Open Access
ARTICLE
A Fast Panoptic Segmentation Network for Self-Driving Scene Understanding
1 Department of Computer Science, Bahiria University, Islamabad, Pakistan
2 Department of Software Engineering, Bahiria University, Karachi, Pakistan
* Corresponding Author: Sumaira Kausar. Email:
Computer Systems Science and Engineering 2022, 43(1), 27-43. https://doi.org/10.32604/csse.2022.022590
Received 12 August 2021; Accepted 28 October 2021; Issue published 23 March 2022
Abstract
In recent years, a gain in popularity and significance of science understanding has been observed due to the high paced progress in computer vision techniques and technologies. The primary focus of computer vision based scene understanding is to label each and every pixel in an image as the category of the object it belongs to. So it is required to combine segmentation and detection in a single framework. Recently many successful computer vision methods has been developed to aid scene understanding for a variety of real world application. Scene understanding systems typically involves detection and segmentation of different natural and manmade things. A lot of research has been performed in recent years, mostly with a focus on things (a well-defined objects that has shape, orientations and size) with a less focus on stuff classes (amorphous regions that are unclear and lack a shape, size or other characteristics Stuff region describes many aspects of scene, like type, situation, environment of scene etc. and hence can be very helpful in scene understanding. Existing methods for scene understanding still have to cover a challenging path to cope up with the challenges of computational time, accuracy and robustness for varying level of scene complexity. A robust scene understanding method has to effectively deal with imbalanced distribution of classes, overlapping objects, fuzzy object boundaries and poorly localized objects. The proposed method presents Panoptic Segmentation on Cityscapes Dataset. Mobilenet-V2 is used as a backbone for feature extraction that is pre-trained on ImageNet. MobileNet-V2 with state-of-art encoder-decoder architecture of DeepLabV3+ with some customization and optimization is employed Atrous convolution along with Spatial Pyramid Pooling are also utilized in the proposed method to make it more accurate and robust. Very promising and encouraging results have been achieved that indicates the potential of the proposed method for robust scene understanding in a fast and reliable way.Keywords
Cite This Article
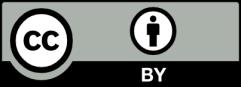