Open Access
ARTICLE
Billiards Optimization with Modified Deep Learning for Fault Detection in Wireless Sensor Network
1 Department of Computer Engineering, College of Engineering, Knowledge University, Erbil, 44001, Iraq
2 Engineering College, Al-Kitab University, Kirkuk, Iraq
3 Energy Eng. Department, Technical College of Engineering, Duhok Polytechnic University, Duhok, Iraq
4 Computer Science Department, College of Science, Nawroz University, Duhok, Iraq
* Corresponding Author: Subhi R. M. Zeebaree. Email:
Computer Systems Science and Engineering 2023, 47(2), 1651-1664. https://doi.org/10.32604/csse.2023.037449
Received 03 November 2022; Accepted 28 December 2022; Issue published 28 July 2023
Abstract
Wireless Sensor Networks (WSNs) gather data in physical environments, which is some type. These ubiquitous sensors face several challenges responsible for corrupting them (mostly sensor failure and intrusions in external agents). WSNs were disposed to error, and effectual fault detection techniques are utilized for detecting faults from WSNs in a timely approach. Machine learning (ML) was extremely utilized for detecting faults in WSNs. Therefore, this study proposes a billiards optimization algorithm with modified deep learning for fault detection (BIOMDL-FD) in WSN. The BIOMDLFD technique mainly concentrates on identifying sensor faults to enhance network efficiency. To do so, the presented BIOMDL-FD technique uses the attention-based bidirectional long short-term memory (ABLSTM) method for fault detection. In the ABLSTM model, the attention mechanism enables us to learn the relationships between the inputs and modify the probability to give more attention to essential features. At the same time, the BIO algorithm is employed for optimal hyperparameter tuning of the ABLSTM model, which is stimulated by billiard games, showing the novelty of the work. Experimental analyses are made to affirm the enhanced fault detection outcomes of the BIOMDL-FD technique. Detailed simulation results demonstrate the improvement of the BIOMDL-FD technique over other models with a maximum classification accuracy of 99.37%.Keywords
Cite This Article
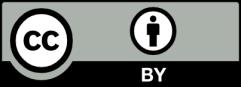