Open Access
ARTICLE
Printed Circuit Board (PCB) Surface Micro Defect Detection Model Based on Residual Network with Novel Attention Mechanism
School of Mechanical Engineering, Hubei University of Technology, Wuhan, 430068, Chain
* Corresponding Author: Defeng Kong. Email:
(This article belongs to the Special Issue: Machine Vision Detection and Intelligent Recognition)
Computers, Materials & Continua 2024, 78(1), 915-933. https://doi.org/10.32604/cmc.2023.046376
Received 28 September 2023; Accepted 23 November 2023; Issue published 30 January 2024
Abstract
Printed Circuit Board (PCB) surface tiny defect detection is a difficult task in the integrated circuit industry, especially since the detection of tiny defects on PCB boards with large-size complex circuits has become one of the bottlenecks. To improve the performance of PCB surface tiny defects detection, a PCB tiny defects detection model based on an improved attention residual network (YOLOX-AttResNet) is proposed. First, the unsupervised clustering performance of the K-means algorithm is exploited to optimize the channel weights for subsequent operations by feeding the feature mapping into the SENet (Squeeze and Excitation Network) attention network; then the improved K-means-SENet network is fused with the directly mapped edges of the traditional ResNet network to form an augmented residual network (AttResNet); and finally, the AttResNet module is substituted for the traditional ResNet structure in the backbone feature extraction network of mainstream excellent detection models, thus improving the ability to extract small features from the backbone of the target detection network. The results of ablation experiments on a PCB surface defect dataset show that AttResNet is a reliable and efficient module. In Torify the performance of AttResNet for detecting small defects in large-size complex circuit images, a series of comparison experiments are further performed. The results show that the AttResNet module combines well with the five best existing target detection frameworks (YOLOv3, YOLOX, Faster R-CNN, TDD-Net, Cascade R-CNN), and all the combined new models have improved detection accuracy compared to the original model, which suggests that the AttResNet module proposed in this paper can help the detection model to extract target features. Among them, the YOLOX-AttResNet model proposed in this paper performs the best, with the highest accuracy of 98.45% and the detection speed of 36 FPS (Frames Per Second), which meets the accuracy and real-time requirements for the detection of tiny defects on PCB surfaces. This study can provide some new ideas for other real-time online detection tasks of tiny targets with high-resolution images.Keywords
Cite This Article
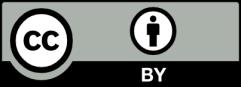