Open Access
ARTICLE
Attention Guided Multi Scale Feature Fusion Network for Automatic Prostate Segmentation
1 School of Information and Communication Engineering, Hainan University, Haikou, China
2 College of Computer Science and Technology, Hainan University, Haikou, China
3 Urology Department, Haikou Municipal People’s Hospital and Central South University Xiangya Medical College Affiliated Hospital, Haikou, China
4 School of Information Science and Technology, Hainan Normal University, Haikou, China
* Corresponding Author: Mengxing Huang. Email:
Computers, Materials & Continua 2024, 78(2), 1649-1668. https://doi.org/10.32604/cmc.2023.046883
Received 18 October 2023; Accepted 06 December 2023; Issue published 27 February 2024
Abstract
The precise and automatic segmentation of prostate magnetic resonance imaging (MRI) images is vital for assisting doctors in diagnosing prostate diseases. In recent years, many advanced methods have been applied to prostate segmentation, but due to the variability caused by prostate diseases, automatic segmentation of the prostate presents significant challenges. In this paper, we propose an attention-guided multi-scale feature fusion network (AGMSF-Net) to segment prostate MRI images. We propose an attention mechanism for extracting multi-scale features, and introduce a 3D transformer module to enhance global feature representation by adding it during the transition phase from encoder to decoder. In the decoder stage, a feature fusion module is proposed to obtain global context information. We evaluate our model on MRI images of the prostate acquired from a local hospital. The relative volume difference (RVD) and dice similarity coefficient (DSC) between the results of automatic prostate segmentation and ground truth were 1.21% and 93.68%, respectively. To quantitatively evaluate prostate volume on MRI, which is of significant clinical significance, we propose a unique AGMSF-Net. The essential performance evaluation and validation experiments have demonstrated the effectiveness of our method in automatic prostate segmentation.Keywords
Cite This Article
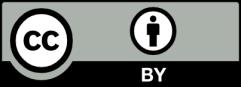