Open Access
ARTICLE
Selection of Wind Turbine Systems for the Sultanate of Oman
1 Department of Electrical and Computer Engineering, Sohar University, Sultanate of Oman
2 Electrical Engineering Department, Faculty of Engineering Technology, Al-Balqa Applied University, Al-Salt, Jordan
* Corresponding Author: M. A. A. Younis. Email:
Computer Systems Science and Engineering 2023, 45(1), 343-359. https://doi.org/10.32604/csse.2023.029510
Received 05 March 2022; Accepted 12 April 2022; Issue published 16 August 2022
Abstract
The Sultanate of Oman has been dealing with a severe renewable energy issue for the past few decades, and the government has struggled to find a solution. In addition, Oman’s strategy for converting power generation to sources of renewable energy includes a goal of 60 percent of national energy demands being met by renewables by 2040, including solar and wind turbines. Furthermore, the use of small-scale energy from wind devices has been on the rise in recent years. This upward trend is attributed to advancements in wind turbine technology, which have lowered the cost of energy from wind. To calculate the internal and external factors that affect the small-scale energy of wind technologies, the study used a fuzzy analytical hierarchy process technique for order of preference by similarity to an ideal solution. As a result, in the decision model, four criteria, seventeen sub-criteria, and three resources of renewable energy were calculated as options from the viewpoint of the Sultanate of Oman. This research is based on an examination of statistics on energy produced by wind turbines at various locations in the Sultanate of Oman. Further, six distinct miniature wind turbines were investigated for four different locations. The outcomes of this study indicate that the tiny wind turbine has a lot of potential in the Sultanate of Oman for applications such as homes, schools, college campuses, irrigation, greenhouses, communities, and small businesses. The government should also use renewable energy resources to help with the renewable energy issue and make sure that the country has enough renewable energy for its long-term growth.Keywords
With one of the world’s largest resource potentials and a fast-expanding demand for electricity, the Sultanate of Oman is positioned to become a pioneer in offshore energy wind in the Middle East region over the next ten years. Energy from wind, alongside with various other environmentally friendly sources of energy, is extensively employed [1–3]. Furthermore, it is becoming gradually clear that environmental contamination is a major concern. The use of clean energy is one of the most effective techniques to lower pollution levels and reduce emissions into the atmosphere. The development of energy from wind is a top goal for the government of Oman.
Within a decade, the Sultanate intends to increase the proportion of renewable energy in global energy sources, with the ultimate goal of reaching 10% renewable energy for Oman. Energy from wind is generally defined as the process through which natural wind is captured, employed for energy, and turned into electrical or mechanical energy for everyday use [4–6]. Wind turbines must be deployed and installed in order to reap the benefits of wind energy. Vertically oriented wind turbines and horizontally oriented wind turbines are the two varieties of modern wind turbines that may be found in the market. Horizontally oriented wind turbines with 2 or 3 blades are the most common configuration. In order to operate, wind turbines must have 3 blades that function on a vertical wind tool, and these blades must be facing squarely into the wind.
Wind turbines can be placed on land or near the shore in aquatic environments, such as the ocean or big reservoirs, and they can generate electricity. Therefore, the wind turbine itself plays a significant role in the improvement of energy efficiency in wind initiatives. Currently, there are a large number of wind turbine manufacturers to choose from. When it comes to energy projects, selecting a reliable supplier has a significant impact on operational efficiency and accomplishment time. Because of this, it is a complicated decision that must keep in mind several diverse considerations. Wind turbine supplier selection in energy projects involving wind is a decision-making procedure involving multi-criteria problems, including a number of various variables. Practices of decision-making for multi-criteria situations, on the other hand, are best described as practices for making judgments where numerous criteria (or objectives) must be examined simultaneously with the intention of attaining an overall position of the alternatives.
In this row, Kahraman et al. [1] employed two fuzzy decision-making strategies for multi-criteria issues in order to pick sources of renewable energy for use in their research. The Analytic Hierarchy Process (AHP) was employed in the first practice, whereas a soft computing technique was employed in the second. Using the presented practice, it was possible to identify the most suitable alternative source of renewable energy for Turkey. According to Lee et al. [2], a unified decision-making technique for multi-criteria problems for the calculation of the best alternatives and solutions using AHP and fuzzy theory was proposed in order to prioritise the energy technologies on the strategic roadmap for the United States. Also, Kahraman and colleagues [3] developed an optimal solution for the scheduling of energy management systems under a variety of hesitations by developing a fuzzy-random interval programming model.
