Open Access
ARTICLE
Missing Value Imputation for Radar-Derived Time-Series Tracks of Aerial Targets Based on Improved Self-Attention-Based Network
Department of Early Warning Intelligence, Early Warning Academy, Wuhan, 430019, China
* Corresponding Author: Yan Zhou. Email:
Computers, Materials & Continua 2024, 78(3), 3349-3376. https://doi.org/10.32604/cmc.2024.047034
Received 22 October 2023; Accepted 08 January 2024; Issue published 26 March 2024
Abstract
The frequent missing values in radar-derived time-series tracks of aerial targets (RTT-AT) lead to significant challenges in subsequent data-driven tasks. However, the majority of imputation research focuses on random missing (RM) that differs significantly from common missing patterns of RTT-AT. The method for solving the RM may experience performance degradation or failure when applied to RTT-AT imputation. Conventional autoregressive deep learning methods are prone to error accumulation and long-term dependency loss. In this paper, a non-autoregressive imputation model that addresses the issue of missing value imputation for two common missing patterns in RTT-AT is proposed. Our model consists of two probabilistic sparse diagonal masking self-attention (PSDMSA) units and a weight fusion unit. It learns missing values by combining the representations outputted by the two units, aiming to minimize the difference between the missing values and their actual values. The PSDMSA units effectively capture temporal dependencies and attribute correlations between time steps, improving imputation quality. The weight fusion unit automatically updates the weights of the output representations from the two units to obtain a more accurate final representation. The experimental results indicate that, despite varying missing rates in the two missing patterns, our model consistently outperforms other methods in imputation performance and exhibits a low frequency of deviations in estimates for specific missing entries. Compared to the state-of-the-art autoregressive deep learning imputation model Bidirectional Recurrent Imputation for Time Series (BRITS), our proposed model reduces mean absolute error (MAE) by 31%~50%. Additionally, the model attains a training speed that is 4 to 8 times faster when compared to both BRITS and a standard Transformer model when trained on the same dataset. Finally, the findings from the ablation experiments demonstrate that the PSDMSA, the weight fusion unit, cascade network design, and imputation loss enhance imputation performance and confirm the efficacy of our design.Keywords
Cite This Article
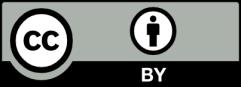