Open Access
ARTICLE
Using MsfNet to Predict the ISUP Grade of Renal Clear Cell Carcinoma in Digital Pathology Images
1 College of Quality and Technical Supervision, Hebei University, Baoding, 071002, China
2 National and Local Joint Engineering Research Center of Metrology Instrument and System, Hebei University, Baoding, 071002, China
3 Hebei Technology Innovation Center for Lightweight of New Energy Vehicle Power System, Hebei University, Baoding, 071002, China
* Corresponding Author: Linyan Xue. Email:
(This article belongs to the Special Issue: Deep Learning in Medical Imaging-Disease Segmentation and Classification)
Computers, Materials & Continua 2024, 78(1), 393-410. https://doi.org/10.32604/cmc.2023.044994
Received 14 August 2023; Accepted 14 November 2023; Issue published 30 January 2024
Abstract
Clear cell renal cell carcinoma (ccRCC) represents the most frequent form of renal cell carcinoma (RCC), and accurate International Society of Urological Pathology (ISUP) grading is crucial for prognosis and treatment selection. This study presents a new deep network called Multi-scale Fusion Network (MsfNet), which aims to enhance the automatic ISUP grade of ccRCC with digital histopathology pathology images. The MsfNet overcomes the limitations of traditional ResNet50 by multi-scale information fusion and dynamic allocation of channel quantity. The model was trained and tested using 90 Hematoxylin and Eosin (H&E) stained whole slide images (WSIs), which were all cropped into 320 × 320-pixel patches at 40× magnification. MsfNet achieved a micro-averaged area under the curve (AUC) of 0.9807, a macro-averaged AUC of 0.9778 on the test dataset. The Gradient-weighted Class Activation Mapping (Grad-CAM) visually demonstrated MsfNet’s ability to distinguish and highlight abnormal areas more effectively than ResNet50. The t-Distributed Stochastic Neighbor Embedding (t-SNE) plot indicates our model can efficiently extract critical features from images, reducing the impact of noise and redundant information. The results suggest that MsfNet offers an accurate ISUP grade of ccRCC in digital images, emphasizing the potential of AI-assisted histopathological systems in clinical practice.Keywords
Cite This Article
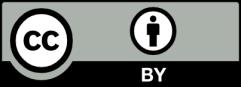