Open Access
ARTICLE
Functional Pattern-Related Anomaly Detection Approach Collaborating Binary Segmentation with Finite State Machine
1 School of Information, Liaoning University, Shenyang, 110036, China
2 School of Computer Science and Technology, Hainan University, Haikou, 570228, China
3 School of Physics, Liaoning University, Shenyang, 110036, China
* Corresponding Author: Jiangyuan Yao. Email:
(This article belongs to the Special Issue: Advanced Data Mining Techniques: Security, Intelligent Systems and Applications)
Computers, Materials & Continua 2023, 77(3), 3573-3592. https://doi.org/10.32604/cmc.2023.044857
Received 10 August 2023; Accepted 08 November 2023; Issue published 26 December 2023
Abstract
The process control-oriented threat, which can exploit OT (Operational Technology) vulnerabilities to forcibly insert abnormal control commands or status information, has become one of the most devastating cyber attacks in industrial automation control. To effectively detect this threat, this paper proposes one functional pattern-related anomaly detection approach, which skillfully collaborates the BinSeg (Binary Segmentation) algorithm with FSM (Finite State Machine) to identify anomalies between measuring data and control data. By detecting the change points of measuring data, the BinSeg algorithm is introduced to generate some initial sequence segments, which can be further classified and merged into different functional patterns due to their backward difference means and lengths. After analyzing the pattern association according to the Bayesian network, one functional state transition model based on FSM, which accurately describes the whole control and monitoring process, is constructed as one feasible detection engine. Finally, we use the typical SWaT (Secure Water Treatment) dataset to evaluate the proposed approach, and the experimental results show that: for one thing, compared with other change-point detection approaches, the BinSeg algorithm can be more suitable for the optimal sequence segmentation of measuring data due to its highest detection accuracy and least consuming time; for another, the proposed approach exhibits relatively excellent detection ability, because the average detection precision, recall rate and F1-score to identify 10 different attacks can reach 0.872, 0.982 and 0.896, respectively.Keywords
Cite This Article
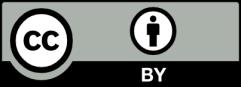