Open Access
ARTICLE
Real-Time Detection and Instance Segmentation of Strawberry in Unstructured Environment
1 School of Artificial Intelligence, Anhui University of Science and Technology, Huainan, 232001, China
2 School of Mechanical Engineering, Anhui University of Science and Technology, Huainan, 232001, China
* Corresponding Author: Fan Ding. Email:
(This article belongs to the Special Issue: Machine Vision Detection and Intelligent Recognition)
Computers, Materials & Continua 2024, 78(1), 1481-1501. https://doi.org/10.32604/cmc.2023.046876
Received 17 October 2023; Accepted 20 November 2023; Issue published 30 January 2024
Abstract
The real-time detection and instance segmentation of strawberries constitute fundamental components in the development of strawberry harvesting robots. Real-time identification of strawberries in an unstructured environment is a challenging task. Current instance segmentation algorithms for strawberries suffer from issues such as poor real-time performance and low accuracy. To this end, the present study proposes an Efficient YOLACT (E-YOLACT) algorithm for strawberry detection and segmentation based on the YOLACT framework. The key enhancements of the E-YOLACT encompass the development of a lightweight attention mechanism, pyramid squeeze shuffle attention (PSSA), for efficient feature extraction. Additionally, an attention-guided context-feature pyramid network (AC-FPN) is employed instead of FPN to optimize the architecture’s performance. Furthermore, a feature-enhanced model (FEM) is introduced to enhance the prediction head’s capabilities, while efficient fast non-maximum suppression (EF-NMS) is devised to improve non-maximum suppression. The experimental results demonstrate that the E-YOLACT achieves a Box-mAP and Mask-mAP of 77.9 and 76.6, respectively, on the custom dataset. Moreover, it exhibits an impressive category accuracy of 93.5%. Notably, the E-YOLACT also demonstrates a remarkable real-time detection capability with a speed of 34.8 FPS. The method proposed in this article presents an efficient approach for the vision system of a strawberry-picking robot.Keywords
Cite This Article
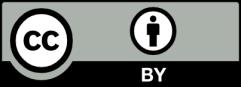