It was decided in this work to combine the techniques of superiority-based fuzzy-stochastic programming, interval linear programming, and diversified integer linear programming in practice. Researcher Mikhailov and colleagues [4] investigated Multiple Criteria Decision Making (MCDM) techniques, in which the goal is for decision-makers to recognise their most favoured alternative based on imprecise or erroneous criteria measurements. In this work, the authors developed a decision support framework for a vendor in the European electricity market to demonstrate the techniques. An MCDM practice was employed in conjunction with the perspectives of various stakeholders for a district heating system in Vancouver, Canada, to calculate and rank the available sources of energy. The study’s main objective was to show that the way information is communicated has an impact on how people rank various options. Sewer heat, natural gas, biomass (wood pellets), and geothermal heat were some of the sources of energy that were available to authors.
In addition, they employed the Preference Ranking Organization Method for Enrichment Evaluation (PROMETHEE) technique to rating the energy alternatives available. In addition, the authors of this article offer a decision-making model for multi-criteria problems based on fuzzy sets for the selection of wind turbine suppliers in energy projects of wind, which they believe will be beneficial. The proposed study is employing two decision-making procedures for multi-criteria problems: the Fuzzy Analytic Hierarchy Process (FAHP) and the Fuzzy Technique for Order of Preference by Similarity to Ideal Solution (FTOPSIS). After figuring out the calculation criteria and options, the final calculation and selection of a wind turbine supplier are done using the FAHP and the FTOPSIS decision-making practices.
Recent studies have concentrated on the practise of making energy investment decisions. According to Olak et al. [6], they investigated the feasibility of establishing large-scale hydroelectric power plants with an electric output of more than 100 kW in the Ping River Basin, Thailand using an MCDM practice. They calculated the projects’ benefits and downsides using AHP, which was founded on five key criteria: engineering and economics, socioeconomics, power generation, the environment, and stakeholder involvement, among other things. In this row, an analytic network technique was employed by Upadhyay et al. [7] to determine which energy resources were most suitable in the manufacturing business. Barros et al. [8] employed a sustainability calculation technique to calculate the general quality of the unified energy systems that they selected. It was decided to employ the following pointers: an economic, an environmental, and a social indicator.
Karatop and colleagues [9] developed a decision-support model that contains a professional sub-system as well as a decision-making technique for multi-criteria issues. It accepted the “new parameters” of the energy market, notably liberalisation and climate change, the system assisted the government in establishing an environment that was more conducive to modern living. The strategy has been successfully adopted in all of the European Union’s thirteen new member states with great success. Beccali et al. [10] did a multi-criteria sustainability evaluation of many energy and power system options in order to look at new capacity-building options for this very complicated system.
As for alternative options, such as a thermal power unit with a coal-fueled boiler, hydropower plants, fluidized bed combustion, mixed cycle gas turbine plants, biomass power plants, wind turbines, and photovoltaic power stations, they calculated the reintegration of a 110 MW thermal power unit. For their research, Sarpong and colleagues [11] conducted a local case study in the United Kingdom, examining the varying degrees of renewable energy provision for local government. It was determined that these small-scale energy solutions had higher perceived social, economic, and environmental costs than alternatives that were implemented on a larger scale. Using a decision-making practise for multi-criteria problems, the authors calculated the advantages and disadvantages of several renewable energy technologies in order to establish whether large-scale projects could have provided energy at a lower environmental, economic, and social cost.
In accordance with the United Kingdom government’s definition of renewable energy, they looked into eight different technologies of renewable energy at various scales: large-scale wind and hydro, micro-hydro, micro-wind, solar photovoltaic, landfill gas, energy from waste, and biomass. A decision-making technique of multi-criteria devised by Goletsis et al. [12] was employed to calculate the environmental impact of producers of renewable energy in fourteen different European Union member states. The strategy was based on an ordered weighted average of qualitative calculations. In a study on the potential practise of natural gas in the energy industry, Butkiene and colleagues [13] conducted an investigation. The criteria were grouped into four categories: environmental, social, economic, and technological. Economic criteria were given the highest priority. The following systems were investigated as potential gas-using alternatives: Gas turbine power plants include things like unified cycle power plants, unified heat and power plants, steam turbine gas-fired power plants, and fuel cell power plants, to name a few types of them.
To examine the various choices, they employed a decision-making practise of multi-criteria, the general index of sustainability, which prioritised economic, environmental, social, and technological considerations. According to Medjoudj et al. [14], a practical model was developed in order to shed light on the application of multi-criteria approaches in renewable energy planning. They generated a matrix that calculates the numerous decision-making strategies of multi-criteria that are appropriate for renewable energy planning, as well as their efficacy in doing so. Topcu et al. [15] conducted a survey on decision analysis in energy and environmental modelling to learn more about the topic. They noticed that there were roughly 252 publications in the database. They also improved and enhanced the survey by categorising the 252 publications according to their publication source, decision analysis practice, application area, and a few more additional criteria, among others. An investigation into the applicability of alternative decision analysis practises in each application area was conducted through the use of statistical studies, including multiple attribute analysis and hypothesis testing.
