Open Access
ARTICLE
Machine Learning for Data Fusion: A Fuzzy AHP Approach for Open Issues
1 Chitkara University Institute of Engineering and Technology, Chitkara University, Punjab, 140401, India
2 Department of Networking and Communications, SRM Institute of Science and Technology, Kattankulathur, Chengalpattu, Tamil Nadu, 603203, India
3 Department of Networking and Communications, Faculty of Engineering and Technology, SRM Institute of Science and Technology, Kattankulathur, Chengalpattu, Tamil Nadu, 603203, India
4 Department of Computer Science and Engineering, Vardhaman College of Engineering, Hyderabad, 501218, India
5 Department of Computer Science and Information Engineering, National Yunlin University of Science and Technology, Yunlin, 64002, Taiwan
6 Intelligence Recognition Industry Service Research Center, National Yunlin University of Science and Technology, Yunlin, 64002, Taiwan
* Corresponding Author: Shih-Yu Chen. Email:
Computers, Materials & Continua 2023, 77(3), 2899-2914. https://doi.org/10.32604/cmc.2023.045136
Received 18 August 2023; Accepted 18 October 2023; Issue published 26 December 2023
Abstract
Data fusion generates fused data by combining multiple sources, resulting in information that is more consistent, accurate, and useful than any individual source and more reliable and consistent than the raw original data, which are often imperfect, inconsistent, complex, and uncertain. Traditional data fusion methods like probabilistic fusion, set-based fusion, and evidential belief reasoning fusion methods are computationally complex and require accurate classification and proper handling of raw data. Data fusion is the process of integrating multiple data sources. Data filtering means examining a dataset to exclude, rearrange, or apportion data according to the criteria. Different sensors generate a large amount of data, requiring the development of machine learning (ML) algorithms to overcome the challenges of traditional methods. The advancement in hardware acceleration and the abundance of data from various sensors have led to the development of machine learning (ML) algorithms, expected to address the limitations of traditional methods. However, many open issues still exist as machine learning algorithms are used for data fusion. From the literature, nine issues have been identified irrespective of any application. The decision-makers should pay attention to these issues as data fusion becomes more applicable and successful. A fuzzy analytical hierarchical process (FAHP) enables us to handle these issues. It helps to get the weights for each corresponding issue and rank issues based on these calculated weights. The most significant issue identified is the lack of deep learning models used for data fusion that improve accuracy and learning quality weighted 0.141. The least significant one is the cross-domain multimodal data fusion weighted 0.076 because the whole semantic knowledge for multimodal data cannot be captured.Keywords
Cite This Article
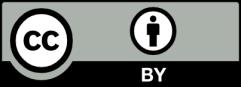