Open Access
ARTICLE
Deep Convolutional Neural Networks for Accurate Classification of Gastrointestinal Tract Syndromes
1 Department of Computer Science, COMSATS University Islamabad, Wah Campus, Wah, 47040, Pakistan
2 Department of Computer Science and Mathematics, Lebanese American University, Beirut, 13-5053, Lebanon
3 Department of Computer Science, HITEC University, Taxila, 47080, Pakistan
4 Department of Computer Science, COMSATS University Islamabad, Attock Campus, Attock, 43600, Pakistan
5 Department of Computer Science, Hanyang University, Seoul, 04763, Korea
* Corresponding Author: Muhammad Ramzan. Email:
(This article belongs to the Special Issue: Deep Learning in Medical Imaging-Disease Segmentation and Classification)
Computers, Materials & Continua 2024, 78(1), 1207-1225. https://doi.org/10.32604/cmc.2023.045491
Received 29 August 2023; Accepted 22 November 2023; Issue published 30 January 2024
Abstract
Accurate detection and classification of artifacts within the gastrointestinal (GI) tract frames remain a significant challenge in medical image processing. Medical science combined with artificial intelligence is advancing to automate the diagnosis and treatment of numerous diseases. Key to this is the development of robust algorithms for image classification and detection, crucial in designing sophisticated systems for diagnosis and treatment. This study makes a small contribution to endoscopic image classification. The proposed approach involves multiple operations, including extracting deep features from endoscopy images using pre-trained neural networks such as Darknet-53 and Xception. Additionally, feature optimization utilizes the binary dragonfly algorithm (BDA), with the fusion of the obtained feature vectors. The fused feature set is input into the ensemble subspace k nearest neighbors (ESKNN) classifier. The Kvasir-V2 benchmark dataset, and the COMSATS University Islamabad (CUI) Wah private dataset, featuring three classes of endoscopic stomach images were used. Performance assessments considered various feature selection techniques, including genetic algorithm (GA), particle swarm optimization (PSO), salp swarm algorithm (SSA), sine cosine algorithm (SCA), and grey wolf optimizer (GWO). The proposed model excels, achieving an overall classification accuracy of 98.25% on the Kvasir-V2 benchmark and 99.90% on the CUI Wah private dataset. This approach holds promise for developing an automated computer-aided system for classifying GI tract syndromes through endoscopy images.Keywords
Cite This Article
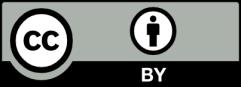