Open Access
ARTICLE
Predicting Traffic Flow Using Dynamic Spatial-Temporal Graph Convolution Networks
1 School of Computer and Data Science, Henan University of Urban Construction, Pingdingshan, 467036, China
2 School of Electrical and Control Engineering, Henan University of Urban Construction, Pingdingshan, 467036, China
* Corresponding Author: Yunchang Liu. Email:
Computers, Materials & Continua 2024, 78(3), 4343-4361. https://doi.org/10.32604/cmc.2024.047211
Received 29 October 2023; Accepted 07 February 2024; Issue published 26 March 2024
Abstract
Traffic flow prediction plays a key role in the construction of intelligent transportation system. However, due to its complex spatio-temporal dependence and its uncertainty, the research becomes very challenging. Most of the existing studies are based on graph neural networks that model traffic flow graphs and try to use fixed graph structure to deal with the relationship between nodes. However, due to the time-varying spatial correlation of the traffic network, there is no fixed node relationship, and these methods cannot effectively integrate the temporal and spatial features. This paper proposes a novel temporal-spatial dynamic graph convolutional network (TSADGCN). The dynamic time warping algorithm (DTW) is introduced to calculate the similarity of traffic flow sequence among network nodes in the time dimension, and the spatiotemporal graph of traffic flow is constructed to capture the spatiotemporal characteristics and dependencies of traffic flow. By combining graph attention network and time attention network, a spatiotemporal convolution block is constructed to capture spatiotemporal characteristics of traffic data. Experiments on open data sets PEMSD4 and PEMSD8 show that TSADGCN has higher prediction accuracy than well-known traffic flow prediction algorithms.Keywords
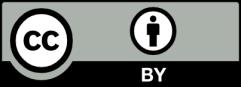