Open Access
ARTICLE
IR-YOLO: Real-Time Infrared Vehicle and Pedestrian Detection
1 Department of Electrical Engineering and Automation, Jiangxi University of Science and Technology, Ganzhou, 341000, China
2 Jiangxi Provincial Key Laboratory of Maglev Technology, Ganzhou, 341000, China
3 Graduate School of Information, Production and Systems, Waseda University, Kitakyushu-shi, Fukuoka, 808-0135, Japan
* Corresponding Author: Hao Zhu. Email:
(This article belongs to the Special Issue: Deep Learning based Object Detection and Tracking in Videos)
Computers, Materials & Continua 2024, 78(2), 2667-2687. https://doi.org/10.32604/cmc.2024.047988
Received 24 November 2023; Accepted 28 December 2023; Issue published 27 February 2024
Abstract
Road traffic safety can decrease when drivers drive in a low-visibility environment. The application of visual perception technology to detect vehicles and pedestrians in infrared images proves to be an effective means of reducing the risk of accidents. To tackle the challenges posed by the low recognition accuracy and the substantial computational burden associated with current infrared pedestrian-vehicle detection methods, an infrared pedestrian-vehicle detection method A proposal is presented, based on an enhanced version of You Only Look Once version 5 (YOLOv5). First, A head specifically designed for detecting small targets has been integrated into the model to make full use of shallow feature information to enhance the accuracy in detecting small targets. Second, the Focal Generalized Intersection over Union (GIoU) is employed as an alternative to the original loss function to address issues related to target overlap and category imbalance. Third, the distribution shift convolution optimization feature extraction operator is used to alleviate the computational burden of the model without significantly compromising detection accuracy. The test results of the improved algorithm show that its average accuracy (mAP) reaches 90.1%. Specifically, the Giga Floating Point Operations Per second (GFLOPs) of the improved algorithm is only 9.1. In contrast, the improved algorithms outperformed the other algorithms on similar GFLOPs, such as YOLOv6n (11.9), YOLOv8n (8.7), YOLOv7t (13.2) and YOLOv5s (16.0). The mAPs that are 4.4%, 3%, 3.5%, and 1.7% greater than those of these algorithms show that the improved algorithm achieves higher accuracy in target detection tasks under similar computational resource overhead. On the other hand, compared with other algorithms such as YOLOv8l (91.1%), YOLOv6l (89.5%), YOLOv7 (90.8%), and YOLOv3 (90.1%), the improved algorithm needs only 5.5%, 2.3%, 8.6%, and 2.3%, respectively, of the GFLOPs. The improved algorithm has shown significant advancements in balancing accuracy and computational efficiency, making it promising for practical use in resource-limited scenarios.Keywords
Cite This Article
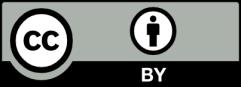