Open Access
ARTICLE
SAM Era: Can It Segment Any Industrial Surface Defects?
1 National Key Laboratory of Advanced Casting Technologies, Shenyang, 110022, China
2 School of Mechanical Engineering & Automation, Northeastern University, Shenyang, 110819, China
* Corresponding Authors: Kechen Song. Email: ; Yunhui Yan. Email:
(This article belongs to the Special Issue: Machine Vision Detection and Intelligent Recognition)
Computers, Materials & Continua 2024, 78(3), 3953-3969. https://doi.org/10.32604/cmc.2024.048451
Received 08 December 2023; Accepted 31 January 2024; Issue published 26 March 2024
Abstract
Segment Anything Model (SAM) is a cutting-edge model that has shown impressive performance in general object segmentation. The birth of the segment anything is a groundbreaking step towards creating a universal intelligent model. Due to its superior performance in general object segmentation, it quickly gained attention and interest. This makes SAM particularly attractive in industrial surface defect segmentation, especially for complex industrial scenes with limited training data. However, its segmentation ability for specific industrial scenes remains unknown. Therefore, in this work, we select three representative and complex industrial surface defect detection scenarios, namely strip steel surface defects, tile surface defects, and rail surface defects, to evaluate the segmentation performance of SAM. Our results show that although SAM has great potential in general object segmentation, it cannot achieve satisfactory performance in complex industrial scenes. Our test results are available at: .Keywords
The birth of foundation models signifies a paradigm shift in the field of artificial intelligence, and sets a new tone for the direction of research. In the past several months, the emergence of ChatGPT, a foundation model, has brought about a revolutionary change in the field of natural language processing. ChatGPT quickly gained a substantial user base due to its outstanding performance and user-friendly interface. Additionally, the encyclopedia-like summarization capabilities and the powerful universality make it easy for people to believe that foundation models have virtually no limitations in handling various problems.
People cannot help but wonder: Has the new era of artificial intelligence arrived? As illustrated in Fig. 1, we present the developmental trajectory of the artificial intelligence. The ubiquitous emergence of various foundation models appears to be steering a paradigm shift, marking 2023 as the commencement of a new era.
Figure 1: Development history of artificial intelligence
Recently, in the field of artificial intelligence, a foundation model called SAM [1] trained on a large visual corpus has also rapidly drawn people’s attention. As a foundational model, SAM has demonstrated powerful segmentation capabilities in various natural scenarios, astonishing researchers with its remarkable performance. Many researchers have begun to try to extend SAM to the field of medical images to solve some existing problems. However, researchers have found that SAM has poor generalization ability when facing a variety of medical scenes, which makes people have to re-discuss the segmentation performance of SAM. Therefore, in this context, it is crucial to investigate reliable and efficient medical segmentation algorithms based on SAM models that can handle frequent changes in medical scenarios. Researchers have tried to combine the SAM model with other theoretical paradigms, and have achieved some encouraging results. As shown in Table 1, we show some improved paradigms for medical images. In general, SAM has been successfully applied to the task of medical image segmentation through fine-tuning, adaptation and direct improvement.
The above improved methods have made some good progress in the medical field, but few researchers extend it to the industrial defect detection field to verify the performance of SAM. The ability of SAM to generalize to this specific scenario of industrial surface defect detection deserves discussion and re-evaluation. Therefore, we focus on defect surface detection for the first time to evaluate and validate the performance of SAM from several aspects. We also make discussions on the future development direction of SAM in the field of defect detection based on the results of the evaluation, which is also the motivation and potential significance of this research.
In this paper, we explore the segmentation capabilities of SAM compared with 13 state-of-the-art models in the field of industrial surface defect detection. We conduct a quantitative comparison and present visual results. The results show that SAM has limitations in industrial surface defect detection and performs averagely in industrial settings. Furthermore, we believe that this provides an opportunity for further research into how SAM can be better applied to industrial surface defect detection tasks.
The main contributions of this paper are summarized as follows:
1. This paper engages in discussions regarding the effect of the paradigm shift brought about by foundation models on the direction of defect detection. What’s more, in light of the new era of SAM, we pioneer the analysis on how to use SAM to serve downstream defect detection tasks with the aim of providing insights and assistance to future researchers.
