Open Access
ARTICLE
An Efficient Intrusion Detection Framework for Industrial Internet of Things Security
1 Department of Information Technology, College of Computer and Information Sciences, Princess Nourah bint Abdulrahman University, P.O.Box 84428, Riyadh, 11671, Saudi Arabia
2 Department of Computer Science and Engineering, Faculty of Electronic Engineering, Menoufia University, Menouf, 32952, Egypt
3 Security Engineering Lab, Computer Science Department, Prince Sultan University, Riyadh, 11586, Saudi Arabia
4 Department of Electronics and Electrical Communications Engineering, Faculty of Electronic Engineering, Menoufia University, Menouf, 32952, Egypt
* Corresponding Author: Walid El-Shafai. Email:
Computer Systems Science and Engineering 2023, 46(1), 819-834. https://doi.org/10.32604/csse.2023.034095
Received 07 July 2022; Accepted 07 November 2022; Issue published 20 January 2023
Abstract
Recently, the Internet of Things (IoT) has been used in various applications such as manufacturing, transportation, agriculture, and healthcare that can enhance efficiency and productivity via an intelligent management console remotely. With the increased use of Industrial IoT (IIoT) applications, the risk of brutal cyber-attacks also increased. This leads researchers worldwide to work on developing effective Intrusion Detection Systems (IDS) for IoT infrastructure against any malicious activities. Therefore, this paper provides effective IDS to detect and classify unpredicted and unpredictable severe attacks in contradiction to the IoT infrastructure. A comprehensive evaluation examined on a new available benchmark TON_IoT dataset is introduced. The data-driven IoT/IIoT dataset incorporates a label feature indicating classes of normal and attack-targeting IoT/IIoT applications. Correspondingly, this data involves IoT/IIoT services-based telemetry data that involves operating systems logs and IoT-based traffic networks collected from a realistic medium-scale IoT network. This is to classify and recognize the intrusion activity and provide the intrusion detection objectives in IoT environments in an efficient fashion. Therefore, several machine learning algorithms such as Logistic Regression (LR), Linear Discriminant Analysis (LDA), K-Nearest Neighbors (KNN), Gaussian Naive Bayes (NB), Classification and Regression Tree (CART), Random Forest (RF), and AdaBoost (AB) are used for the detection intent on thirteen different intrusion datasets. Several performance metrics like accuracy, precision, recall, and F1-score are used to estimate the proposed framework. The experimental results show that the CART surpasses the other algorithms with the highest accuracy values like 0.97, 1.00, 0.99, 0.99, 1.00, 1.00, and 1.00 for effectively detecting the intrusion activities on the IoT/IIoT infrastructure on most of the employed datasets. In addition, the proposed work accomplishes high performance compared to other recent related works in terms of different security and detection evaluation parameters.Keywords
Cite This Article
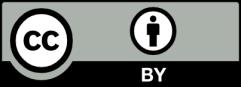