Researchers determined that the importance of multi-criteria decision-making practices, as well as energy-related environmental research, has greatly increased in recent years. Büyüközkan et al. [16] approached the energy policy problem as a decision-making technique of multi-criteria, with interacting criteria and options, rather than as a single problem. Her analysis of alternative sources of energy for Turkey’s energy resources was conducted with the help of the ANP. To make a preliminary calculation of the feasibility of erecting a few energy wind turbines on the Italian island of Sanlier et al. [17] suggested a decision-making procedure for multi-criteria problems to aid in the selection and calculation of one or more alternatives. The practise was developed to aid in the selection and calculation of one or more alternatives. The four different wind turbine configurations were calculated and compared. They employed a multi-criteria approach to calculate the many alternatives they presented. Fuzzy principles, outranking, priority setting, weighted averages, and their combinations were researched and employed by Sadeghi et al. [18] in their energy planning decisions, among other things. They did a math problem based on more than 90 published articles to see if the approaches worked.
The hierarchy practise was shown to be the most popular approach, which was monitored by outranking practises and the elimination and choice practise of interpreting reality as the second and third most popular strategies, respectively. It was the work of Nigim and colleagues [19] that employed the calculation of decision-making tactics for multi-criteria energy resource problems that enabled Turkey to select the most cost-effective power-generating alternative available. They also provided a rating of the various options as well as a unified decision-assistance framework for selecting the best-suited multi-attribute technique for the situation. Stakeholder talks with renewable energy projects and wind stakeholders, as well as the issues that have occurred, were documented by Kumar et al. [20], who also developed a new practical model for multi-participant and multi-criteria problem solving.
For the purpose of developing a new regulatory framework, they looked at a variety of case studies, with a particular emphasis on the Greek renewable energy sector. Pang and colleagues [21] investigated how the decision-making procedure for multi-criteria problems may be applied to the calculation of a regional action plan for the diffusion of renewable energy technology in the Philippines. They also conducted a case study on the island of Sardinia, which is located in the Mediterranean Sea. They employed the Elimination and Choice Expressing Reality (ELECTRE)-III technique in a fuzzy environment, which was successful. Using the analysis of the parametric diagram breakdown into indifference zones corresponding to basic efficient solutions, Ramezanzade and colleagues [22] proposed an interactive practise for dealing with fuzzy multi-objective linear programming problems based on the analysis of the parametric diagram breakdown into indifference zones corresponding to basic efficient solutions. On a national scale, the practise was demonstrated to be effective in addressing uncertainty and imprecision in the coefficients of an input–output energy–economy planning model. The practise is meant to help people who make decisions about how the energy system and the economy work together on a national level.
Through the use of group tactics in conjunction with a decision-making procedure for multi-criteria problems, Shao and colleagues [23] established a unified system for prioritising project proposals in Armenia’s energy business. They established a multi-criteria ranking strategy that was a unified of the ELECTRE III and PROMETHEE practices, which served as the foundation for a unified group project ranking practise that was further refined. When Saraswat and her colleagues [24] used the PROMETHEE II outranking method to get everyone in a group to agree on wind and renewable energy projects, they came up with an adaptable group decision-making framework that has been used by other people.
The suggested framework was put to the test in a case study involving the extraction of geothermal resources on the Greek island of Chios, which was conducted as part of the project. Montoya and colleagues [25] developed criteria and options for evaluating innovative and renewable energy technologies that were based on the analysis and synthesis of parameters using the information deficiency approach to construct energy indicators for evaluating energy systems that met the sustainability criterion using the information deficiency approach to construct energy indicators for evaluating energy systems that met the sustainability criterion. The considerations taken into consideration included energy resources, environmental capacity, social factors, and economic indicators. Wu and colleagues [26] extended PROMETHEE, a multi-criteria technique for ranking alternative projects, by using fuzzy input data. The proposed practise was applied in this study in order to rank and calculate low-temperature geothermal energy alternative energy exploitation strategies.
This section outlines a practise for selecting wind turbine suppliers in energy projects powered by wind. The approach is divided into three steps [27–30]. The first phase requires the company to create a comprehensive hierarchy of all the factors that may have an impact on the company. This is accomplished by extensively examining the chain in question and finding any potential flaws. The overlaps are then calculated, and similar traits are employed to group the results. This exercise should be done whenever the chain undergoes a significant modification. The practise then moves on to giving weights to the criteria based on their relevance. FAHP is employed for this, and expert opinions are incorporated. The third phase involves examining the scores of several criteria under some main categories. The FTOPSIS technique is employed in the fourth step to calculate the organization’s readiness to utilise green raw materials. Finally, the consequences for management have been examined.