2. To verify the performance of SAM, we conduct a series of experiments on three industrial benchmarks. Experimental results show that SAM has a substantial gap in performance compared with state-of-the-art saliency detection methods. However, some positive cases also illustrate the great potential of SAM for defect detection, which also proves that it is feasible to improve the model based on SAM.
Meta recently released a groundbreaking model called the Segment Anything Model (SAM). As the title suggests, the paper focuses on zero-shot segmentation, similar to how ChatGPT addresses every query in natural language processing. SAM leverages interactive annotation data to construct the largest existing dataset (SA-1B) with high-quality and diverse masks. The experimental results demonstrate that SAM is effective in zero-sample instance segmentation, edge detection, and other tasks. Additionally, Meta open-sourced the code and demo website in the original paper, allowing for the prompt engineering or automatic generation strategies to be used to obtain desired segmentation masks, as displayed in Fig. 2 on the demo website. The attained segmentation results clearly indicate the immense potential of SAM.
Figure 2: We use several prompt projects to obtain the segmentation results on the demo website, and the image is from the demo website publicly available by SAM
However, segmentation is a vast field, with approaches such as interactive segmentation and edge detection not being ideal for industrial surface defect detection tasks. As industrial production efficiency improves, industrial surface defect detection must be carried out in real-time, making interactive segmentation methods unfeasible. Furthermore, defect detection tasks typically only require defect and background segmentation, thus rendering instance segmentation methods unsuitable. Therefore, we use the strategy of SAM to generate masks automatically to obtain all segmentation results.
Zero-shot generalization aims to learn a model that is trained with a specific training data during the training phase, while in the testing phase it generalizes this model to a new class of data that has not been seen during the training phase. Due to the complexity and change of the industrial manufacturing environment, there will always be various unknown types of defects. Therefore, this problem setting has attracted a lot of attention from researchers.
For example, Li et al. [7] proposed a zero-shot surface defect recognition with class knowledge graph framework to establish the relationship between base and novel defect classes. Zhu et al. [8] integrated semantic attribute predictors with visual features during training, which achieved significant improvements on multiple unseen classes. With the development of foundation models of visual language, studies that exploit the zero-shot capability of large models have begun to emerge in the field of defects. Yong et al. [9] combined a contrastive language image pre-training model with cue engineering to enhance the zero-shot generalization ability of the model, showing that improvements based on the foundational vision model are expected to be an alternative to existing defect detection models under insufficient dataset. In addition, Manettas et al. [10] used a data simulation generation framework to generate synthetic defect images to solve the problem of insufficient data sets in defect detection, which improved the zero-shot training problem in the training phase.
In recent years, network models based on convolution neural networks structures [11] have flourished in the salient object detection field with the rapid development of deep learning technology. Wu et al. [12] proposed a novel Cascaded Partial Decoder framework (CPD) for fast and accurate salient object detection. Inspired by edge mapping and segmentation, Wu et al. [13] proposed a novel Stacked Cross Refinement Network (SCRN), aiming to refine salient objects and edge features simultaneously. Pang et al. [14] proposed a multi-scale aggregation interaction strategy, which uses consistency loss to highlight the difference between foreground and background. To address the dilution problem in the top-down process of high-level features, references [15–17] generated saliency maps by progressive context-aware feature interactions. The above methods are all built using convolutional neural networks, which have limitations in capturing global features. Therefore, researchers [18,19] began to use the advantages of transformer architecture to build saliency detection models, and achieve more excellent results. However, the transformer architecture makes the model difficult to deploy due to the huge number of parameters, so lightweight salient object detection models [20,21] have also been widely explored to promote practical applications.
