Open Access
ARTICLE
RepBoTNet-CESA: An Alzheimer’s Disease Computer Aided Diagnosis Method Using Structural Reparameterization BoTNet and Cubic Embedding Self Attention
1 College of Computer Science and Artificial Intelligence, Wenzhou University, Wenzhou, 325035, China
2 Key Laboratory of Intelligence Image Processing and Analysis, Wenzhou University, Wenzhou, 325035, China
* Corresponding Author: Zhongyi Hu. Email:
(This article belongs to the Special Issue: Deep Learning in Computer-Aided Diagnosis Based on Medical Image)
Computers, Materials & Continua 2024, 79(2), 2879-2905. https://doi.org/10.32604/cmc.2024.048725
Received 16 December 2023; Accepted 07 April 2024; Issue published 15 May 2024
Abstract
Various deep learning models have been proposed for the accurate assisted diagnosis of early-stage Alzheimer’s disease (AD). Most studies predominantly employ Convolutional Neural Networks (CNNs), which focus solely on local features, thus encountering difficulties in handling global features. In contrast to natural images, Structural Magnetic Resonance Imaging (sMRI) images exhibit a higher number of channel dimensions. However, during the Position Embedding stage of Multi Head Self Attention (MHSA), the coded information related to the channel dimension is disregarded. To tackle these issues, we propose the RepBoTNet-CESA network, an advanced AD-aided diagnostic model that is capable of learning local and global features simultaneously. It combines the advantages of CNN networks in capturing local information and Transformer networks in integrating global information, reducing computational costs while achieving excellent classification performance. Moreover, it uses the Cubic Embedding Self Attention (CESA) proposed in this paper to incorporate the channel code information, enhancing the classification performance within the Transformer structure. Finally, the RepBoTNet-CESA performs well in various AD-aided diagnosis tasks, with an accuracy of 96.58%, precision of 97.26%, and recall of 96.23% in the AD/NC task; an accuracy of 92.75%, precision of 92.84%, and recall of 93.18% in the EMCI/NC task; and an accuracy of 80.97%, precision of 83.86%, and recall of 80.91% in the AD/EMCI/LMCI/NC task. This demonstrates that RepBoTNet-CESA delivers outstanding outcomes in various AD-aided diagnostic tasks. Furthermore, our study has shown that MHSA exhibits superior performance compared to conventional attention mechanisms in enhancing ResNet performance. Besides, the Deeper RepBoTNet-CESA network fails to make further progress in AD-aided diagnostic tasks.Keywords
Cite This Article
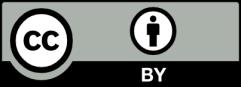
This work is licensed under a Creative Commons Attribution 4.0 International License , which permits unrestricted use, distribution, and reproduction in any medium, provided the original work is properly cited.