This article belongs to the Special Issue: Optimization for Artificial Intelligence Application)
Computers, Materials & Continua 2023, 77(3), 3969-3992. https://doi.org/10.32604/cmc.2023.041819
Received 08 May 2023; Accepted 30 October 2023; Issue published 26 December 2023
Abstract
Tomato leaf diseases significantly impact crop production, necessitating early detection for sustainable farming. Deep Learning (DL) has recently shown excellent results in identifying and classifying tomato leaf diseases. However, current DL methods often require substantial computational resources, hindering their application on resource-constrained devices. We propose the Deep Tomato Detection Network (DTomatoDNet), a lightweight DL-based framework comprising 19 learnable layers for efficient tomato leaf disease classification to overcome this. The Convn kernels used in the proposed (DTomatoDNet) framework is 1 × 1, which reduces the number of parameters and helps in more detailed and descriptive feature extraction for classification. The proposed DTomatoDNet model is trained from scratch to determine the classification success rate. 10,000 tomato leaf images (1000 images per class) from the publicly accessible dataset, covering one healthy category and nine disease categories, are utilized in training the proposed DTomatoDNet approach. More specifically, we classified tomato leaf images into Target Spot (TS), Early Blight (EB), Late Blight (LB), Bacterial Spot (BS), Leaf Mold (LM), Tomato Yellow Leaf Curl Virus (YLCV), Septoria Leaf Spot (SLS), Spider Mites (SM), Tomato Mosaic Virus (MV), and Tomato Healthy (H). The proposed DTomatoDNet approach obtains a classification accuracy of 99.34%, demonstrating excellent accuracy in differentiating between tomato diseases. The model could be used on mobile platforms because it is lightweight and designed with fewer layers. Tomato farmers can utilize the proposed DTomatoDNet methodology to detect disease more quickly and easily once it has been integrated into mobile platforms by developing a mobile application.Keywords
Cite This Article
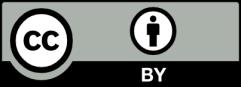