The success of saliency detection in natural images has brought great inspiration to researchers in the industrial field. However, due to the significant disparities between defect images and natural images, it can be challenging to achieve superior performance by directly applying methods from the aforementioned fields to the industrial sector. In addition, with the rising demand for automation and efficiency in industrial environments, there is a growing need for effective supervised testing. While unsupervised zero-shot generalization holds great promise, supervised detection is more practical in current industrial fields due to its higher accuracy and detection rate. For example, Song et al. [22] proposed an encoder-decoder residual network (EDRNet) architecture to solve the detection problem of steel strip surface defects. Zhou et al. [23] used a cascade feature integration module to fuse multi-branch features and then used an intensive attention mechanism to gradually restore spatial details to obtain the final features, Therefore, the detection accuracy of defect images is effectively improved. In order to solve the problem that existing defect saliency methods often only focus on feature interaction and ignore edge information, Ding et al. [24] proposed a cross-scale edge purification network to explore feature correlation at different scales and achieved state-of-the-art results on defect datasets.
This section describes the defect benchmark dataset used for performance evaluation, the Mask selection strategy, and the evaluation metrics used.
No Line breaks between paragraphs belonging to the same section. To evaluate the effectiveness and the applicability of SAM, we conduct extensive and convincing experiments on three benchmark datasets related to surface defects, including SD-saliency-900 dataset [25], Magnetic tile dataset (MT) [26] and NRSD-MN dataset [27], whose details are shown in Table 2 and Fig. 3.
Figure 3: Examples of three datasets
1) SD-saliency-900 dataset: It contains complex industrial images, such as challenging scenes with low contrast and defect scale changes. To be specific, it contains three defect categories: patch, inclusion, and scratch, where each one includes 300 images.
2) MT dataset: It contains 392 defective images with different resolutions and corrupted by various factors such as illumination and textured background. We randomly divided training sets and test sets in a 1:1 ratio to train and test all models.
3) NRSD-MN dataset: The NRSD-MN dataset is also regarded as an evaluation dataset for validating the performance of saliency detection methods, which contain 2086 images for training and 1130 images including 965 artificial images, which are more challenging to segment for testing. Affected by different production processes and external factors, the surface of the rails has different degrees of oxidation and corrosion.
Under the automatic generation strategy of SAM, multiple masks are generated for each image. Given the specificity of industrial surface defect detection tasks, a single image commonly contains multiple significant defects. Therefore, we employ a selection strategy that superimposes or negates the multiple masks generated by SAM for each image to produce masks that are closest to the ground truth. This strategy is more advantageous for SAM compared with simply selecting the mask with the highest IOU or F-measure score among the multiple masks. As shown in Fig. 4, we show an example of our process of selecting and selecting masks. For other state-of-the-art models, we use masks automatically generated by their test code.
Figure 4: Mask selection details
We use five evaluation measures to evaluate SAM and compare it with other salient object detection methods, including Sa [28], MAE, max Em [29], Fw [30] and max Fm [31].
S-measure (Sa, a = 0.5) It focuses on assessing structural similarity, which is closer to human visual perception. The calculation formula is:
MAE (Mae) The MAE measures the dissimilarity between the normalized predicted saliency map P and the ground truth G. We normalize P and G to [0; 1], so the MAE score can be computed as:
E-measure (Em) capture image-level statistics and local pixel matching information. In our experiment, we adopt maximum E-measure (max Em) as our evaluation metric:
Weighted F-measure (Fw) is a weighted version of traditional widely used F-measure, Thus, it amends the interpolation, dependency, and equal importance flaws of MAE and F-measure, where we set β2 to 0.3 as suggested in [19]:
F-measure (Fm) is defined as a weighted harmonic mean of precision and recall for comprehensively evaluating the quality of the saliency map. We adopt maximum F-measure (max Fm) as our evaluation metric.
4 Experiments and Performance Evaluation
This section describes all the saliency detection methods compared and the results on the three publicly available datasets. All methods are implemented on the PyTorch framework with one NVIDIA GeForce RTX 3080 GPU (10 GB memory). For a fair comparison, all the experiments are carried out in Ubuntu 20.04 + python 3.8 environments.
More specifically, we use the public source code settings of saliency detection methods and the test results provided by the authors after training to ensure the comparability of results. Some publicly available methods for natural images lack test results on the defect dataset provided by the authors, and we conduct an adequate training process for these methods. We do not change the hyperparameters in the public source code other than the batch size, as this tends to lead to worse detection results. For the three defect saliency detection methods [29–31], we use the comparison results on the defect datasets provided by the authors themselves in the open literature. What’s more, we train each method three times on each dataset to get a more effective result to eliminate randomness.
