Open Access
ARTICLE
Robust Malicious Executable Detection Using Host-Based Machine Learning Classifier
1 Department of Computer and Systems Engineering, Ain Shams University, Cairo, 11517, Egypt
2 Department of Computer Engineering Technology, ElSewedy University of Technology, Cairo, 44629, Egypt
* Corresponding Author: Khaled Soliman. Email:
Computers, Materials & Continua 2024, 79(1), 1419-1439. https://doi.org/10.32604/cmc.2024.048883
Received 21 December 2023; Accepted 22 February 2024; Issue published 25 April 2024
Abstract
The continuous development of cyberattacks is threatening digital transformation endeavors worldwide and leads to wide losses for various organizations. These dangers have proven that signature-based approaches are insufficient to prevent emerging and polymorphic attacks. Therefore, this paper is proposing a Robust Malicious Executable Detection (RMED) using Host-based Machine Learning Classifier to discover malicious Portable Executable (PE) files in hosts using Windows operating systems through collecting PE headers and applying machine learning mechanisms to detect unknown infected files. The authors have collected a novel reliable dataset containing 116,031 benign files and 179,071 malware samples from diverse sources to ensure the efficiency of RMED approach. The most effective PE headers that can highly differentiate between benign and malware files were selected to train the model on 15 PE features to speed up the classification process and achieve real-time detection for malicious executables. The evaluation results showed that RMED succeeded in shrinking the classification time to 91 milliseconds for each file while reaching an accuracy of 98.42% with a false positive rate equal to 1.58. In conclusion, this paper contributes to the field of cybersecurity by presenting a comprehensive framework that leverages Artificial Intelligence (AI) methods to proactively detect and prevent cyber-attacks.Keywords
Supplementary Material
Supplementary Material FileCite This Article
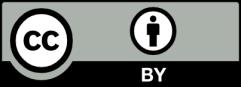