Open Access
ARTICLE
Human Gait Recognition for Biometrics Application Based on Deep Learning Fusion Assisted Framework
1 Department of Electrical Engineering, HITEC University, Taxila, 47080, Pakistan
2 Department of Computer Science and Mathematics, Lebanese American University, Beirut, Lebanon
3 Department of Computer Science, HITEC University, Taxila, 47080, Pakistan
4 Department of Information Systems, College of Computer and Information Sciences, Princess Nourah bint Abdulrahman University, P.O. Box 84428, Riyadh, 11671, Saudi Arabia
5 Department of Computer Science, Hanyang University, Seoul, 04763, Korea
* Corresponding Author: Muhammad Attique Khan. Email:
Computers, Materials & Continua 2024, 78(1), 357-374. https://doi.org/10.32604/cmc.2023.043061
Received 20 June 2023; Accepted 07 November 2023; Issue published 30 January 2024
Abstract
The demand for a non-contact biometric approach for candidate identification has grown over the past ten years. Based on the most important biometric application, human gait analysis is a significant research topic in computer vision. Researchers have paid a lot of attention to gait recognition, specifically the identification of people based on their walking patterns, due to its potential to correctly identify people far away. Gait recognition systems have been used in a variety of applications, including security, medical examinations, identity management, and access control. These systems require a complex combination of technical, operational, and definitional considerations. The employment of gait recognition techniques and technologies has produced a number of beneficial and well-liked applications. This work proposes a novel deep learning-based framework for human gait classification in video sequences. This framework’s main challenge is improving the accuracy of accuracy gait classification under varying conditions, such as carrying a bag and changing clothes. The proposed method’s first step is selecting two pre-trained deep learning models and training from scratch using deep transfer learning. Next, deep models have been trained using static hyperparameters; however, the learning rate is calculated using the particle swarm optimization (PSO) algorithm. Then, the best features are selected from both trained models using the Harris Hawks controlled Sine-Cosine optimization algorithm. This algorithm chooses the best features, combined in a novel correlation-based fusion technique. Finally, the fused best features are categorized using medium, bi-layer, and tri-layered neural networks. On the publicly accessible dataset known as the CASIA-B dataset, the experimental process of the suggested technique was carried out, and an improved accuracy of 94.14% was achieved. The achieved accuracy of the proposed method is improved by the recent state-of-the-art techniques that show the significance of this work.Keywords
Cite This Article
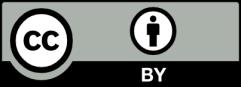