Tables 3–5 report the quantitative results on three industrial surface defect datasets, in which thirteen representative detection methods are compared to verify the effectiveness of SAM, including nine methods for saliency detection of natural scene (CPD [12], SCRN [13], MINet [14], GCPANet [15], F3Net [16], C2FNet [17], VST [18], ICON [19] and EDN [20] and CorrNet [21]), three methods for saliency detection of surface defects (EDRNet [22], DACNet [23] and CSEPNet [24]).
Taking the results of SD-saliency-900 dataset as an example, we show the performance of SAM (VIT-H) and other 13 methods on five metrics denoted as Sa, MAE, max Em, Fw and max Fm as reported in Table 3. Briefly speaking, the performance of SAM on three industrial benchmarks is limited and still lags far behind state-of-the-art models. The best method CSEPNet outperforms SAM by 24%, 87%, 25%, 30%, 29% on Sa, Mae, Em, Fw and Fm, respectively. Under the selection of strong human intervention in favor of SAM method, SAM still has a large gap compared to other state-of-the-art (SOTA) methods. We can also draw this conclusion in the results in Tables 4 and 5, which also proves the fact that SAM has relatively poor generalization in the defect domain. To some extent, SAM is not able to segment arbitrary defects.
As shown in Fig. 5, for an intuitive comparison of the performance of SAM, we provide visualization results of SAM and several state-of-art methods on three datasets. It can be seen that SAM is unable to segment defects in some challenging scenes, such as complex and low contrast images. Even in the case of strong manual intervention of SAM, the existing state-of-the-art algorithms can still achieve more competitive performance.
Figure 5: Visual comparisons with nine SOTA methods on several challenging defect scenes
In addition, we conducted qualitative experiments on three datasets respectively. We selected five representative methods as competitors to the SAM method.
1) Comparisons on the SD-saliency-900 dataset: As shown in Fig. 6, there are many different defect types on the steel strip surface, and the segmentation effect is not the same when SAM faces different types. When facing the inclusion defect with weak texture features, SAM is easy to segment its boundary as a dividing line. This indicates that SAM has identified the defect, however there is an ambiguity expression as to whether it should belong to the foreground or background. Secondly, SAM can hardly identify the patch defects with extremely low contrast, so it will segment the whole image into foreground. SAM can accurately mine the features of scratch defects, because scratch defects are relatively bright in the figure. Scratch defects do not have features such as camouflage or low contrast, and are closer to natural images, which is why SAM can segment them accurately. At the same time, it can be observed in the last row that SAM can even segment some scratch defects that are not labeled at the boundary position.
Figure 6: Qualitative comparisons with five representative methods on the SD-saliency-900 dataset
2) Comparisons on the MT dataset: As shown in Fig. 7, different from strip surface defects, magnet tile defects contain more tiny defects and single defects. In fact, industrial scenarios often contain more minor defects. Therefore, to some extent, the qualitative results of MT dataset can better reflect the performance of SAM on defective surfaces. It can be seen in the first and second rows that the SAM method can generalize well to a single defect in the tile defect when trained with zero defect samples. At the same time, for slender and small defects, SAM can also achieve accurate segmentation. The results in the third row show that SAM is even more accurate than manual annotation when segmenting slender defects. However, when facing the uneven defects that often appear in the magnet and are difficult to observe manually, SAM is difficult to predict the defect location. This indicates that SAM has poor segmentation ability in the face of defect types with high similarity between foreground and background.
Figure 7: Qualitative comparisons with five representative methods on the MT dataset
3) Comparisons on the NRSD-MN dataset: Because the NRSD dataset contains some man-made defects rather than natural defects, SAM produced better results when faced with this dataset. Metrics such as Mae and Sa also have the lowest percentage compared to the most advanced methods compared to the strip and magnetic tile datasets. Lines one through four of Fig. 8 also illustrate this trend. However, when faced with ambiguous background and foreground, SAM still incorrectly predicts the background as a defect, as shown in the fifth line of Fig. 8. This shows that the SAM method has some limitations, but it also gives us a glimpse of the future potential of SAM in the direction of defect detection.