3.1 Fuzzy Analytic Hierarchy Process
By integrating Saaty’s AHP with fuzzy set theory, the FAHP practise expands Saaty’s AHP [27]. The relative intensity of the factors in the related criteria is indicated using fuzzy ratio scales in FAHP. As a result, a fuzzy judgement matrix can be built. Alternatives’ ultimate scores are likewise signified by fuzzy numbers [31–33]. The best option is found by applying specific algebraic operators to the fuzzy integers. These fuzzy integers are used to represent all of the members of the judgement matrix and weight vectors in this method.
A fuzzy judgement vector is then created for each criterion using fuzzy numbers to reflect the relative importance of one kind over the other [34,35]. These judgement vectors are then used to compute the weight of each criterion using the fuzzy pairwise comparison matrix. Tab. 1 depicts the meaning of linguistic expressions as fuzzy integers, and Tab. 2 explains how to determine the Consistency Ratio (CR) using the random consistency index. In Fig. 1, the fuzzy membership function for linguistic phrases is presented for criteria and sub-criteria. Specialists are demanded to provide their opinions on the arrangement of language terms, which are subsequently transformed and examined to arrive at the final weights.
Figure 1: Fuzzy membership function for linguistic expressions for criteria and sub-criteria
3.2 Fuzzy Technique for Order of Preference by Similarity to Ideal Solution
To represent linguistic value, fuzzy set theory can be employed [28]. As a result, the FTOPSIS approach is well suited to handle real-world application issues in a fuzzy setting. TOPSIS was designed as one of the classic multi-criteria decision-making systems [5–8]. It is founded on the principle that the chosen option should be the closest to the ideal solution while being the furthest away from the ideal negative solution. TOPSIS also has a customizable and easy-to-understand computation technique. It has the ability to consider multiple criteria with various units at the same time [29–31]. Following are the steps involved in FAHP-TOPSIS estimation.
Step 1: Make a decision on the linguistic rating values for the alternative in relation to the criteria.
It is being assumed that there are m possible alternatives called A = {A1, A2…Am} which are to be calculated against the criteria, C = {C1, C2……Cn}. Further, the criteria weights are signified by wj = {j = 1, 2, …, n}. Also, the performance ratings of each expert Dk{k = 1, 2, …K} for each alternative Ai{i = 1, 2….m} with respect to criteria Cj{j = 1, 2, …n} are signified by a membership function. The linguistic variable Tab. 3 contains the scale that was employed to rate the solutions.
Step 2: Next step is calculating aggregate fuzzy ratings for the alternatives
Let us assume, if the fuzzy ratings of all experts are termed as triangular fuzzy numbers
And, if the fuzzy rating of the kth experts are
Step 3: Next step is constructing the fuzzy decision matrix
The fuzzy decision matrix for the alternatives
Step 4: Afterward create normalize fuzzy decision matrix.
The raw data are normalized by means of linear scale transformation to carry the numerous criteria scales into a comparable scale. The normalized fuzzy decision matrix
where
Step 5: Construct the weighted normalized matrix
The weighted normalized matrix (wj) for criteria is calculated by multiplying the weights (wj) of calculation criteria with the normalized fuzzy decision matrix
Note that
Step 6: Determine the fuzzy positive ideal solution (FPIS) and fuzzy negative ideal solution (FNIS)
The FPIS and FNIS of the alternatives is computed as follows:
i = 1, 2, 3…m; j = 1, 2, …n
Step 7: Next step is to compute the distance of each alternative.
The distance (
Step 8: Compute the closenessoefficient ClCi of each alternative
The closeness coefficient ClCi embodies the distances to the fuzzy positive ideal solution (dt−) and the fuzzy negative ideal solution (dt−) simultaneously. The closeness coefficient of each alternative is considered as:
Step 9: Next step ranks the alternatives
In step 9, the various alternatives are ranked or selected according to the maximum closeness coefficient ClCi values in decreasing order.
There are favorable elements in the market of the Sultanate of Oman that allow wind power investors to find excellent investment prospects in the country. The government, in addition to demonstrating concrete maintenance for energy wind improvement, provides subsidies to energy wind projects to decrease monetary risk, thus enabling stakeholders to test and establish new operations in addition to their natural resources. A large number of energy projects based on wind are already under construction in this region. According to this, the authors have proposed a decision-making practice for multi-criteria problems based on fuzzy sets that can be employed in the selection of wind turbine suppliers in wind energy projects. All of the criteria are used when evaluating wind turbine suppliers and they were chosen by 27 experts (renewable energy scientists, mechanical engineers, and more) and a literature review, as shown in Tab. 4 and Fig. 2.
