Open Access
ARTICLE
Smart Healthcare Activity Recognition Using Statistical Regression and Intelligent Learning
1 Department of Computer Sciences, Government Arts College (Autonomous), Salem, 636007, India
2 Department of Computer Sciences, College of Computer and Information Sciences, Princess Nourah Bint Abdulrahman University, Riyadh, 11671, Saudi Arabia
3 Department of Information Systems, College of Computer and Information Sciences, Princess Nourah Bint Abdulrahman University, Riyadh, 11671, Saudi Arabia
* Corresponding Author: Nithya Rekha Sivakumar. Email:
Computers, Materials & Continua 2024, 78(1), 1189-1205. https://doi.org/10.32604/cmc.2023.034815
Received 28 July 2022; Accepted 30 June 2023; Issue published 30 January 2024
Abstract
In this present time, Human Activity Recognition (HAR) has been of considerable aid in the case of health monitoring and recovery. The exploitation of machine learning with an intelligent agent in the area of health informatics gathered using HAR augments the decision-making quality and significance. Although many research works conducted on Smart Healthcare Monitoring, there remain a certain number of pitfalls such as time, overhead, and falsification involved during analysis. Therefore, this paper proposes a Statistical Partial Regression and Support Vector Intelligent Agent Learning (SPR-SVIAL) for Smart Healthcare Monitoring. At first, the Statistical Partial Regression Feature Extraction model is used for data preprocessing along with the dimensionality-reduced features extraction process. Here, the input dataset the continuous beat-to-beat heart data, triaxial accelerometer data, and psychological characteristics were acquired from IoT wearable devices. To attain highly accurate Smart Healthcare Monitoring with less time, Partial Least Square helps extract the dimensionality-reduced features. After that, with these resulting features, SVIAL is proposed for Smart Healthcare Monitoring with the help of Machine Learning and Intelligent Agents to minimize both analysis falsification and overhead. Experimental evaluation is carried out for factors such as time, overhead, and false positive rate accuracy concerning several instances. The quantitatively analyzed results indicate the better performance of our proposed SPR-SVIAL method when compared with two state-of-the-art methods.Keywords
Cite This Article
K. Akilandeswari, N. R. Sivakumar, H. K. Alkahtani, S. Basheer and S. A. Ghorashi, "Smart healthcare activity recognition using statistical regression and intelligent learning," Computers, Materials & Continua, vol. 78, no.1, pp. 1189–1205, 2024. https://doi.org/10.32604/cmc.2023.034815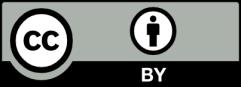