Open Access
ARTICLE
U-Net Inspired Deep Neural Network-Based Smoke Plume Detection in Satellite Images
1 Centre for Cyber Physical Systems, Vellore Institute of Technology (VIT), Chennai, Tamil Nadu, 600127, India
2 School of Computer Science and Engineering, Vellore Institute of Technology (VIT), Chennai, Tamil Nadu, 600127, India
* Corresponding Author: Ayesha Shaik. Email:
(This article belongs to the Special Issue: Machine Vision Detection and Intelligent Recognition)
Computers, Materials & Continua 2024, 79(1), 779-799. https://doi.org/10.32604/cmc.2024.048362
Received 05 December 2023; Accepted 30 January 2024; Issue published 25 April 2024
Abstract
Industrial activities, through the human-induced release of Green House Gas (GHG) emissions, have been identified as the primary cause of global warming. Accurate and quantitative monitoring of these emissions is essential for a comprehensive understanding of their impact on the Earth’s climate and for effectively enforcing emission regulations at a large scale. This work examines the feasibility of detecting and quantifying industrial smoke plumes using freely accessible geo-satellite imagery. The existing system has so many lagging factors such as limitations in accuracy, robustness, and efficiency and these factors hinder the effectiveness in supporting timely response to industrial fires. In this work, the utilization of grayscale images is done instead of traditional color images for smoke plume detection. The dataset was trained through a ResNet-50 model for classification and a U-Net model for segmentation. The dataset consists of images gathered by European Space Agency’s Sentinel-2 satellite constellation from a selection of industrial sites. The acquired images predominantly capture scenes of industrial locations, some of which exhibit active smoke plume emissions. The performance of the above-mentioned techniques and models is represented by their accuracy and IOU (Intersection-over-Union) metric. The images are first trained on the basic RGB images where their respective classification using the ResNet-50 model results in an accuracy of 94.4% and segmentation using the U-Net Model with an IOU metric of 0.5 and accuracy of 94% which leads to the detection of exact patches where the smoke plume has occurred. This work has trained the classification model on grayscale images achieving a good increase in accuracy of 96.4%.Keywords
Cite This Article
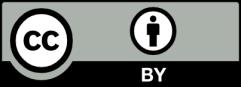