Figure 2: The tree structure between factors and turbine alternatives
Machine Feature: When compared to a real windmill, the model wind turbine employed in this activity is more accurate. This model is a straightforward machine, consisting primarily of a wheel and an axle. A gust of wind blows on the blades (the paper wheel), which causes the axle to rotate (the straw). As the straw rotates, the piece of thread begins to wind itself around the straw, and the paperclip rises with the straw [30,31].
Environmental: Wind energy, like all sources of energy, has the potential to have negative environmental implications, including the reduction, degradation, or fragmentation of habitats for plants, fish, and wildlife. Wind energy, like all other sources of energy, has the potential to have negative environmental consequences. Also, revolving turbine blades can be a danger to flying species such as bats and birds, which can become entangled in them. In light of the potential negative implications that energy from wind can have on wildlife and the possibility for these problems to delay wind improvement in high-quality wind resource areas, permitting issues, siting, and addressing impact minimization are among the highest priorities for the wind industry. In addition, the wind industry has a lot of issues to deal with, such as how wind turbines should be placed and how they should be approved [32,33].
Technological: A wind turbine is an equipment that is employed to transform the kinetic energy of the wind into electrical energy by spinning the blades. Various sizes of wind turbines are available, with small-scale versions employed for supplying electricity to rural cottages or cabins and community-scale models employed to supply electricity to a small number of residences within a community. On an industrial scale, a significant number of huge turbines are grouped together to form wind farms, which are typically placed in rural areas or offshore. The phrase “windmill,” which normally refers to the conversion of energy from the wind into mechanical energy for milling or pumping, is sometimes employed to refer to a wind turbine, despite the fact that the two are not synonymous. On the other hand, “wind turbine” is used a lot by people who talk about renewable energy in a mainstream way (see also “wind power”) [34,35].
Monetary: The monetary sub-problem is primarily constituted of two criteria that reflect primarily the impacts, which together account for nearly all of the investment and operational expenditures that are necessary. In order to determine investment expenses, benchmark data for electrical apparatus (transformers, substations, reactive power control measures, and shunts) as well as decommissioning, commissioning, interconnection, and costs are employed. The benchmark data is employed to compute the investment expenses. Aside from the direct monetary impact of the wind farm equipment (which we consider to be the same for all candidate sites—identical installations), the continuing investments are mostly influenced by the geographic location of the wind farm site. If you want to know how much money it will cost to get wind power to your home or business, you need to think about things like the distance between your wind farm and the onshore substation (like the length of the cable and the amount of reactive power) and the water depth in the area where you want to build your wind farm.
The data gathered from the designed questionnaire were linguistic terms supplied by domain experts, as indicated in Tab. 5. Because the experts came from a variety of backgrounds and areas of expertise in energy systems, they were asked to reach an agreement on possible energy systems, criteria, and fuzzy terminology. Tab. 5 also shows the average of the triangular fuzzy numbers calculated for each energy system in comparison to the criteria. As a result, the disparate viewpoints based on fuzzily defined figures were gathered and consolidated. In addition, utilising [2,5,8,15,19,23], the experts’ viewpoints were compiled and given in Tab. 5. To regulate the importance of each factor, the decision makers used fuzzy linguistic concepts and analysed each energy system in relation to the chosen criteria. Based on the fuzzy linguistic phrases and numbers supplied in Tabs. 1, 2, and Fig. 1, the outcomes of FAHP practise were determined in the preceding section. Tabs. 6–8, and 9 for level 2 are similarly provided as an average of triangular fuzzy numbers and corresponding weights for each set of level 2’s attributes. Tab. 10 also shows the global weights and rankings of each criterion in order of hierarchy.
In recent years, Oman’s overall final consumption has consistently climbed. In 2000, energy usage was 588 ktoe (6,833 GWh), but by 2011 it had risen to 1,591 ktoe (18,512 GWh) [35]. As multiple sectors interact, the residential sector consumes the most energy, accounting for 49%, followed by commercial and public services (34%), and industry (14%). The number of electrical subscribers increased by 8.6% from 727,483 in 2011 to 790,277 in 2012. Residential customers account for the vast bulk of electricity users, accounting for 71% of the total. Wind energy has various benefits, which is why it is one of the fastest-growing energy sources on the planet. The study’s purpose is to figure out how to get through the barriers that prohibit more people from using wind power. Wind-generated electricity has been increasing at a rapid rate in recent decades. Between 2001 and 2016, the combined wind power installation capacity climbed from 23.9 to 486.79 GW.