Figure 8: Qualitative comparisons with five representative methods on the NRSD-MN dataset
As shown in Fig. 9, we present example images demonstrating the accurate segmentation predictions of SAM, where other state-of-the-art models failed to accurately segment these defects. It can be observed that SAM has a good zero-sample generalization ability in the face of tiny defect images. This is because SAM is trained on large-scale instance segmentation datasets and has excellent fine-grained instance partitioning ability. This ability enables SAM to segment multiple different instances, including small defect instances and other interfering impurities, when faced with defect images. On the other hand, other models are trained on smaller defect datasets and pay more attention to the most significant defect regions. More importantly, it is easier for SAM to mine small defect features due to the difference in the resolution of the input. Therefore, under the selection strategy with manual intervention, SAM has good zero-shot generalization ability when facing small defect images. In fact, tiny defect images tend to appear more frequently in industrial scenes than other defects. Meanwhile, SAM can completely segment the defects in the face of some single defect images. These findings indicate the significant potential of SAM in in the field of defect detection. It may be worth focusing on refining SAM based on the large model for specific contexts to ensure that it is better suited for downstream tasks. This is a direction that may require ongoing attention in the future.
Figure 9: Some good cases of SAM on three industrial benchmarks
4.5 Extension Experiment on MT Dataset
To further evaluate the performance of SAM, we conduct detailed and rich experiments on MT dataset. Specifically, since the MT dataset is not partitioned into training and test sets. Therefore, not only following the 1:1 partition as in many literatures, we also repartition the training and test sets according to the ratio of 7:3 commonly used in defect detection. We selected the three most advanced methods in Table 1, namely ICON [22], EDRNet [23] and CSEPNet [24], for comparison with SAM. As shown in Table 6, when the training and test sets are divided according to the ratio of 7 to 3, the performance of all methods is improved to some extent. When the number of defect images involved in training increases, the saliency detection algorithm has more prominent defect recognition ability. However, there is still a large gap between SAM and the three most advanced models in various indicators. This further indicates that SAM lacks zero-shot generalization ability to defects.
5.1 Limitations of Segment Anything Model
Segment anything model aims to advance segmentation to the era of foundation models, marking a groundbreaking task. However, while the overall performance of SAM is satisfactory, there are numerous limitations concerning its practical application to downstream defect detection tasks.
1) Limitations of real-time: In industry, real-time surface defect detection tasks are indispensable to keep up with the growing demand for production efficiency. What’s more, the real-time requirements in different industrial scenarios are usually different. Even though the SAM can process single-image prompts in real-time, its collective performance is not in real-time. As shown in Table 7, we report the average processing time of some representative on the three publicly available defect datasets. We use the NVIDIA 3080 GPU for evaluation of all the methods to ensure a fair comparison. SAM has the largest number of parameters compared to other saliency models. What’s more, the processing speed of SAM is only 0.2 FPS, which is much lower than any of the saliency detection methods, indicating that SAM does not have real-time processing ability.
2) Limitations of the task attribute: SAM is designed to be versatile and broadly applicable, instead of being specifically tailored for semantic segmentation or saliency detection tasks. The automatic generation strategy of SAM will produce multiple masks when processing each image, necessitating manual intervention to select the most effective mask, which is impractical for industrial defect detection tasks. The image-level method for real-time identification of suspected defect images and some rough localization methods, such as object detection, are more suitable in some cases.
3) Limitations of specific scenarios: In fact, each mask generated by SAM only encapsulates one defect, which makes it difficult to apply SAM to the downstream task of defect detection under the existing framework. To optimize the performance of SAM in saliency detection tasks, we resort to post-processing the masks. However, this manual intervention strategy still fails to meet the performance of SAM in specific defect detection scenarios, such as low contrast, cluttered background, and multiple significant defect regions. More importantly, the screening strategy in this paper is to more effectively tap the potential of SAM in challenging industrial scenarios. Therefore, the limitations of SAM when facing real specific defect tasks are greater than the results presented in this paper.
