Open Access
ARTICLE
Enhanced Object Detection and Classification via Multi-Method Fusion
1 Department of Computer Science, Air University, Islamabad, 44000, Pakistan
2 Department of Information Systems, College of Computer and Information Sciences, Princess Nourah bint Abdulrahman University, P.O. Box 84428, Riyadh, 11671, Saudi Arabia
3 Department of Computer Science, College of Computer Science and Information System, Najran University, Najran, 55461, Saudi Arabia
4 Department of Computer Sciences, Faculty of Computing and Information Technology, Northern Border University, Rafha, 91911, Saudi Arabia
5 Department of Computer Engineering, Tech University of Korea, 237 Sangidaehak-ro, Siheung-si, Gyeonggi-do, 15073, South Korea
* Corresponding Author: Jeongmin Park. Email:
(This article belongs to the Special Issue: Machine Vision Detection and Intelligent Recognition)
Computers, Materials & Continua 2024, 79(2), 3315-3331. https://doi.org/10.32604/cmc.2024.046501
Received 04 October 2023; Accepted 10 January 2024; Issue published 15 May 2024
Abstract
Advances in machine vision systems have revolutionized applications such as autonomous driving, robotic navigation, and augmented reality. Despite substantial progress, challenges persist, including dynamic backgrounds, occlusion, and limited labeled data. To address these challenges, we introduce a comprehensive methodology to enhance image classification and object detection accuracy. The proposed approach involves the integration of multiple methods in a complementary way. The process commences with the application of Gaussian filters to mitigate the impact of noise interference. These images are then processed for segmentation using Fuzzy C-Means segmentation in parallel with saliency mapping techniques to find the most prominent regions. The Binary Robust Independent Elementary Features (BRIEF) characteristics are then extracted from data derived from saliency maps and segmented images. For precise object separation, Oriented FAST and Rotated BRIEF (ORB) algorithms are employed. Genetic Algorithms (GAs) are used to optimize Random Forest classifier parameters which lead to improved performance. Our method stands out due to its comprehensive approach, adeptly addressing challenges such as changing backdrops, occlusion, and limited labeled data concurrently. A significant enhancement has been achieved by integrating Genetic Algorithms (GAs) to precisely optimize parameters. This minor adjustment not only boosts the uniqueness of our system but also amplifies its overall efficacy. The proposed methodology has demonstrated notable classification accuracies of 90.9% and 89.0% on the challenging Corel-1k and MSRC datasets, respectively. Furthermore, detection accuracies of 87.2% and 86.6% have been attained. Although our method performed well in both datasets it may face difficulties in real-world data especially where datasets have highly complex backgrounds. Despite these limitations, GA integration for parameter optimization shows a notable strength in enhancing the overall adaptability and performance of our system.Keywords
Cite This Article
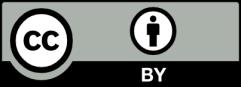