Furthermore, recent technological advancements in wind turbine systems have significantly reduced the cost of wind energy. This assists in overcoming obstacles such as the high initial cost of installing and operating small wind turbines. Small wind turbines have a rotor swept area of less than 200 m2 and a rated power of less than 50 kW. Small wind turbines are also characterised as turbines with a power output of less than 100 kW. This suggests that modest wind turbines are capable of supplying electricity to a wide range of locations, including residential and commercial complexes, schools, farms, and ranches, to mention a few. Tab. 11 demonstrates how tiny wind turbines are classified according to their swept area, rated power, and common applications [35]. This suggests that the customer has a selection of small wind turbines from which to choose to meet their individual demands and requirements. This research chose seven different wind turbines that might be used for the applications listed in Tab. 11. Throughout this investigation, the wind turbines are referred to as WT1, WT2, WT3, WT4, WT5, WT6, and WT7. The operating parameters and specifications of the selected wind turbines are shown in Tab. 12 [35].
The authors used this information to input technological data for seven wind energy projects, as shown in Tab. 13, utilising Tab. 2 from the practise section. The FAHP global weights of criteria are used as inputs in the FTOPSIS practise to generate a rank for each alternative. Eqs. (1)–(6) are used in this case, and a normalised decision-matrix is generated. The normalised values of the decision-matrix are then multiplied by the weights of each criterion using Eqs. (7)–(9), providing a fuzzy weighted normalised decision-matrix. Eq. (10) is then used to derive the fuzzy negative-ideal solution and the fuzzy positive-ideal solution. Eqs. (11)–(12) are then used to calculate the distance between the option value and the NIS and PIS. As a last step, the performance value of each criterion was calculated using Eq. (13), and the ranking of alternatives was based on the performance score, which is shown in Tab. 14 and in Fig. 3.
Figure 3: Overall calculation of alternatives
This study developed a unique decision-making strategy based on MCDM which uses fuzzy sets for wind turbine supplier selection in wind energy projects. To rank turbine vendors for energy projects involving wind, the proposed fuzzy decision-making practise for multi-criteria problems integrates FAHP and FTOPSIS models. The weight of the criteria is calculated using FAHP. These values are employed by FTOPSIS to rate the turbine vendors. Experts and academics have worked together to come up with a set of criteria for evaluating the quality of wind turbine providers. These criteria include machine attributes as well as financial, technological, and environmental issues.
Additionally, MCDM problems encompass domains in which we are unable to determine if the solution to a given problem is totally true or completely false. This paper also attempts to solve these problems without taking into account their imprecision will yield ineffective results. Fuzzy logic is critical in order to discover efficient and effective solutions to these difficulties. The authors compared the results from the AHP technique with those from other techniques to make the evaluation process more meaningful and to determine whether the proposed methodology is appropriate or not.
Furthermore, the authors compared findings using the AHP technique using the same resources and criteria. The findings of both techniques demonstrate that the accepted fuzzy-AHP-TOPSIS methodology produces the most effective outcomes, and the difference between the two methodologies is not too great. According to the findings of this work, supplier WT1 is the best option. Furthermore, the conclusion is as follows:
• The proposed framework is the Sultanate of Oman’s first of its kind wind turbine calculation and selection model, based on expert interviews and reviews.
• As a follow-up, this is the first study to examine how the FAHP and FTOPSIS models combine to calculate renewable sources of energy.
• The resolutions of this work can serve as a beneficial guide for both energy projects and other renewable energy initiatives, such as wind turbines and solar panels.
• For more research on this topic, the study could be extended to include other decision-making practises of multi-criteria such as data envelopment analysis, the unified comprehensive solution approach, and so on.
Moreover, decision-making procedures are still in their infancy due to the intricacy of renewable energy choices; hence, more empirical evidence is required to be very conclusive and emphatic regarding the proposed mechanism. There are many additional MCDM ways available in technology, and many integration methods have yet to evaluate their effects, so this is also a weakness of our study.
Funding Statement: The authors received no specific funding for this study.
Conflicts of Interest: The author declares that they have no conflicts of interest to report regarding the present study.