5.2 The Application Prospect of Segment Anything Model
Assuming the training and testing images have the same distribution, existing saliency detection algorithms demonstrate remarkable accuracy. However, in practical detection, defect images in different industrial settings exhibit significant distributional diversity due to varying production conditions and product types, which requires researchers to design network architectures tailored to specific industrial scenarios. Furthermore, due to the rarity of defect images in industrial settings and the diversity of detection conditions, it is impossible to collect and label defect samples from all industrial settings.
Although SAM does not exhibit the best performance under the current framework, the zero-shot transfer learning approach suggests tremendous potential for the application of SAM in defect detection. Additionally, as shown in Fig. 6, some promising examples indicate that SAM has already surpassed the best methods in predicting the integrity of small and hidden defects. In future defect detection research, several potential improvement directions can be pursued based on SAM. 1) Fine-tuning or improving SAM model enhance its fitting ability to defect data, and 2) using it as a foundation model to construct new network that train defect data and adapt to downstream defect detection tasks, such as saliency detection, domain generalization and other industrial detection tasks.
In the field of medical image, there have been many successful improvement cases, but there is still no clear and effective improvement method in the field of defect detection. The application of basic large model in the field of defect detection is most urgent to solve the problem of parameter quantity, so as to promote the development of real-time. It is an improved idea to try to replace VIT-H with VIT-Tiny as encoder with a smaller number of parameters, and combine knowledge distillation theory to realize lighter MobileSAM. Secondly, in the face of extremely scarce defect images, it is effective to construct a defect-oriented high-precision defect detection model by combining the small-sample theory and domain generalization theory for transfer learning training. It has been proved in many literatures that the theory of small sample learning and domain generalization is feasible in the field of defect detection.
In addition, based on the unique interaction characteristics of SAM and the integration of expert knowledge to fine-tune the model, a defect SAMPrompter method is generated to remove the cumbersome annotation steps. Finally, modifying the image encoder to construct a defect 3DSAM system suitable for the defect video volume input can accelerate the efficiency of some industrial defect detection tasks in some specific scenarios.
Overall, although the current framework of SAM is still insufficient for defect detection scenarios, it paves the way for a new solution in future automated defect detection. Based on the basic large model theory and combined with different industrial scenarios and advanced theories, the multi-task oriented intelligent industrial defect detection model under the guidance of multiple theories will be the development direction of defect tasks in the future.
This paper evaluates the performance of SAM in defect detection tasks. The experiments indicate that the performance of SAM in defect detection falls far behind that of state-of-the-art models. However, it can be inferred from some positive outcomes that SAM has great potential in the field of defect detection. We hope that our paper can provide help and insights for future researchers.
It is foreseeable that researchers would need to focus on making targeted improvements to the foundation model SAM or utilizing its exceptional features to make it suitable for downstream tasks in the future. The combination of large model and various advanced theories is one of the development directions of defect detection in the future. This direction of research merits ongoing attention.
Acknowledgement: We would like to express our sincere appreciation to the National Natural Science Foundation of China, Chunhui Plan Cooperative Project of Ministry of Education, and the 111 Project for providing the necessary financial support to conduct this research project.
Funding Statement: This work was supported by the National Natural Science Foundation of China (51805078), Project of National Key Laboratory of Advanced Casting Technologies (CAT2023-002), and the 111 Project (B16009).
Author Contributions: Study conception and design: Kechen Song, Wenqi Cui; data collection: Kechen Song, Wenqi Cui; analysis and interpretation of results: Han Yu, Yunhui Yan; draft manuscript preparation: Wenqi Cui, Xingjie Li. All authors reviewed the results and approved the final version of the manuscript.
Availability of Data and Materials: The data that support the findings of this study are openly available.
Conflicts of Interest: The authors declare that they have no conflicts of interest to report regarding the present study.
