Open Access
ARTICLE
Grid Search for Predicting Coronary Heart Disease by Tuning Hyper-Parameters
1 Department of ECE, Mahendra Institute of Technology, Namakkal, Tamilnadu, 637503, India
2 Department of ECE, Sona College of Technology, Salem, Tamilnadu, 636005, India
3 Department of ECE, Koneru Lakshmaiah Education Foundation, Vijayawada, Andhra Pradesh, 522502, India
4 Department of Information Technology, Kongu Engineering College, Perundurai, Tamilnadu, 638060, India
5 Department of Embedded Technology, Vellore Institute of Technology, Vellore, Tamilnadu, 632014, India
* Corresponding Author: S. Prabu. Email:
Computer Systems Science and Engineering 2022, 43(2), 737-749. https://doi.org/10.32604/csse.2022.022739
Received 17 August 2021; Accepted 22 November 2021; Issue published 20 April 2022
Abstract
Diagnosing the cardiovascular disease is one of the biggest medical difficulties in recent years. Coronary cardiovascular (CHD) is a kind of heart and blood vascular disease. Predicting this sort of cardiac illness leads to more precise decisions for cardiac disorders. Implementing Grid Search Optimization (GSO) machine training models is therefore a useful way to forecast the sickness as soon as possible. The state-of-the-art work is the tuning of the hyperparameter together with the selection of the feature by utilizing the model search to minimize the false-negative rate. Three models with a cross-validation approach do the required task. Feature Selection based on the use of statistical and correlation matrices for multivariate analysis. For Random Search and Grid Search models, extensive comparison findings are produced utilizing retrieval, F1 score, and precision measurements. The models are evaluated using the metrics and kappa statistics that illustrate the three models’ comparability. The study effort focuses on optimizing function selection, tweaking hyperparameters to improve model accuracy and the prediction of heart disease by examining Framingham datasets using random forestry classification. Tuning the hyperparameter in the model of grid search thus decreases the erroneous rate achieves global optimization.Keywords
Cite This Article
S. Prabu, B. Thiyaneswaran, M. Sujatha, C. Nalini and S. Rajkumar, "Grid search for predicting coronary heart disease by tuning hyper-parameters," Computer Systems Science and Engineering, vol. 43, no.2, pp. 737–749, 2022. https://doi.org/10.32604/csse.2022.022739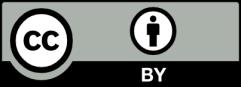