Open Access
ARTICLE
Stock Price Forecasting: An Echo State Network Approach
1 Hunan University of Finance and Economics, Changsha, China
2 University Malaysia Sabah, Kota Kinabalu, Malaysia
3 Yali High School International Department, Changsha, China
* Corresponding Author: Jingjing Lin. Email:
Computer Systems Science and Engineering 2021, 36(3), 509-520. https://doi.org/10.32604/csse.2021.014189
Received 04 September 2020; Accepted 21 November 2020; Issue published 18 January 2021
Abstract
Forecasting stock prices using deep learning models suffers from problems such as low accuracy, slow convergence, and complex network structures. This study developed an echo state network (ESN) model to mitigate such problems. We compared our ESN with a long short-term memory (LSTM) network by forecasting the stock data of Kweichow Moutai, a leading enterprise in China’s liquor industry. By analyzing data for 120, 240, and 300 days, we generated forecast data for the next 40, 80, and 100 days, respectively, using both ESN and LSTM. In terms of accuracy, ESN had the unique advantage of capturing nonlinear data. Mean absolute error (MAE) was used to present the accuracy results. The MAEs of the data forecast by ESN were 0.024, 0.024, and 0.025, which were, respectively, 0.065, 0.007, and 0.009 less than those of LSTM. In terms of convergence, ESN has a reservoir state-space structure, which makes it perform faster than other models. Root-mean-square error (RMSE) was used to present the convergence time. In our experiment, the RMSEs of ESN were 0.22, 0.27, and 0.26, which were, respectively, 0.08, 0.01, and 0.12 less than those of LSTM. In terms of network structure, ESN consists only of input, reservoir, and output spaces, making it a much simpler model than the others. The proposed ESN was found to be an effective model that, compared to others, converges faster, forecasts more accurately, and builds time-series analyses more easily.Keywords
Cite This Article
Citations
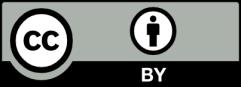