Open Access
ARTICLE
Spatiotemporal Prediction of Urban Traffics Based on Deep GNN
1 School of Computer Science, Hangzhou Dianzi University, Hangzhou, 310018, China
2 Zhejiang Institute of Communications, Hangzhou, 310012, China
3 School of Cyberspace Security, Hangzhou Dianzi University, Hangzhou, 310018, China
* Corresponding Author: Ning Zheng. Email:
(This article belongs to the Special Issue: Big Data Analysis for Healthcare Applications)
Computers, Materials & Continua 2024, 78(1), 265-282. https://doi.org/10.32604/cmc.2023.040067
Received 03 March 2023; Accepted 13 September 2023; Issue published 30 January 2024
Abstract
Traffic prediction already plays a significant role in applications like traffic planning and urban management, but it is still difficult to capture the highly non-linear and complicated spatiotemporal correlations of traffic data. As well as to fulfil both long-term and short-term prediction objectives, a better representation of the temporal dependency and global spatial correlation of traffic data is needed. In order to do this, the Spatiotemporal Graph Neural Network (S-GNN) is proposed in this research as a method for traffic prediction. The S-GNN simultaneously accepts various traffic data as inputs and investigates the non-linear correlations between the variables. In terms of modelling, the road network is initially represented as a spatiotemporal directed graph, with the features of the samples at the time step being captured by a convolution module. In order to assign varying attention weights to various adjacent area nodes of the target node, the adjacent areas information of nodes in the road network is then aggregated using a graph network. The data is output using a fully connected layer at the end. The findings show that S-GNN can improve short- and long-term traffic prediction accuracy to a greater extent; in comparison to the control model, the RMSE of S-GNN is reduced by about 0.571 to 9.288 and the MAE (Mean Absolute Error) by about 0.314 to 7.678. The experimental results on two real datasets, Pe MSD7(M) and PEMS-BAY, also support this claim.Keywords
Cite This Article
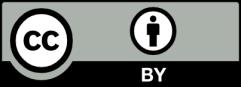