References
1. C. Kahraman, I. Kaya and S. Cebi, “Renewable energy system selection based on computing with words,” International Journal of Computational Intelligence Systems, vol. 3, no. 4, pp. 461–473, 2010. [Google Scholar]
2. H. C. Lee and C. T. Chang, “Comparative analysis of MCDM methods for ranking renewable energy sources in Taiwan,” Renewable and Sustainable Energy Reviews, vol. 92, no. 5, pp. 883–896, 2018. [Google Scholar]
3. C. Kahraman, I. Kaya and S. Cebi, “A comparative analysis for multiattribute selection among renewable energy alternatives using fuzzy axiomatic design and fuzzy analytic hierarchy process,” Energy, vol. 34, no. 10, pp. 1603–1616, 2009. [Google Scholar]
4. L. Mikhailov and M. G. Singh, “Fuzzy analytic network process and its application to the development of decision support systems,” IEEE Transactions on Systems, Man, and Cybernetics,” Part C (Applications and Reviews), vol. 33, no. 1, pp. 33–41, 2003. [Google Scholar]
5. J. K. Chen and I. S. Chen, “Using a novel conjunctive MCDM approach based on DEMATEL, fuzzy ANP, and TOPSIS as an innovation support system for Taiwanese higher education,” Professional Systems with Applications, vol. 37, no. 3, pp. 1981–1990, 2010. [Google Scholar]
6. M. Çolak and I. Kaya, “Prioritization of renewable energy alternatives by using an integrated fuzzy MCDM model: A real case application for urkey,” Renewable and Sustainable Energy Reviews, vol. 80, no. 2, pp. 840–853, 2017. [Google Scholar]
7. S. Upadhyay and M. P. Sharma, “A review on configurations, control and sizing methodologies of hybrid energy systems,” Renewable and Sustainable Energy Reviews, vol. 38, no. 7, pp. 47–63, 2014. [Google Scholar]
8. J. J. C. Barros, M. L. Coira, M. P. D. López and A. D. Gochi, “Assessing the global sustainability of different electricity generation systems,” Energy, vol. 89, no. 5, pp. 473–489, 2015. [Google Scholar]
9. B. Karatop, B. Taşkan, E. Adar and C. Kubat, “Decision analysis related to the renewable energy investments in Turkey based on a fuzzy-AHP-EDAS-fuzzy-FMEA approach,” Computers & Industrial Engineering, vol. 151, no. 5, pp. 106958–106969, 2021. [Google Scholar]
10. M. Beccali, M. Cellura and D. Ardente, “Decision making in energy planning: The ELECTRE multi characteristic analysis approach compared to a fuzzy-sets methodology,” Energy Conversion and Management, vol. 39, no. 16, pp. 1869–1881, 1998. [Google Scholar]
11. S. K. Sarpong, J. Sarkis and X. Wang, “Assessing green supply chain practices in the Ghanaian mining industry: A framework and estimation,” International Journal of Production Economics, vol. 181, no. 1, pp. 325–341, 2016. [Google Scholar]
12. Y. Goletsis, C. Papaloukas, D. I. Fotiadis, A. Likas and L. K. Michalis, “Automated ischemic beat classification using genetic algorithms and multicharacteristic decision analysis,” IEEE Transactions on Biomedical Engineering, vol. 1, no. 10, pp. 1717–1725, 2004. [Google Scholar]
13. I. S. Butkiene, E. K. Zavadskas and D. Streimikiene, “Multi-characteristic decision-making for the assessment of renewable energy technologies in a household: A review,” Energies, vol. 13, no. 5, pp. 1164–1178, 2020. [Google Scholar]
14. R. Medjoudj, F. Iberraken and D. Aissani, “Combining AHP method with BOCR merits to analyze the outcomes of business electricity sustainability,” Applications and Theory of Analytic Hierarchy Process-Decision Making for Strategic Decisions, vol. 45, no. 6, pp. 277–295, 2016. [Google Scholar]
15. Y. I. Topcu and F. Ulengin, “Energy for the future: An integrated decision aid for the case of Turkey,” Energy, vol. 29, no. 1, pp. 137–154, 2004. [Google Scholar]
16. G. Büyüközkan and G. Cifçi, “A novel hybrid MCDM approach based on fuzzy DEMATEL, fuzzy ANP and fuzzy TOPSIS to calculate green suppliers,” Professional Systems with Applications, vol. 39, no. 3, pp. 3000–3011, 2012. [Google Scholar]
17. N. Sanlier, A. Dadeviren, S. Bilici and A. Abubakirova, “Determining the knowledge of food safety and purchasing behavior of the consumers living in Turkey and Kazakhstan,” African Journal of Microbiology Research, vol. 5, no. 18, pp. 2724–2732, 2011. [Google Scholar]
