Open Access
ARTICLE
A Deep Learning Ensemble Method for Forecasting Daily Crude Oil Price Based on Snapshot Ensemble of Transformer Model
1 Department of Mathematics, Faculty of Science, Suez Canal University, Ismailia, 41522, Egypt
2 Mining and Petroleum Department, Faculty of Engineering, Al-Azhar University, Qena, Egypt
3 School of Computer Science and Technology, Central South University, Changsha, Hunan, China
4 College of Computer Engineering and Sciences, Prince Sattam Bin Abdulaziz University, Al-Kharj, 11942, Saudi Arabia
5 Higher Future Institute for Specialized Technological Studies, Cairo, 3044, Egypt
* Corresponding Author: Ahmed Ali. Email:
Computer Systems Science and Engineering 2023, 46(1), 929-950. https://doi.org/10.32604/csse.2023.035255
Received 14 August 2022; Accepted 13 November 2022; Issue published 20 January 2023
Abstract
The oil industries are an important part of a country’s economy. The crude oil’s price is influenced by a wide range of variables. Therefore, how accurately can countries predict its behavior and what predictors to employ are two main questions. In this view, we propose utilizing deep learning and ensemble learning techniques to boost crude oil’s price forecasting performance. The suggested method is based on a deep learning snapshot ensemble method of the Transformer model. To examine the superiority of the proposed model, this paper compares the proposed deep learning ensemble model against different machine learning and statistical models for daily Organization of the Petroleum Exporting Countries (OPEC) oil price forecasting. Experimental results demonstrated the outperformance of the proposed method over statistical and machine learning methods. More precisely, the proposed snapshot ensemble of Transformer method achieved relative improvement in the forecasting performance compared to autoregressive integrated moving average ARIMA (1,1,1), ARIMA (0,1,1), autoregressive moving average (ARMA) (0,1), vector autoregression (VAR), random walk (RW), support vector machine (SVM), and random forests (RF) models by 99.94%, 99.62%, 99.87%, 99.65%, 7.55%, 98.38%, and 99.35%, respectively, according to mean square error metric.Keywords
Cite This Article
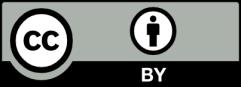