Open Access
ARTICLE
Selective and Adaptive Incremental Transfer Learning with Multiple Datasets for Machine Fault Diagnosis
1 School of Science and Technology, Hong Kong Metropolitan University, Hong Kong, 999077, China
2 Department of Computer Science and Information Engineering, Asia University, Taichung, 41354, Taiwan
3 Center for Advanced Information Technology, Kyung Hee University, Seoul, 02447, Korea
4 Symbiosis Centre for Information Technology, Symbiosis International University, Pune, Maharashtra, 411042, India
5 Department of Electrical and Computer Engineering, Lebanese American University, Beirut, 1102, Lebanon
6 School of Computing, Skyline University College, Sharjah, 1797, United Arab Emirates
7 Department of Business Administration, Asia University, Taichung, 41354, Taiwan
8 Center for Interdisciplinary Research, University of Petroleum and Energy Studies, Dehradun, 248007, India
9 Department of Computer Science & Engineering, Chandigarh University, Chandigarh, 160036, India
10 Centro de Investigación en Computación, Instituto Politécnico Nacional, UPALM-Zacatenco, Mexico City, 07320, Mexico
* Corresponding Authors: Kwok Tai Chui. Email: ; Brij B. Gupta. Email:
(This article belongs to the Special Issue: Development and Industrial Application of AI Technologies)
Computers, Materials & Continua 2024, 78(1), 1363-1379. https://doi.org/10.32604/cmc.2023.046762
Received 13 October 2023; Accepted 27 November 2023; Issue published 30 January 2024
Abstract
The visions of Industry 4.0 and 5.0 have reinforced the industrial environment. They have also made artificial intelligence incorporated as a major facilitator. Diagnosing machine faults has become a solid foundation for automatically recognizing machine failure, and thus timely maintenance can ensure safe operations. Transfer learning is a promising solution that can enhance the machine fault diagnosis model by borrowing pre-trained knowledge from the source model and applying it to the target model, which typically involves two datasets. In response to the availability of multiple datasets, this paper proposes using selective and adaptive incremental transfer learning (SA-ITL), which fuses three algorithms, namely, the hybrid selective algorithm, the transferability enhancement algorithm, and the incremental transfer learning algorithm. It is a selective algorithm that enables selecting and ordering appropriate datasets for transfer learning and selecting useful knowledge to avoid negative transfer. The algorithm also adaptively adjusts the portion of training data to balance the learning rate and training time. The proposed algorithm is evaluated and analyzed using ten benchmark datasets. Compared with other algorithms from existing works, SA-ITL improves the accuracy of all datasets. Ablation studies present the accuracy enhancements of the SA-ITL, including the hybrid selective algorithm (1.22%–3.82%), transferability enhancement algorithm (1.91%–4.15%), and incremental transfer learning algorithm (0.605%–2.68%). These also show the benefits of enhancing the target model with heterogeneous image datasets that widen the range of domain selection between source and target domains.Keywords
Cite This Article
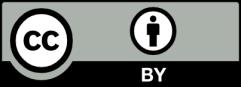