Open Access
ARTICLE
An Improved Solov2 Based on Attention Mechanism and Weighted Loss Function for Electrical Equipment Instance Segmentation
1 Key Laboratory of Modern Power System Simulation and Control & Renewable Energy Technology, Ministry of Education, Northeast Electric Power University, Jilin, 132012, China
2 School of Electrical Engineering, Northeast Electric Power University, Jilin, 132012, China
3 Baishan Power Supply Company, State Grid Jilin Electric Power Co, Ltd., Jilin, 134300, China
* Corresponding Author: Junpeng Wu. Email:
(This article belongs to the Special Issue: Advanced Artificial Intelligence and Machine Learning Frameworks for Signal and Image Processing Applications)
Computers, Materials & Continua 2024, 78(1), 677-694. https://doi.org/10.32604/cmc.2023.045759
Received 06 September 2023; Accepted 19 November 2023; Issue published 30 January 2024
Abstract
The current existing problem of deep learning framework for the detection and segmentation of electrical equipment is dominantly related to low precision. Because of the reliable, safe and easy-to-operate technology provided by deep learning-based video surveillance for unmanned inspection of electrical equipment, this paper uses the bottleneck attention module (BAM) attention mechanism to improve the Solov2 model and proposes a new electrical equipment segmentation mode. Firstly, the BAM attention mechanism is integrated into the feature extraction network to adaptively learn the correlation between feature channels, thereby improving the expression ability of the feature map; secondly, the weighted sum of CrossEntropy Loss and Dice loss is designed as the mask loss to improve the segmentation accuracy and robustness of the model; finally, the non-maximal suppression (NMS) algorithm to better handle the overlap problem in instance segmentation. Experimental results show that the proposed method achieves an average segmentation accuracy of mAP of 80.4% on three types of electrical equipment datasets, including transformers, insulators and voltage transformers, which improve the detection accuracy by more than 5.7% compared with the original Solov2 model. The segmentation model proposed can provide a focusing technical means for the intelligent management of power systems.Keywords
Cite This Article
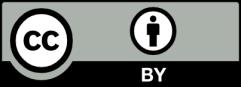