18. A. Sadeghi, T. Larimian and A. Molabashi, “Estimation of renewable energy sources for generating electricity in province of yazd: A fuzzy MCDM approach,” Procedia-Social and Behavioral Sciences, vol. 62, no. 10, pp. 1095–1099, 2012. [Google Scholar]
19. K. Nigim, N. Munier and J. Green, “Pre-feasibility MCDM tools to aid communities in prioritizing local viable renewable energy sources,” Renewable Energy, vol. 29, no. 11, pp. 1775–1791, 2004. [Google Scholar]
20. A. Kumar, B. Sah, A. R. Singh, Y. Deng, X. He et al., “A review of multi characteristic decision making (MCDM) towards sustainable renewable energy development,” Renewable and Sustainable Energy Reviews, vol. 69, no. 4, pp. 596–609, 2017. [Google Scholar]
21. N. Pang, Q. Meng and M. Nan, “Multi-characteristic estimation and selection of renewable energy battery energy storage system-a case study of Tibet, China,” IEEE Access, vol. 9, no. 8, pp. 119857–119870, 2021. [Google Scholar]
22. M. Ramezanzade, H. Karimi, K. Almutairi, H. A. Xuan, J. Saebi et al., “Implementing MCDM techniques for ranking renewable energy projects under fuzzy environment: A case study,” Sustainability, vol. 13, no. 22, pp. 12858–12869, 2021. [Google Scholar]
23. M. Shao, Z. Han, J. Sun, C. Xiao, S. Zhang et al., “A review of multi-characteristic decision making applications for renewable energy site selection,” Renewable Energy, vol. 157, no. 4, pp. 377–403, 2020. [Google Scholar]
24. S. K. Saraswat, A. K. Digalwar, S. S. Yadav and G. Kumar, “MCDM and GIS based modelling technique for assessment of solar and wind farm locations in India,” Renewable Energy, vol. 169, no. 6, pp. 865–884, 2021. [Google Scholar]
25. F. G. Montoya, M. J. Aguilera and F. M. Agugliaro, “Renewable energy production in Spain: A review,” Renewable and Sustainable Energy Reviews, vol. 33, no. 7, pp. 509–531, 2014. [Google Scholar]
26. Y. Wu, T. Zhang, R. Gao and C. Wu, “Portfolio planning of renewable energy with energy storage technologies for different applications from electricity grid,” Applied Energy, vol. 287, no. 1, pp. 116562–116574, 2021. [Google Scholar]
27. T. L. Saaty, “Decision making-the analytic hierarchy and network processes (AHP/ANP),” Journal of Systems Science and Systems Engineering, vol. 13, no. 1, pp. 1–35, 2004. [Google Scholar]
28. L. A. Zadeh, “Fuzzy sets,” Fuzzy Sets, Fuzzy Logic, and Fuzzy Systems, vol. 1, no. 1, pp. 394–432., 1996. [Google Scholar]
29. M. Kabak and M. Dağdeviren, “Prioritization of renewable energy sources for Turkey by using a hybrid MCDM methodology,” Energy Conversion and Management, vol. 79, no. 5, pp. 25–33, 2014. [Google Scholar]
30. Y. Wu, J. Wang, S. Ji and Z. Song, “Renewable energy investment risk assessment for nations along China’s belt & road initiative: An ANP-cloud model method,” Energy, vol. 190, no. 6, pp. 116381–116398, 2020. [Google Scholar]
31. D. Timmons, J. M. Harris and B. Roach, “The economics of renewable energy,” Global Development and Environment Institute Journal, vol. 52, no. 12, pp. 1–52, 2014. [Google Scholar]
32. M. M. D. Widianta, T. Rizaldi, D. P. S. Setyohadi and R. Riskiawan, “Comparison of multi-characteristic decision support methods (AHP, TOPSIS, SAW & PROMENTHEE) for employee placement,” Journal of Physics: Conference Series, vol. 953, no. 1, pp. 012116–012126, 2018. [Google Scholar]
33. F. Torfi and A. Rashidi, “Selection of project managers in construction firms using analytic hierarchy process (AHP) and fuzzy TOPSIS: A case study,” Journal of Construction in Developing Countries, vol. 16, no. 1, pp. 69–89, 2011. [Google Scholar]
34. M. Pourmehdi, M. M. Paydar and E. A. Gangraj, “Reaching sustainability through collection center selection considering risk: Using the integration of fuzzy ANP-TOPSIS and FMEA,” Soft Computing, vol. 25, no. 16, pp. 10885–10899, 2021. [Google Scholar]
35. R. Ahshan, A. Albadi, N. Hosseinzadeh and M. Shafiq, “Small wind turbine systems for application in Oman,” in Proc. of the 2018 5th Int. Conf. on Electric Power and Energy Conversion Systems, Kitakyushu, Japan, pp. 1–6, 2018. https://doi.org/10.1109/EPECS.2018.8443520. [Google Scholar]
Cite This Article
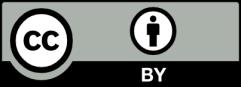