Open Access
ARTICLE
Nonlinear Dynamic System Identification of ARX Model for Speech Signal Identification
1 Department of ECE, ITER, Siksha ‘O’ Anusandhan (Deemed to be University), Bhubaneswar, India
2 Department of CSE, Chitkara University Institute of Engineering & Technology, Punjab, India
3 Department of CSE, ITER, Siksha ‘O’ Anusandhan (Deemed to be University), Bhubaneswar, India
* Corresponding Author: Mihir N. Mohanty. Email:
Computer Systems Science and Engineering 2023, 46(1), 195-208. https://doi.org/10.32604/csse.2023.029591
Received 07 March 2022; Accepted 25 May 2022; Issue published 20 January 2023
Abstract
System Identification becomes very crucial in the field of nonlinear and dynamic systems or practical systems. As most practical systems don’t have prior information about the system behaviour thus, mathematical modelling is required. The authors have proposed a stacked Bidirectional Long-Short Term Memory (Bi-LSTM) model to handle the problem of nonlinear dynamic system identification in this paper. The proposed model has the ability of faster learning and accurate modelling as it can be trained in both forward and backward directions. The main advantage of Bi-LSTM over other algorithms is that it processes inputs in two ways: one from the past to the future, and the other from the future to the past. In this proposed model a backward-running Long-Short Term Memory (LSTM) can store information from the future along with application of two hidden states together allows for storing information from the past and future at any moment in time. The proposed model is tested with a recorded speech signal to prove its superiority with the performance being evaluated through Mean Square Error (MSE) and Root Means Square Error (RMSE). The RMSE and MSE performances obtained by the proposed model are found to be 0.0218 and 0.0162 respectively for 500 Epochs. The comparison of results and further analysis illustrates that the proposed model achieves better performance over other models and can obtain higher prediction accuracy along with faster convergence speed.Keywords
Cite This Article
R. K. Pattanaik, M. N. Mohanty, S. K. Mohapatra and B. K. Pattanayak, "Nonlinear dynamic system identification of arx model for speech signal identification," Computer Systems Science and Engineering, vol. 46, no.1, pp. 195–208, 2023. https://doi.org/10.32604/csse.2023.029591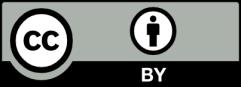