References
1. A. Kirillov et al., “Segment anything,” arXiv preprint arXiv:2304.02643, 2023. [Google Scholar]
2. J. Ma and B. Wang, “Segment anything in medical images,” arXiv preprint arXiv:2304.12306, 2023. [Google Scholar]
3. T. Shaharabany, A. Dahan, R. Giryes, and L. Wolf, “AutoSAM: Adapting SAM to medical images by overloading the prompt encoder,” arXiv preprint arXiv:2306.06370, 2023. [Google Scholar]
4. S. Gong et al., “3DSAM-adapter: Holistic adaptation of SAM from 2D to 3D for promptable medical image segmentation,” arXiv preprint arXiv:2306.13465, 2023. [Google Scholar]
5. Y. Gao, W. Xia, D. Hu, and X. Gao, “DeSAM: Decoupling segment anything model for generalizable medical image segmentation,” arXiv preprint arXiv:2306.00499, 2023. [Google Scholar]
6. W. Lei, X. Wei, X. Zhang, K. Li, and S. Zhang, “MedLSAM: Localize and segment anything model for 3D medical images,” arXiv preprint arXiv:2306.14752, 2023. [Google Scholar]
7. Z. Li, L. Gao, Y. Gao, X. Li, and H. Li, “Zero-shot surface defect recognition with class knowledge graph,” Adv. Eng. Inform., vol. 54, pp. 101813, 2022. doi: 10.1016/j.aei.2022.101813. [Google Scholar] [CrossRef]
8. P. Zhu, H. Wang, and V. Saligrama, “Zero shot detection,” IEEE Trans. Circuits Syst Video Technol, vol. 30, no. 4, pp. 998–1010, 2019. doi: 10.1109/TCSVT.2019.2899569. [Google Scholar] [CrossRef]
9. G. Yong, K. Jeon, D. Gil, and G. Lee, “Prompt engineering for zero-shot and few-shot defect detection and classification using a visual-language pretrained model,” Comput. Aided Civ. Infrastruct. Eng., vol. 38, pp. 1536–1554, 2023. doi: 10.1111/mice.12954. [Google Scholar] [CrossRef]
10. C. Manettas, N. Nikolakis, and K. Alexopoulos, “Synthetic datasets for deep learning in computer-vision assisted tasks in manufacturing,” in Proc. CIRP, vol. 103, pp. 237–242, 2021. doi: 10.1016/j.procir.2021.10.038. [Google Scholar] [CrossRef]
11. P. Stavropoulos, A. Papacharalampopoulos, and D. Petridis, “A vision-based system for real-time defect detection: A rubber compound part case study,” in Proc. CIRP, vol. 93, pp. 1230–1235, 2020. doi: 10.1016/j.procir.2020.04.159. [Google Scholar] [CrossRef]
12. Z. Wu, L. Su, and Q. Huang, “Cascaded partial decoder for fast and accurate salient object detection,” in Proc. IEEE/CVF Conf. Comput. Vis. Pattern Recognit. (CVPR), 2019, pp. 3907–3916. [Google Scholar]
13. Z. Wu, L. Su, and Q. Huang, “Stacked cross refinement network for edge-aware salient object detection,” in Proc. IEEE/CVF Int. Conf. Comput. Vis. (ICCV), 2019, pp. 7264–7273. [Google Scholar]
14. Y. Pang, X. Zhao, L. Zhang, and H. Lu, “Multi-scale interactive network for salient object detection,” in Proc. IEEE/CVF Conf. Comput. Vis. Pattern Recognit. (CVPR), 2020, pp. 9413–9422. [Google Scholar]
15. Z. Chen, Q. Xu, R. Cong, and Q. Huang, “Global context-aware progressive aggregation network for salient object detection,” in Proc. AAAI Conf. Artif. Intell., 2020, vol. 34, no. 7, pp. 10599–10606. [Google Scholar]
16. J. Wei, S. Wang, and Q. Huang, “F3Net: Fusion, feedback and focus for salient object detection,” in Proc. AAAI. Conf. Artif. Intell., vol. 34, no. 7, pp. 12321–12328, 2020. doi: 10.1609/aaai.v34i07.6916. [Google Scholar] [CrossRef]
17. Y. Sun, G. Chen, T. Zhou, Y. Zhang, and N. Liu, “Context-aware crosslevel fusion network for camouflaged object detection,” arXiv preprint arXiv:2105.12555, 2021. [Google Scholar]
