Open Access
ARTICLE
Implicit Continuous User Authentication for Mobile Devices based on Deep Reinforcement Learning
1 Government Engineering College, Idukki, 685603, India
2 APJ Abdul Kalam Technological University, Thiruvananthapuram, 695016, India
* Corresponding Author: Christy James Jose. Email:
Computer Systems Science and Engineering 2023, 44(2), 1357-1372. https://doi.org/10.32604/csse.2023.025672
Received 01 December 2021; Accepted 21 February 2022; Issue published 15 June 2022
Abstract
The predominant method for smart phone accessing is confined to methods directing the authentication by means of Point-of-Entry that heavily depend on physiological biometrics like, fingerprint or face. Implicit continuous authentication initiating to be loftier to conventional authentication mechanisms by continuously confirming users’ identities on continuing basis and mark the instant at which an illegitimate hacker grasps dominance of the session. However, divergent issues remain unaddressed. This research aims to investigate the power of Deep Reinforcement Learning technique to implicit continuous authentication for mobile devices using a method called, Gaussian Weighted Cauchy Kriging-based Continuous Czekanowski’s (GWCK-CC). First, a Gaussian Weighted Non-local Mean Filter Preprocessing model is applied for reducing the noise present in the raw input face images. Cauchy Kriging Regression function is employed to reduce the dimensionality. Finally, Continuous Czekanowski’s Classification is utilized for proficient classification between the genuine user and attacker. By this way, the proposed GWCK-CC method achieves accurate authentication with minimum error rate and time. Experimental assessment of the proposed GWCK-CC method and existing methods are carried out with different factors by using UMDAA-02 Face Dataset. The results confirm that the proposed GWCK-CC method enhances authentication accuracy, by 9%, reduces the authentication time, and error rate by 44%, and 43% as compared to the existing methods.Keywords
Cite This Article
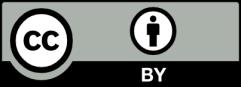