Open Access
ARTICLE
A Spectral Convolutional Neural Network Model Based on Adaptive Fick’s Law for Hyperspectral Image Classification
1 School of Artificial Intelligence (School of Future Technology), Nanjing University of Information Science & Technology, Nanjing, 210044, China
2 College of Computer Science and Engineering, Shandong University of Science and Technology, Qingdao, 266590, China
3 Department of Mathematics, Chaudhary Charan Singh University, Meerut, Uttar Pradesh, 250004, India
* Corresponding Author: Chien-Ming Chen. Email:
Computers, Materials & Continua 2024, 79(1), 19-46. https://doi.org/10.32604/cmc.2024.048347
Received 05 December 2023; Accepted 07 March 2024; Issue published 25 April 2024
Abstract
Hyperspectral image classification stands as a pivotal task within the field of remote sensing, yet achieving high-precision classification remains a significant challenge. In response to this challenge, a Spectral Convolutional Neural Network model based on Adaptive Fick’s Law Algorithm (AFLA-SCNN) is proposed. The Adaptive Fick’s Law Algorithm (AFLA) constitutes a novel metaheuristic algorithm introduced herein, encompassing three new strategies: Adaptive weight factor, Gaussian mutation, and probability update policy. With adaptive weight factor, the algorithm can adjust the weights according to the change in the number of iterations to improve the performance of the algorithm. Gaussian mutation helps the algorithm avoid falling into local optimal solutions and improves the searchability of the algorithm. The probability update strategy helps to improve the exploitability and adaptability of the algorithm. Within the AFLA-SCNN model, AFLA is employed to optimize two hyperparameters in the SCNN model, namely, “numEpochs” and “miniBatchSize”, to attain their optimal values. AFLA’s performance is initially validated across 28 functions in 10D, 30D, and 50D for CEC2013 and 29 functions in 10D, 30D, and 50D for CEC2017. Experimental results indicate AFLA’s marked performance superiority over nine other prominent optimization algorithms. Subsequently, the AFLA-SCNN model was compared with the Spectral Convolutional Neural Network model based on Fick’s Law Algorithm (FLA-SCNN), Spectral Convolutional Neural Network model based on Harris Hawks Optimization (HHO-SCNN), Spectral Convolutional Neural Network model based on Differential Evolution (DE-SCNN), Spectral Convolutional Neural Network (SCNN) model, and Support Vector Machines (SVM) model using the Indian Pines dataset and Pavia University dataset. The experimental results show that the AFLA-SCNN model outperforms other models in terms of Accuracy, Precision, Recall, and F1-score on Indian Pines and Pavia University. Among them, the Accuracy of the AFLA-SCNN model on Indian Pines reached 99.875%, and the Accuracy on Pavia University reached 98.022%. In conclusion, our proposed AFLA-SCNN model is deemed to significantly enhance the precision of hyperspectral image classification.Keywords
Cite This Article
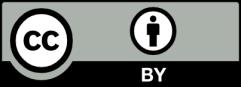