Open Access
ARTICLE
Speech Enhancement via Residual Dense Generative Adversarial Network
1 School of Information Science and Engineering, Southeast University, Nanjing, 210096, China
2 Medizinische Physik and Cluster of Excellence “Hearing4all”, Department of Medical Physics and Acoustics, University of Oldenburg, 26129, Oldenburg, Germany
* Corresponding Author: Lin Zhou. Email:
Computer Systems Science and Engineering 2021, 38(3), 279-289. https://doi.org/10.32604/csse.2021.016524
Received 04 January 2021; Accepted 17 February 2021; Issue published 19 May 2021
Abstract
Generative adversarial networks (GANs) are paid more attention to dealing with the end-to-end speech enhancement in recent years. Various GAN-based enhancement methods are presented to improve the quality of reconstructed speech. However, the performance of these GAN-based methods is worse than those of masking-based methods. To tackle this problem, we propose speech enhancement method with a residual dense generative adversarial network (RDGAN) contributing to map the log-power spectrum (LPS) of degraded speech to the clean one. In detail, a residual dense block (RDB) architecture is designed to better estimate the LPS of clean speech, which can extract rich local features of LPS through densely connected convolution layers. Meanwhile, sequential RDB connections are incorporated on various scales of LPS. It significantly increases the feature learning flexibility and robustness in the time-frequency domain. Simulations show that the proposed method achieves attractive speech enhancement performance in various acoustic environments. Specifically, in the untrained acoustic test with limited priors, e.g., unmatched signal-to-noise ratio (SNR) and unmatched noise category, RDGAN can still outperform the existing GAN-based methods and masking-based method in the measures of PESQ and other evaluation indexes. It indicates that our method is more generalized in untrained conditions.Keywords
Cite This Article
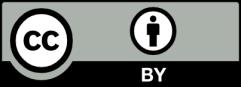