Open Access
ARTICLE
Depression Intensity Classification from Tweets Using FastText Based Weighted Soft Voting Ensemble
1 Department of Artificial Intelligence, The Islamia University of Bahawalpur, Bahawalnagar, 63100, Pakistan
2 Department of Information Technology, Khwaja Fareed University of Engineering and IT, RYKhan, 64200, Pakistan
3 Department of Computer Science & Information Technology, The Islamia University of Bahawalpur, Bahawalnagar, 63100, Pakistan
4 Department of Signal Theory and Communications and Telematic Engineering, Unviersity of Valladolid, Paseo de Belén 15, Valladolid, 47011, Spain
5 Faculty of Social Science and Humanities, Universidad Europea del Atlántico Isabel Torres 21, Santander, 39011, Spain
6 Department of Project Management, Universidad Internacional Iberoamericana, Campeche, 24560, México
7 Fundación Universitaria Internacional de Colombia, Bogotá, 111611, Colombia
8 Universidad Internacional Iberoamericana, Arecibo, Puerto Rico, 00613, USA
9 Universidade Internacional do Cuanza, Cuito, 46703, Angola
10 Department of Information and Communication Engineering, Yeungnam University, Gyeongsan, 38541, South Korea
* Corresponding Author: Imran Ashraf. Email:
Computers, Materials & Continua 2024, 78(2), 2047-2066. https://doi.org/10.32604/cmc.2024.037347
Received 31 October 2022; Accepted 11 August 2023; Issue published 27 February 2024
Abstract
Predicting depression intensity from microblogs and social media posts has numerous benefits and applications, including predicting early psychological disorders and stress in individuals or the general public. A major challenge in predicting depression using social media posts is that the existing studies do not focus on predicting the intensity of depression in social media texts but rather only perform the binary classification of depression and moreover noisy data makes it difficult to predict the true depression in the social media text. This study intends to begin by collecting relevant Tweets and generating a corpus of 210000 public tweets using Twitter public application programming interfaces (APIs). A strategy is devised to filter out only depression-related tweets by creating a list of relevant hashtags to reduce noise in the corpus. Furthermore, an algorithm is developed to annotate the data into three depression classes: ‘Mild,’ ‘Moderate,’ and ‘Severe,’ based on International Classification of Diseases-10 (ICD-10) depression diagnostic criteria. Different baseline classifiers are applied to the annotated dataset to get a preliminary idea of classification performance on the corpus. Further FastText-based model is applied and fine-tuned with different preprocessing techniques and hyperparameter tuning to produce the tuned model, which significantly increases the depression classification performance to an 84% F1 score and 90% accuracy compared to baselines. Finally, a FastText-based weighted soft voting ensemble (WSVE) is proposed to boost the model’s performance by combining several other classifiers and assigning weights to individual models according to their individual performances. The proposed WSVE outperformed all baselines as well as FastText alone, with an F1 of 89%, 5% higher than FastText alone, and an accuracy of 93%, 3% higher than FastText alone. The proposed model better captures the contextual features of the relatively small sample class and aids in the detection of early depression intensity prediction from tweets with impactful performances.Keywords
Cite This Article
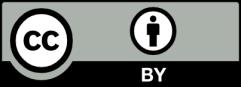