18. N. Liu, N. Zhang, K. Wan, L. Shao, and J. Han, “Visual saliency transformer,” in Proc. IEEE/CVF Int. Conf. Comput. Vis. (ICCV), 2021, pp. 4722–4732. [Google Scholar]
19. M. Zhuge, D. P. Fan, N. Liu, D. Zhang, D. Xu and L. Shao, “Salient object detection via integrity learning,” IEEE Tran. Pattern Anal. Mach. Intell., vol. 45, no. 3, pp. 3738–3752, 2023. [Google Scholar] [PubMed]
20. Y. H. Wu, Y. Liu, L. Zhang, M. M. Cheng, and B. Ren, “EDN: Salient object detection via extremely-downsampled network,” IEEE Trans. Image Process., vol. 31, pp. 3125–3136, 2022. doi: 10.1109/TIP.2022.3164550. [Google Scholar] [PubMed] [CrossRef]
21. G. Li, Z. Liu, X. Zhang, and W. Lin, “Lightweight salient object detection in optical remote-sensing images via semantic matching and edge alignment,” IEEE Trans. Geosci. Remote. Sens., vol. 61, pp. 1–11, 2023. doi: 10.1109/TGRS.2023.3235717. [Google Scholar] [CrossRef]
22. G. Song, K. Song, and Y. Yan, “EDRNet: Encoder-decoder residual network for salient object detection of strip steel surface defects,” IEEE Trans. Instrum. Meas., vol. 69, no. 12, pp. 9709–9719, 2020. doi: 10.1109/TIM.2020.3002277. [Google Scholar] [CrossRef]
23. X. Zhou et al., “Dense attention-guided cascaded network for salient object detection of strip steel surface defects,” IEEE Trans. Instrum. Meas., vol. 71, pp. 1–14, 2022. doi: 10.1109/TIM.2021.3132082. [Google Scholar] [CrossRef]
24. T. Ding, G. Li, Z. Liu, and Y. Wang, “Cross-scale edge purification network for salient object detection of steel defect images,” Meas., vol. 199, no. 1, pp. 111429, 2022. doi: 10.1016/j.measurement.2022.111429. [Google Scholar] [CrossRef]
25. G. Song, K. Song, and Y. Yan, “Saliency detection for strip steel surface defects using multiple constraints and improved texture features,” Opt. Laser. Eng., vol. 128, pp. 106000, 2020. doi: 10.1016/j.optlaseng.2019.106000. [Google Scholar] [CrossRef]
26. Y. Huang, C. Qiu, and K. Yuan, “Surface defect saliency of magnetic tile,” The Vis. Comput., vol. 36, no. 1, pp. 85–96, 2020. doi: 10.1007/s00371-018-1588-5. [Google Scholar] [CrossRef]
27. D. Zhang, K. Song, J. Xu, Y. He, M. Niu and Y. Yan, “MCnet: Multiple context information segmentation network of no-service rail surface defects,” IEEE Trans. Instrum. Meas., vol. 70, pp. 1–9, 2021. doi: 10.1109/TIM.2021.3127641. [Google Scholar] [CrossRef]
28. D. P. Fan, M. M. Cheng, Y. Liu, T. Li, and A. Borji, “Structure-measure: A new way to evaluate foreground maps,” in 2017 IEEE Int. Conf. Comput. Vis. (ICCV), Venice, Italy, 2017, pp. 4558–4567. [Google Scholar]
29. D. P. Fan, C. Gong, Y. Cao, B. Ren, M. M. Cheng and A. Borji, “Enhanced-alignment measure for sbinary foreground map evaluation,” arXiv preprint arXiv:1805.10421, 2018. [Google Scholar]
30. A. Borji, M. M. Cheng, H. Jiang, and J. Li, “Salient object detection: A benchmark,” IEEE Trans. Image Process., vol. 24, no. 12, pp. 5706–5722, 2015. doi: 10.1109/TIP.2015.2487833. [Google Scholar] [PubMed] [CrossRef]
31. R. Margolin, L. Zelnik-Manor, and A. Tal, “How to evaluate foreground maps,” in 2014 IEEE Conf. Comput. Vis. Pattern Recognit. (CVPR), Columbus, OH, USA, 2014, pp. 248–255. [Google Scholar]
Cite This Article
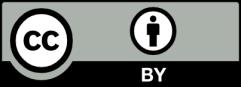