Open Access
ARTICLE
Multilevel Attention Unet Segmentation Algorithm for Lung Cancer Based on CT Images
1 Xi’an Institute of Optics and Precision Mechanics, Chinese Academy of Sciences, Xi’an, China
2 School of Biomedical Engineering, Fourth Military Medical University, Xi’an, China
3 University of Illinois at Urbana Champion, Champaign, USA
* Corresponding Author: Shi Qiu. Email:
(This article belongs to the Special Issue: Deep Learning in Computer-Aided Diagnosis Based on Medical Image)
Computers, Materials & Continua 2024, 78(2), 1569-1589. https://doi.org/10.32604/cmc.2023.046821
Received 16 October 2023; Accepted 07 December 2023; Issue published 27 February 2024
Abstract
Lung cancer is a malady of the lungs that gravely jeopardizes human health. Therefore, early detection and treatment are paramount for the preservation of human life. Lung computed tomography (CT) image sequences can explicitly delineate the pathological condition of the lungs. To meet the imperative for accurate diagnosis by physicians, expeditious segmentation of the region harboring lung cancer is of utmost significance. We utilize computer-aided methods to emulate the diagnostic process in which physicians concentrate on lung cancer in a sequential manner, erect an interpretable model, and attain segmentation of lung cancer. The specific advancements can be encapsulated as follows: 1) Concentration on the lung parenchyma region: Based on 16-bit CT image capturing and the luminance characteristics of lung cancer, we proffer an intercept histogram algorithm. 2) Focus on the specific locus of lung malignancy: Utilizing the spatial interrelation of lung cancer, we propose a memory-based Unet architecture and incorporate skip connections. 3) Data Imbalance: In accordance with the prevalent situation of an overabundance of negative samples and a paucity of positive samples, we scrutinize the existing loss function and suggest a mixed loss function. Experimental results with pre-existing publicly available datasets and assembled datasets demonstrate that the segmentation efficacy, measured as Area Overlap Measure (AOM) is superior to 0.81, which markedly ameliorates in comparison with conventional algorithms, thereby facilitating physicians in diagnosis.Keywords
Cite This Article
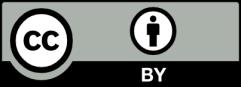